A new approach to parameter estimation of mixture of two normal distributions
COMMUNICATIONS IN STATISTICS-SIMULATION AND COMPUTATION(2024)
摘要
Mixtures of two-component normal distributions (MixN) have various applications in statistical inference with flexibility in density fitting. The best estimation of the five model parameters still represents a challenge. This article proposes more accurate density fittings given a random sample in both the moment-based and likelihood-based estimation frameworks. Motivated by the excellent performance of the Quasi-Monte Carlo method in quantile estimations, we propose an innovative approach to improve the accuracy of parameter estimations by reinforcing the representativeness of observed data via the distribution-free Harrell-Davis quantile estimators. The revision on the penalized maximum likelihood method is also considered due to the unpleasant properties of the original likelihood function under MixN. The bootstrap bias-corrected moment estimators are given as another revision. A sequential algorithm for optimization (SNTO) is conducted in finding numerical solutions for the two types of parameter estimation methods. SNTO is more adapted to MixN and shows strong advantages in the likelihood-based estimation compared to the famous EM algorithm. Simulation results show that our proposed approach can effectively improve estimation accuracy and increase resistance to small sample sizes and/or high percent overlaps between two mixture components. A real data example is given to illustrate the efficiency of our proposed methods.
更多查看译文
关键词
Maximum likelihood estimation,Mixture normal distributions,Moment estimation,Penalized maximum likelihood estimation,Quasi-Monte Carlo,SNTO algorithm
AI 理解论文
溯源树
样例
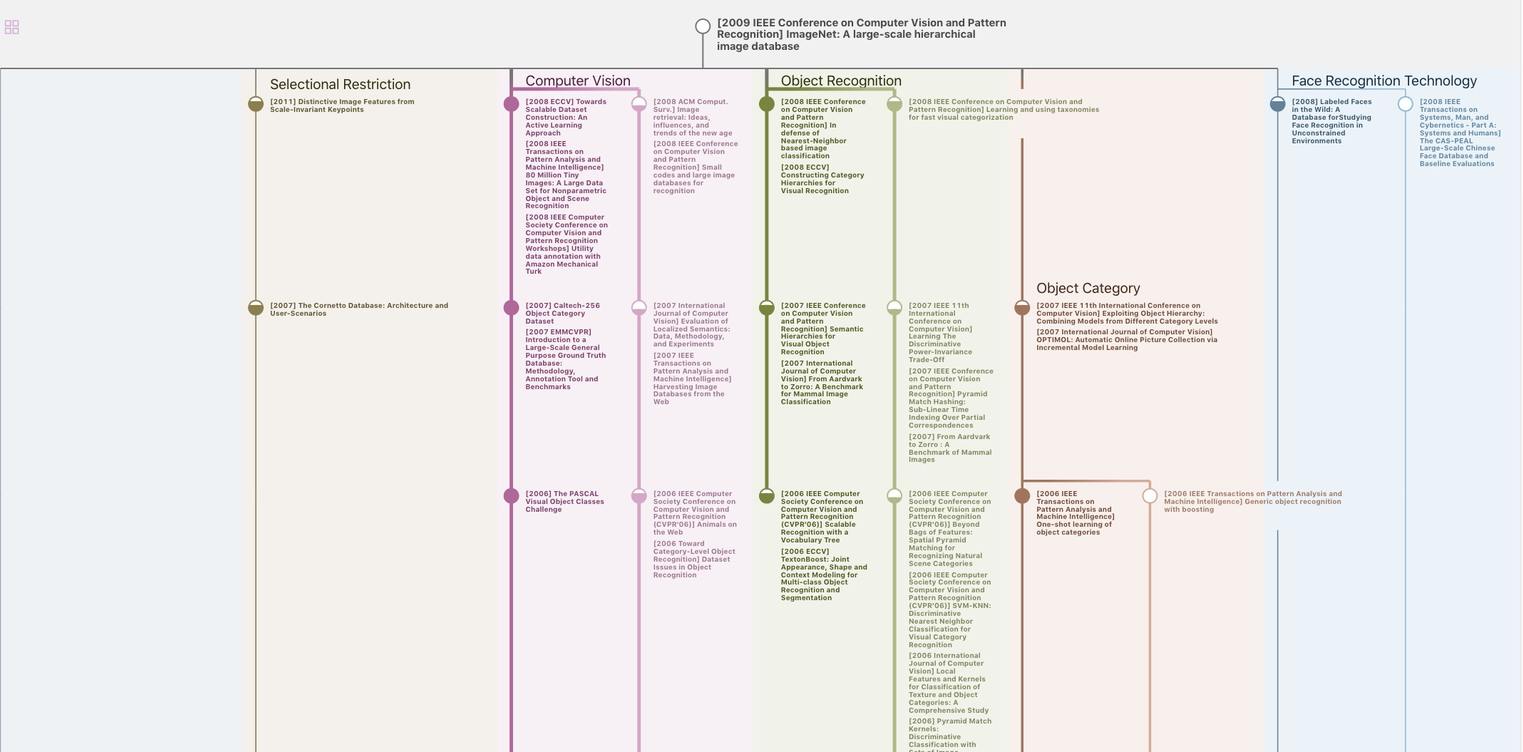
生成溯源树,研究论文发展脉络
Chat Paper
正在生成论文摘要