Site-Specific Beam Codebook Design for Distributed RIS Networks Using Deep Reinforcement Learning.
GLOBECOM (Workshops)(2023)
摘要
Reconfigurable intelligent surfaces (RISs) have recently been identified as a prominent technology capable of augmenting propagation environments by intelligently redirecting signals towards designated receivers. Instead of having a large single RIS, this paper proposes a distributed deployment of smaller RISs to reap the full benefits of spatial diversity and reduced computational complexity. Nonetheless, determining the optimal phase shift configuration for distributed RISs presents challenges, attributed to the passive nature of their reflective elements and complexities associated with obtaining accurate channel state information (CSI) in millimeter wave multi-input multi-output systems. To address this, the paper introduces a multi-agent deep reinforcement learning (MA-DRL) framework that circumvents the need for CSI, relying solely on received power measurements for feedback. The MA-DRL framework jointly designs beamforming and reflection codebooks for the base station and distributed RISs, respectively. Simulation results demonstrate the superiority of the distributed RIS approach compared to a centralized RIS configuration with an equivalent number of reflecting elements, showcasing reduced beam training overhead. Moreover, the proposed MA-DRL method outperforms widely-adopted discrete Fourier transform (DFT) codebooks, achieving an impressive 89% reduction in beam training overhead while utilizing only four beams.
更多查看译文
关键词
Deep Reinforcement Learning,Reconfigurable Intelligent Surface,Codebook Design,Phase Shift,Base Station,Discrete Fourier Transform,Joint Design,Reinforcement Learning Framework,Deep Reinforcement Learning Framework,Accurate Channel State Information,Deep Neural Network,Phase Change,Additive Noise,Singular Value Decomposition,Exhaustive Search,Beampattern,Feasible Set,Codeword,Coherence Time,User Equipment,Beamforming Gain,Beamforming Vector,Critic Network,Beam Reflectance,Learning Epochs,Received Signal Strength,Channel Coherence Time,Deterministic Policy Gradient,Number Of Beams
AI 理解论文
溯源树
样例
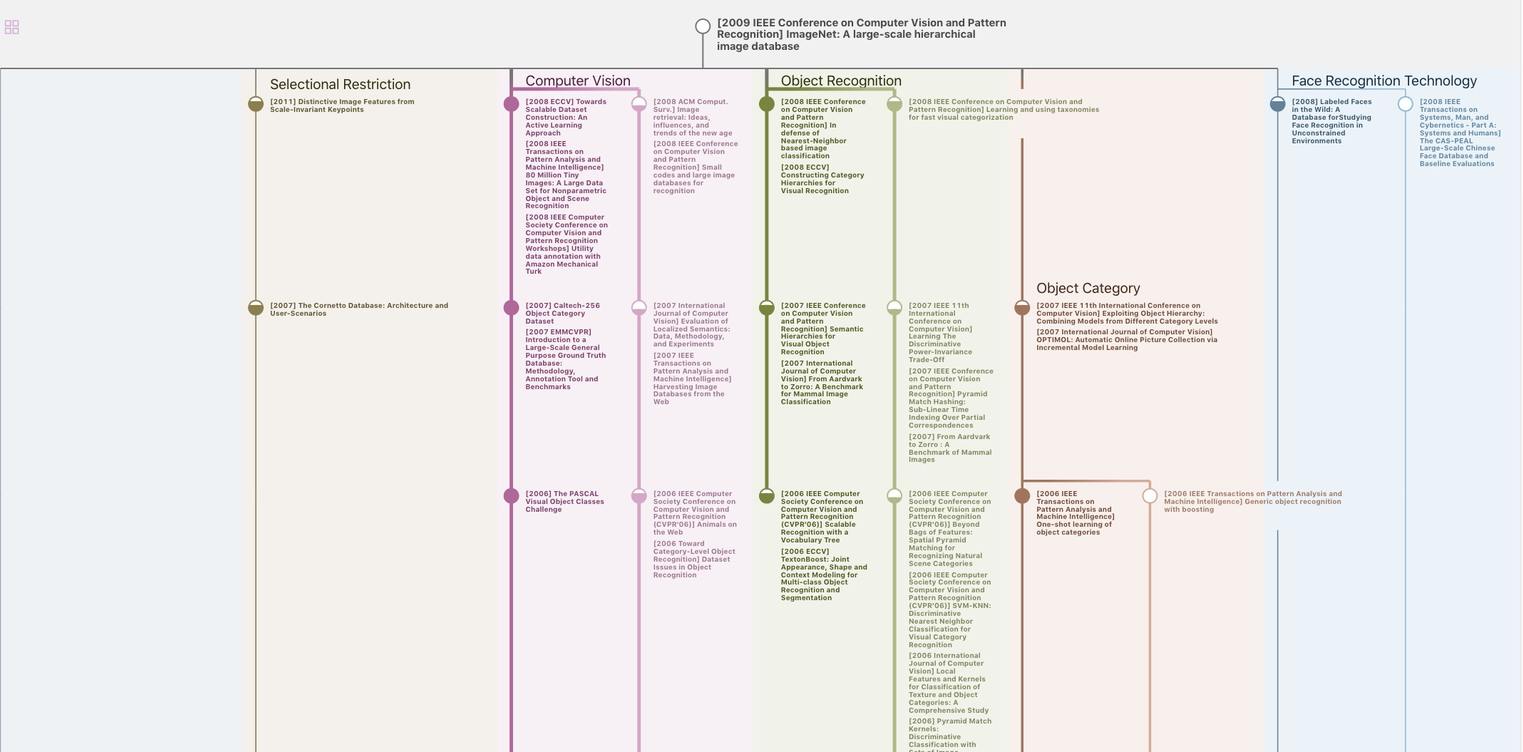
生成溯源树,研究论文发展脉络
Chat Paper
正在生成论文摘要