Image denoising via neighborhood-based multidimensional Gaussian process regression
SIGNAL IMAGE AND VIDEO PROCESSING(2022)
Abstract
As an elegant nonparametric Bayesian method, Gaussian process regression (GPR) has been successfully applied to image denoising. However, the traditional GPR faces the problem of intensive computational complexity and difficulty in capturing local-structure information. In this paper, a novel neighborhood-based image denoising method motivated by the GPR is proposed. An image is divided into several patches, and each pixel in the patches is predicted by its neighborhoods through the GPR, which allows the priors to be local and relevant and reduces the complexity of the GPR. Besides, a composite covariance function is designed to capture the local similarity between pixels. The extensive experiments demonstrate that the proposed method can not only produce a favorable denoising performance, but also have a relatively low time consumption.
MoreTranslated text
Key words
Image denoising, Gaussian process regression, Pixel neighborhoods, Composite covariance function, Multidimensional input data
AI Read Science
Must-Reading Tree
Example
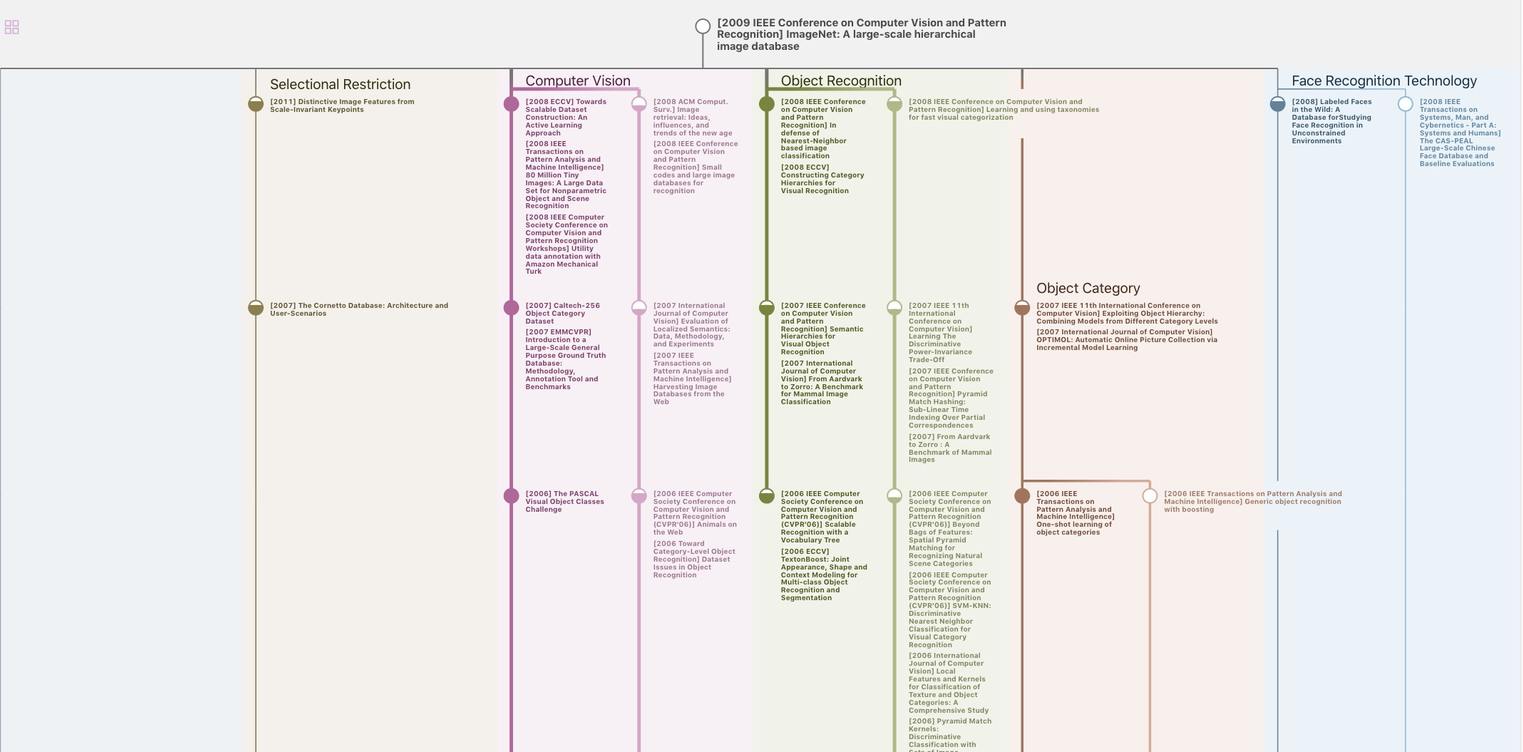
Generate MRT to find the research sequence of this paper
Chat Paper
Summary is being generated by the instructions you defined