Leveraging enterprise knowledge graph to infer web events’ influences via self-supervised learning
Journal of Web Semantics(2022)
摘要
Knowledge graph (KG) techniques have achieved successful results in many tasks, especially in semantic web and natural language processing domains. In recent years, representation learning on KG has been successfully applied to e-business applications, such as event-driven automatic investment strategies. However, there is still limited research about learning events’ influence on KG for modern quantitative investment. In this paper, we propose a novel event influence learning framework to predict stock market trends, called ST-Trend, leveraging enterprise knowledge graph to represent company correlation relationships, for mining the deep background knowledge of web events, with three self-supervised learning tasks. In particular, we devise two jointly self-supervised tasks to identify the relations between web events and companies. The first task is for generating ground-truth event-company correlation labels based on the enterprise knowledge graph. The second task is used to train how to identify the correlated companies of an event based on the generated correlation labels, with the encoding of web events, company features, and technical sequential data. We then design the prediction network to infer an event’s influence on stock price trends of the identified correlated companies based on the enterprise KG. Finally, we perform extensive experiments on a massive real-life dataset to validate the effectiveness of our proposed framework, and the experimental results demonstrate its superior performance in predicting stock market trends via considering events’ influences with the enterprise knowledge graph.
更多查看译文
关键词
Information retrieval,Knowledge graph,Stock prediction,Self-supervised learning
AI 理解论文
溯源树
样例
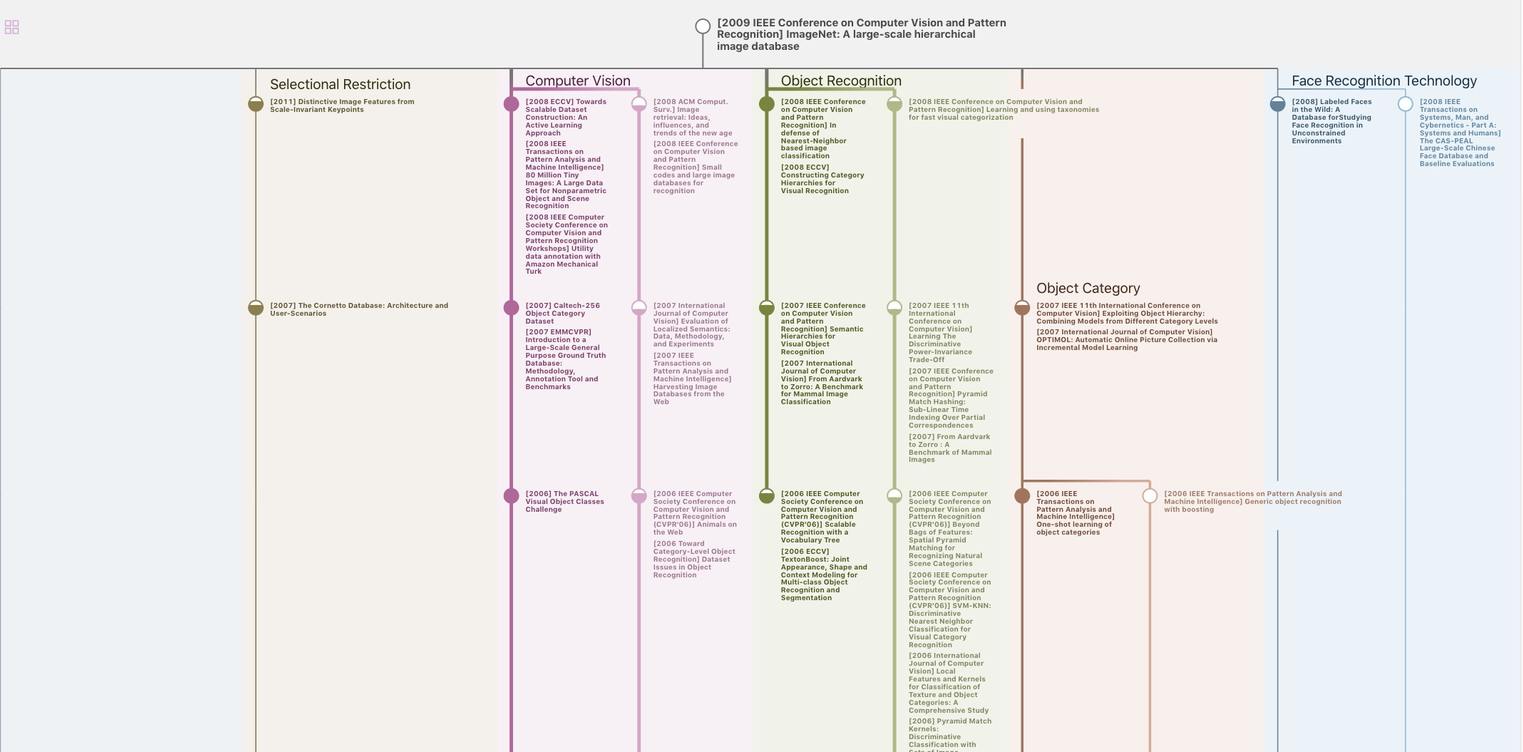
生成溯源树,研究论文发展脉络
Chat Paper
正在生成论文摘要