Compact mixed-integer programming formulations in quadratic optimization
JOURNAL OF GLOBAL OPTIMIZATION(2022)
摘要
We present a technique for producing valid dual bounds for nonconvex quadratic optimization problems. The approach leverages an elegant piecewise linear approximation for univariate quadratic functions due to Yarotsky (Neural Netw 94:103–114, 2017), formulating this (simple) approximation using mixed-integer programming (MIP). Notably, the number of constraints, binary variables, and auxiliary continuous variables used in this formulation grows logarithmically in the approximation error. Combining this with a diagonal perturbation technique to convert a nonseparable quadratic function into a separable one, we present a mixed-integer convex quadratic relaxation for nonconvex quadratic optimization problems. We study the strength (or sharpness ) of our formulation and the tightness of its approximation. Further, we show that our formulation represents feasible points via a Gray code. We close with computational results on problems with quadratic objectives and/or constraints, showing that our proposed method (i) across the board outperforms existing MIP relaxations from the literature, and (ii) on hard instances produces better bounds than exact solvers within a fixed time budget.
更多查看译文
关键词
Quadratic optimization, Nonconvex optimization, Mixed-integer programming, Gray Code
AI 理解论文
溯源树
样例
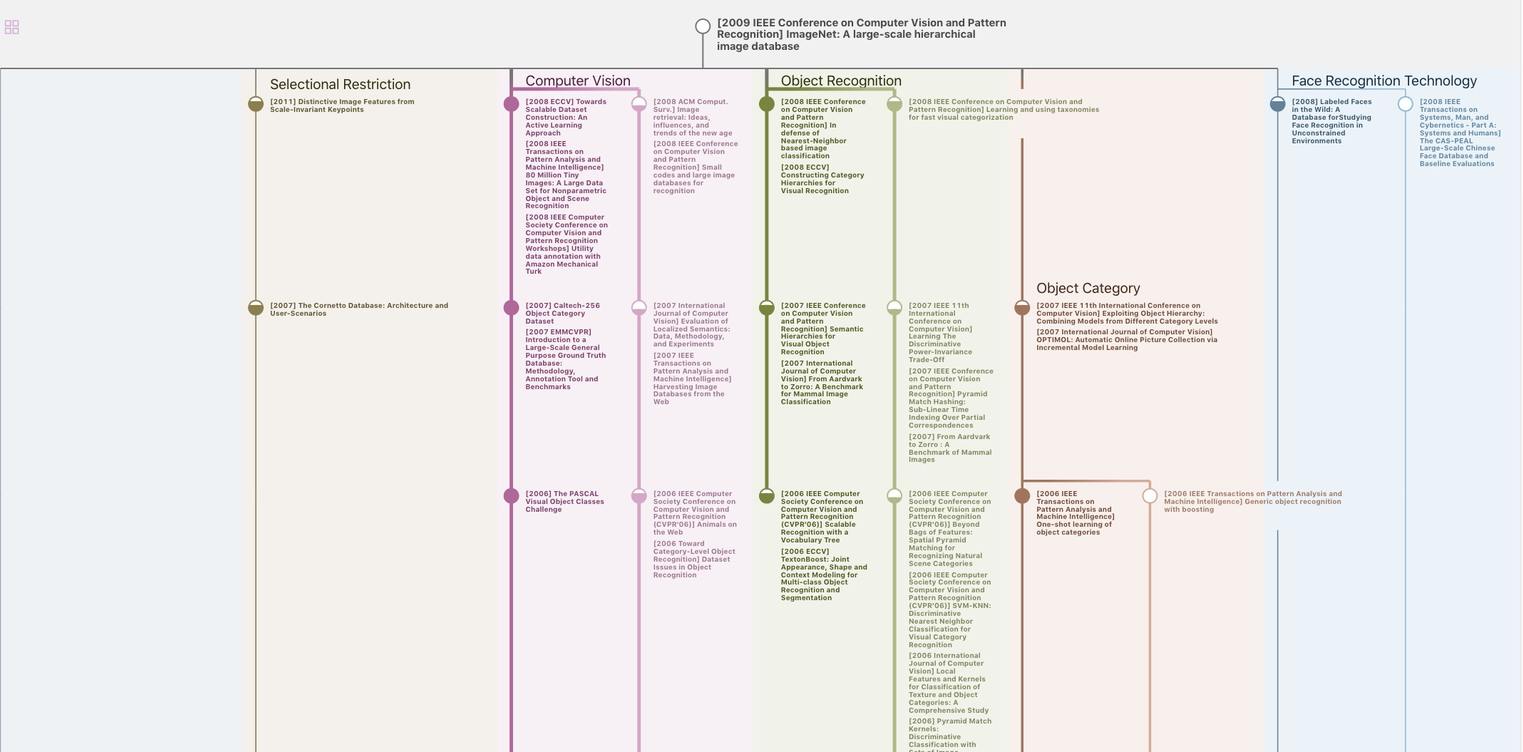
生成溯源树,研究论文发展脉络
Chat Paper
正在生成论文摘要