Application of multi-objective particle swarm optimization based on short-term memory and K-means clustering in multi-modal multi-objective optimization
Engineering Applications of Artificial Intelligence(2022)
摘要
To solve the multi-modal multi-objective optimization problems in which the same Pareto Front (PF) may correspond to multiple different Pareto Optimal Sets (PSs), an improved multi-objective particle swarm optimizer with short-term memory and K-means clustering (MOPSO-SMK) is proposed in this paper. According to the framework of multi-objective particle swarm optimization (MOPSO) algorithm, the designs of updating mechanism and population maintenance mechanism are the keys to obtain the optimal solutions. As a significant influence factor of the updating mechanism, the inertia weight has been discussed in this paper. In the improved algorithm, a new update model for the value of pbest based on short-term memory is proposed. The update strategies based on K-means clustering are adopted to obtain the better gbest and elite archive. 16 multi-modal multi-objective optimization functions are used to verify the feasibility and effectiveness of the proposed MOPSO-SMK. As the results show, MOPSO-SMK has more advantages in four indexes (1/PSP, 1/HV, IGDX, and IGDF) compared with other three multi-objective optimization algorithms.
更多查看译文
关键词
Multi-modal multi-objective,K-means clustering,Short-term memory,Dynamic inertia weight,Elite archiving
AI 理解论文
溯源树
样例
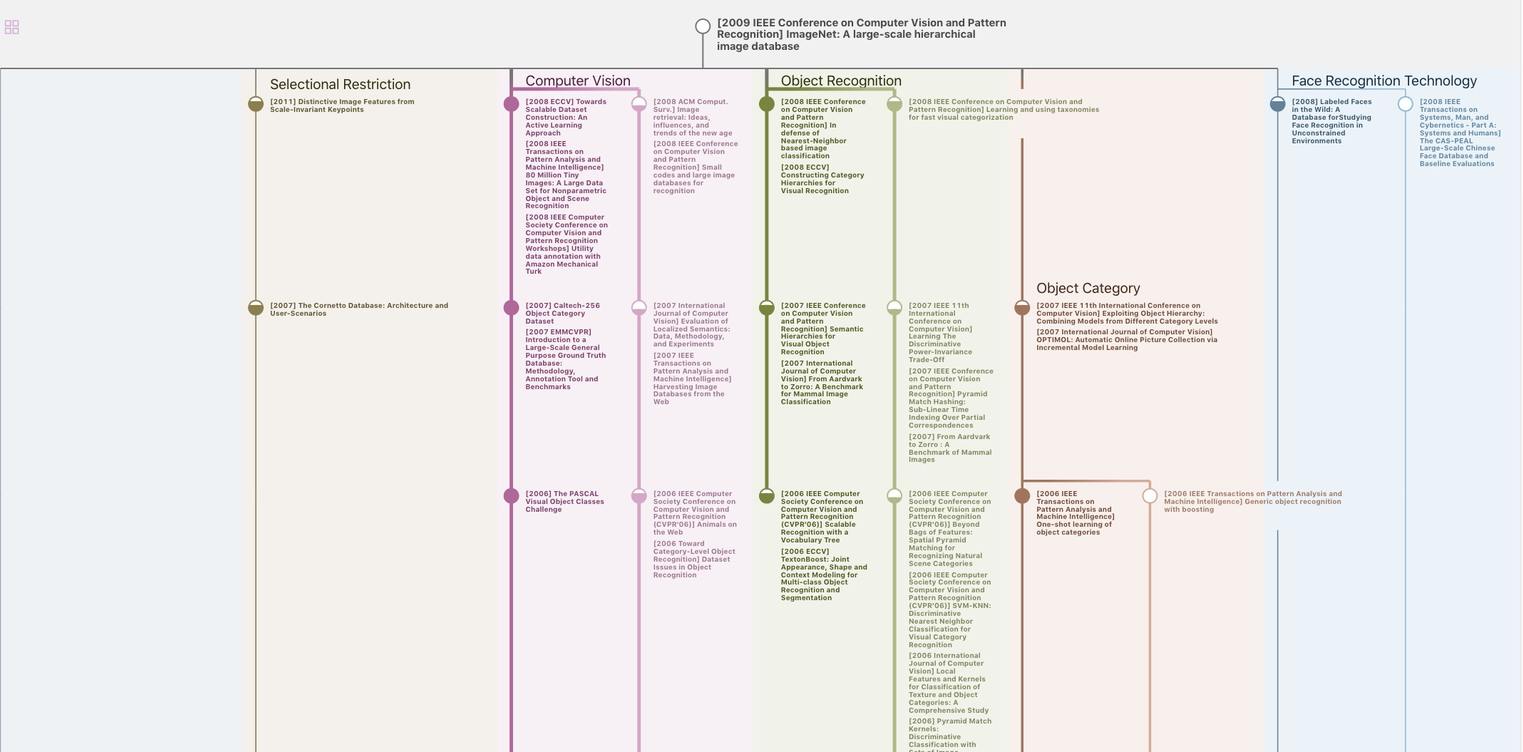
生成溯源树,研究论文发展脉络
Chat Paper
正在生成论文摘要