Data-driven agent-based model building for animal movement through Exploratory Data Analysis
Ecological Modelling(2022)
摘要
Fields studying animal movement are data-starved due to large monetary and time costs of data collection, and, moreover, not all quantities of interest can be measured. Despite limited data, diverse models have been developed to study animal movement. Many of these models are based on random walks that use Gaussian noise. Examinations of real movement data show that the assumptions made in these models are not always valid on all spatial and time scales, and these shortcomings suggest that new models may be needed. We provide methods for developing and training more realistic models, specifically agent-based models of animal movement, by taking a data-driven approach. We took an exploratory data analysis (EDA) approach that allowed us to develop a model that best reproduced the movement patterns we observed in a real dataset of animal locations. In our EDA, we examined distributions of positions, calculated the autocorrelation of the movement data, used Fourier analysis, calculated mean-squared displacements, and tested for correlations. We introduce the fused-lasso regression-analysis method for identifying large and sudden changes in position through a non-parametric fit that is sensitive to discontinuities. We introduce the copula and kernel-density estimates as methods for approximating coordinate-system-independent movement correlations from the marginal location differences represented in our data set; use of the copula allows us to create correlated, non-Gaussian noise. With the insights gained from our EDA, we created a Langevin model that describes the movements of an individual animal, features non-Gaussian noise and incorporates multiple movement patterns. We extended this model to an agent-based model that describes the movements of several groups of deer. We compared our Langevin model to three models built on different assumptions that result in substantial differences in the area covered by an animal. Using our agent-based model, we simulated three groups of deer with parameters sampled from our data to illustrate the amount of area covered by deer in our model and the ability of groups to overlap in space. This overlapping behavior is an important feature of models of processes driven by interactions between animals, such as infectious diseases; our model could be useful for studying the spread of infectious diseases in animal populations, such as chronic wasting disease. While we have applied our data-driven methods to animal movement, these methods are widely applicable to developing and training models using a wide range of data sources.
更多查看译文
关键词
Agent-based modeling,Exploratory Data Analysis,Non-Gaussian noise,Fused-lasso,Tracking data,Data-driven modeling
AI 理解论文
溯源树
样例
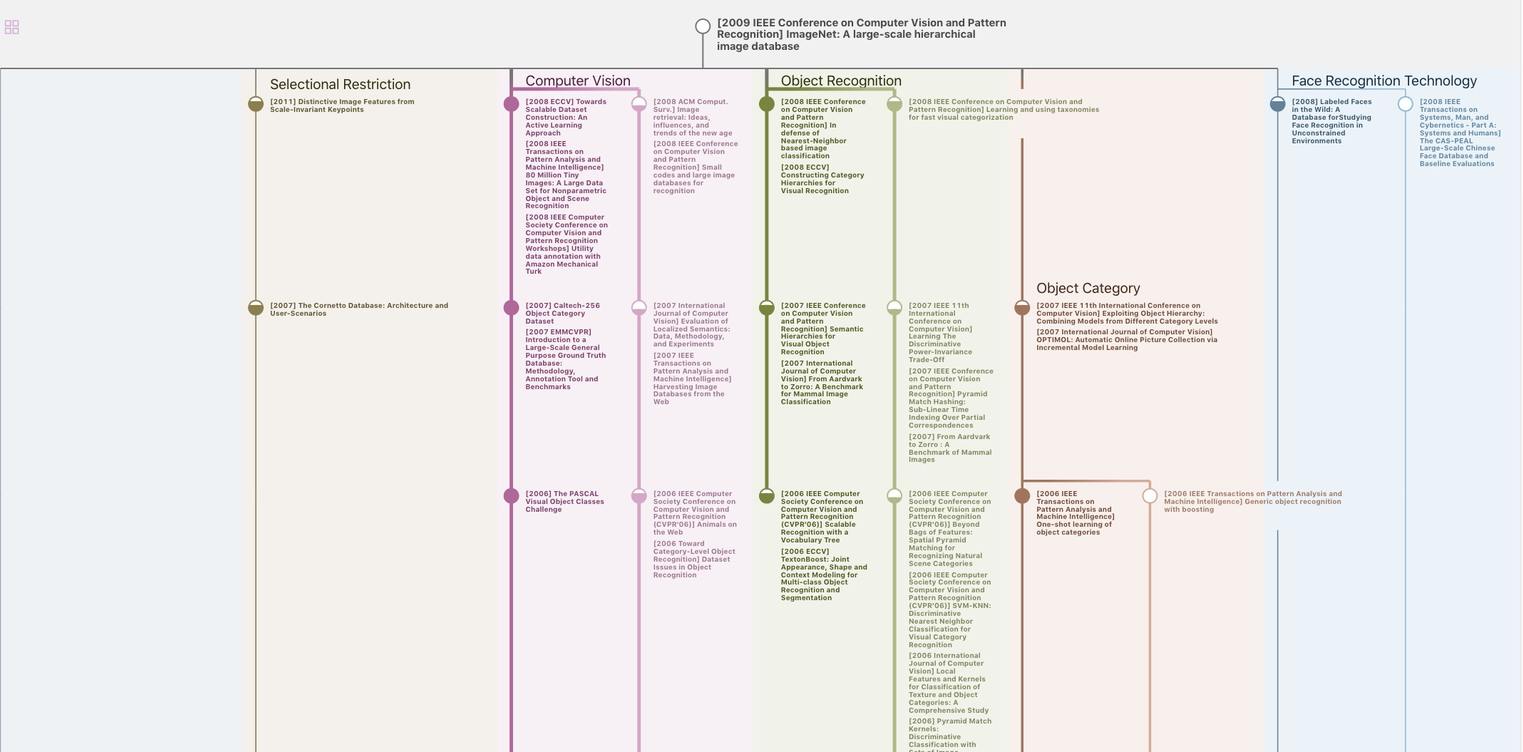
生成溯源树,研究论文发展脉络
Chat Paper
正在生成论文摘要