Clustering-based adaptive data augmentation for class-imbalance in machine learning (CADA): additive manufacturing use case
NEURAL COMPUTING & APPLICATIONS(2022)
摘要
Large amount of data are generated from in-situ monitoring of additive manufacturing (AM) processes which is later used in prediction modelling for defect classification to speed up quality inspection of products. A high volume of this process data is defect-free (majority class) and a lower volume of this data has defects (minority class) which result in the class-imbalance issue. Using imbalanced datasets, classifiers often provide sub-optimal classification results, i.e. better performance on the majority class than the minority class. However, it is important for process engineers that models classify defects more accurately than the class with no defects since this is crucial for quality inspection. Hence, we address the class-imbalance issue in manufacturing process data to support in-situ quality control of additive manufactured components. For this, we propose cluster-based adaptive data augmentation (CADA) for oversampling to address the class-imbalance problem. Quantitative experiments are conducted to evaluate the performance of the proposed method and to compare with other selected oversampling methods using AM datasets from an aerospace industry and a publicly available casting manufacturing dataset. The results show that CADA outperformed random oversampling and the SMOTE method and is similar to random data augmentation and cluster-based oversampling. Furthermore, the results of the statistical significance test show that there is a significant difference between the studied methods. As such, the CADA method can be considered as an alternative method for oversampling to improve the performance of models on the minority class.
更多查看译文
关键词
Class-imbalance,Melt-pool defects classification,Aerospace application,Additive manufacturing,Polar transformation,Random forests
AI 理解论文
溯源树
样例
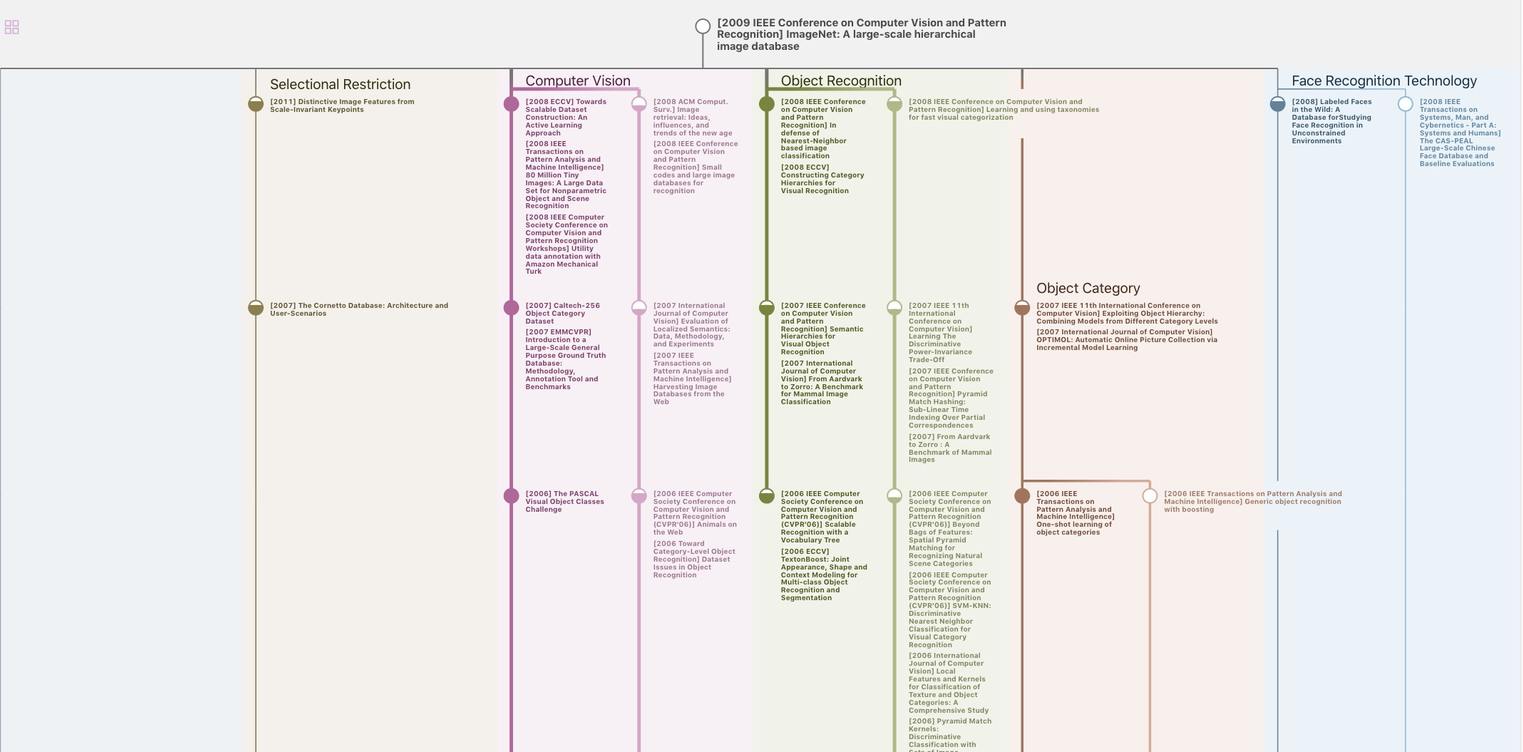
生成溯源树,研究论文发展脉络
Chat Paper
正在生成论文摘要