Deep-AIR: A Hybrid CNN-LSTM Framework for Fine-Grained Air Pollution Estimation and Forecast in Metropolitan Cities
IEEE ACCESS(2022)
摘要
Air pollution presents a serious health challenge in urban metropolises. While accurately monitoring and forecasting air pollution are highly crucial, existing data-driven models have yet fully captured the complex interactions between the temporal characteristics of air pollution and the spatial characteristics of urban dynamics. Our proposed Deep-AIR fills this gap to provide fine-grained city-wide air pollution estimation and station-wide forecast, by exploiting domain-specific features (including Air Pollution, Weather, Urban Morphology, Transport, and Time-sensitive features), with a hybrid CNN-LSTM structure to capture the spatio-temporal features, and 1 x 1 convolution layers to enhance the learning of temporal and spatial interaction. Deep-AIR outperforms compatible baselines by a higher accuracy of 1.5%, 2.7%, and 2.3% for Hong Kong and 1.4%, 1.4% and 3.3% for Beijing in fine-grained 1-hr pollution estimation, and 1-hr and 24-hr forecasts, respectively. Saliency analysis reveals that for Hong Kong, spatial features, including street canyon and road density, are the best predictors for NO2, while temporal features, including historical air pollutants and weather, are the best predictors for PM2.5. For Beijing, historical air pollutant data, traffic congestion, wind direction and seasonal indicator are the best predictors for all pollutants. PM10 in Hong Kong is achieving the best estimation and forecast accuracy, whilst CO in Beijing is achieving the best results.
更多查看译文
关键词
Fine-grained air pollution estimation and forecast, spatial-temporal data, deep learning, CNN-LSTM, street canyon effect, traffic speed, traffic congestion, domain-specific knowledge, saliency analysis, city-wide, station-wide, Hong Kong, Beijing
AI 理解论文
溯源树
样例
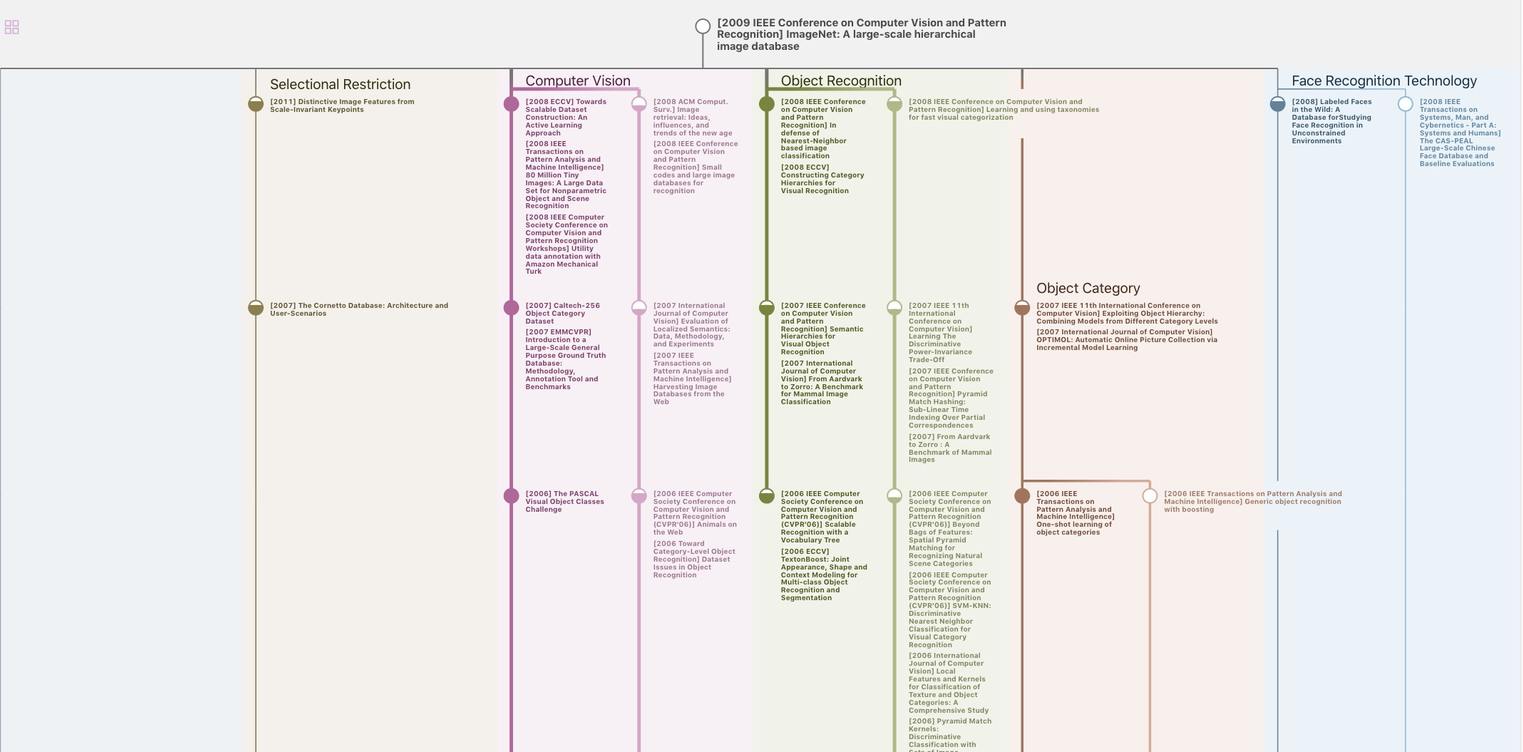
生成溯源树,研究论文发展脉络
Chat Paper
正在生成论文摘要