Robust Kernelized Multiview Clustering Based on High-Order Similarity Learning
IEEE ACCESS(2022)
摘要
This paper explores the robust kernelized multi-view clustering (MVC) for nonlinear data. The existing MVC methods aim to excavate the complementary and consensus information from multi-view data lies in the linear space for clustering. However, in real-world scenarios, data points usually lie in multiple nonlinear spaces, leading to undesirable clustering results. To this end, we propose a robust kernelized MVC method based on high-order similarity learning (RKHSL), to jointly learn the local structure affinities in original space, the nonlinear affinities in mapping kernel space, and the high-order correlations in tensor space. Specifically, the first-order similarity (FOS) is learned to excavate the local structure affinities and the second-order similarity (SOS) is learned in the high-dimensional kernel space to excavate the nonlinear affinities of data points. Afterwards, the third-order similarity (TOS) based on low-rank tensor is learned to excavate the global consistency from multiple views. Extensive experiments on five commonly benchmark datasets show that the proposed method outperforms state-of-the-art methods in most scenarios and is capable of revealing a reliable affinity graph structure concealed in different data points.
更多查看译文
关键词
Tensors,Kernel,Manifolds,Data models,Correlation,Software,Representation learning,Multi-view clustering,high-order similarity,low-rank tensor learning,kernel method
AI 理解论文
溯源树
样例
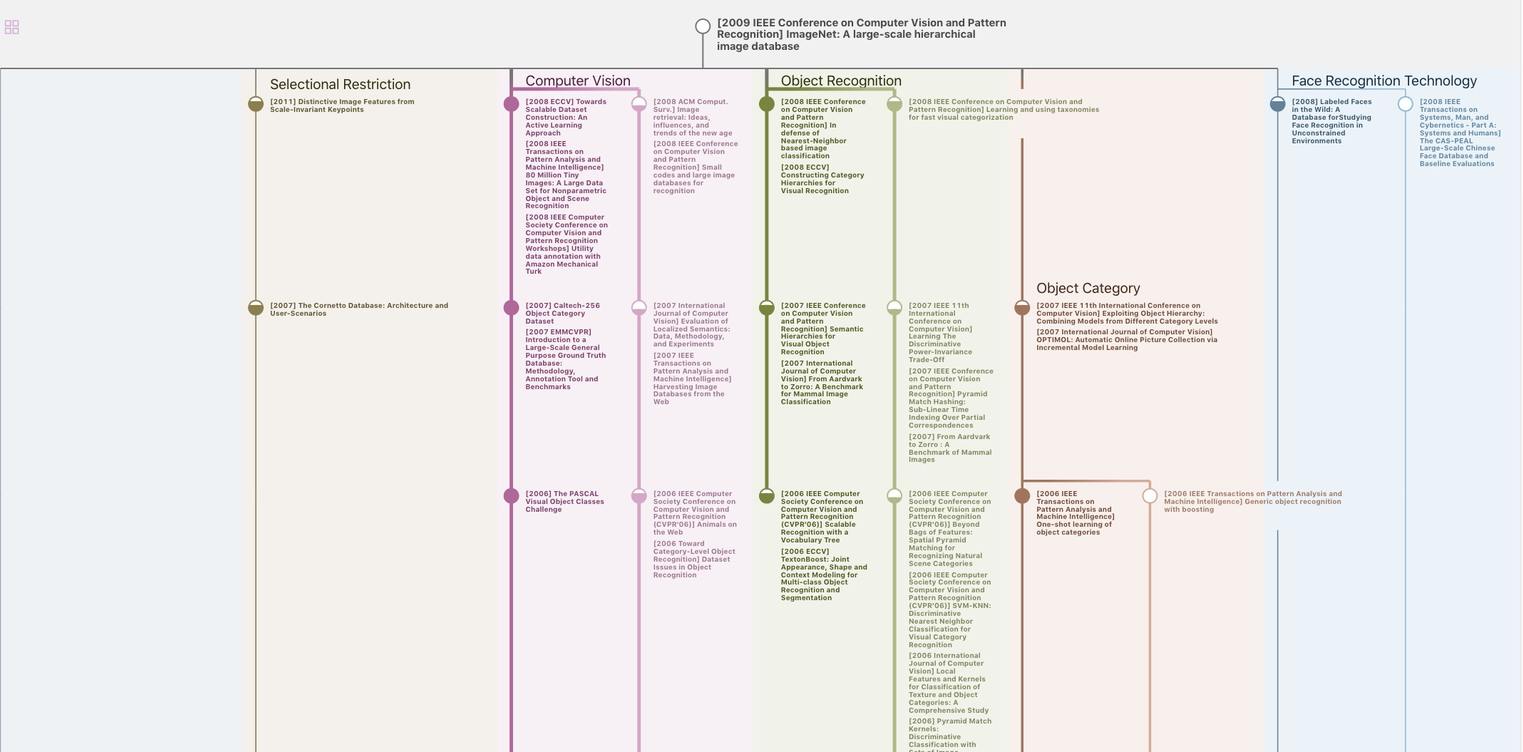
生成溯源树,研究论文发展脉络
Chat Paper
正在生成论文摘要