Hybrid Feature Extraction Model to Categorize Student Attention Pattern and Its Relationship with Learning
ELECTRONICS(2022)
摘要
The increase of instructional technology, e-learning resources, and online courses has created opportunities for data mining and learning analytics in the pedagogical domain. A large amount of data is obtained from this domain that can be analyzed and interpreted so that educators can understand students' attention. In a classroom where students have their own computers in front of them, it is important for instructors to understand whether students are paying attention. We collected on- and off-task data to analyze the attention behaviors of students. Educational data mining extracts hidden information from educational records, and we are using it to classify student attention patterns. A hybrid method is used to combine various techniques like classifications, regressions, or feature extraction. In our work, we combined two feature extraction techniques: principal component analysis and linear discriminant analysis. Extracted features are used by a linear and kernel support vector machine (SVM) to classify attention patterns. Classification results are compared with linear and kernel SVM. Our hybrid method achieved the best results in terms of accuracy, precision, recall, F1, and kappa. Also, we correlated attention with learning. Here, learning corresponds to tests and a final course grade. For determining the correlation between grades and attention, Pearson's correlation coefficient and p-value were used.
更多查看译文
关键词
hybrid feature extraction, educational data mining, correlation, learning analytics, attention pattern
AI 理解论文
溯源树
样例
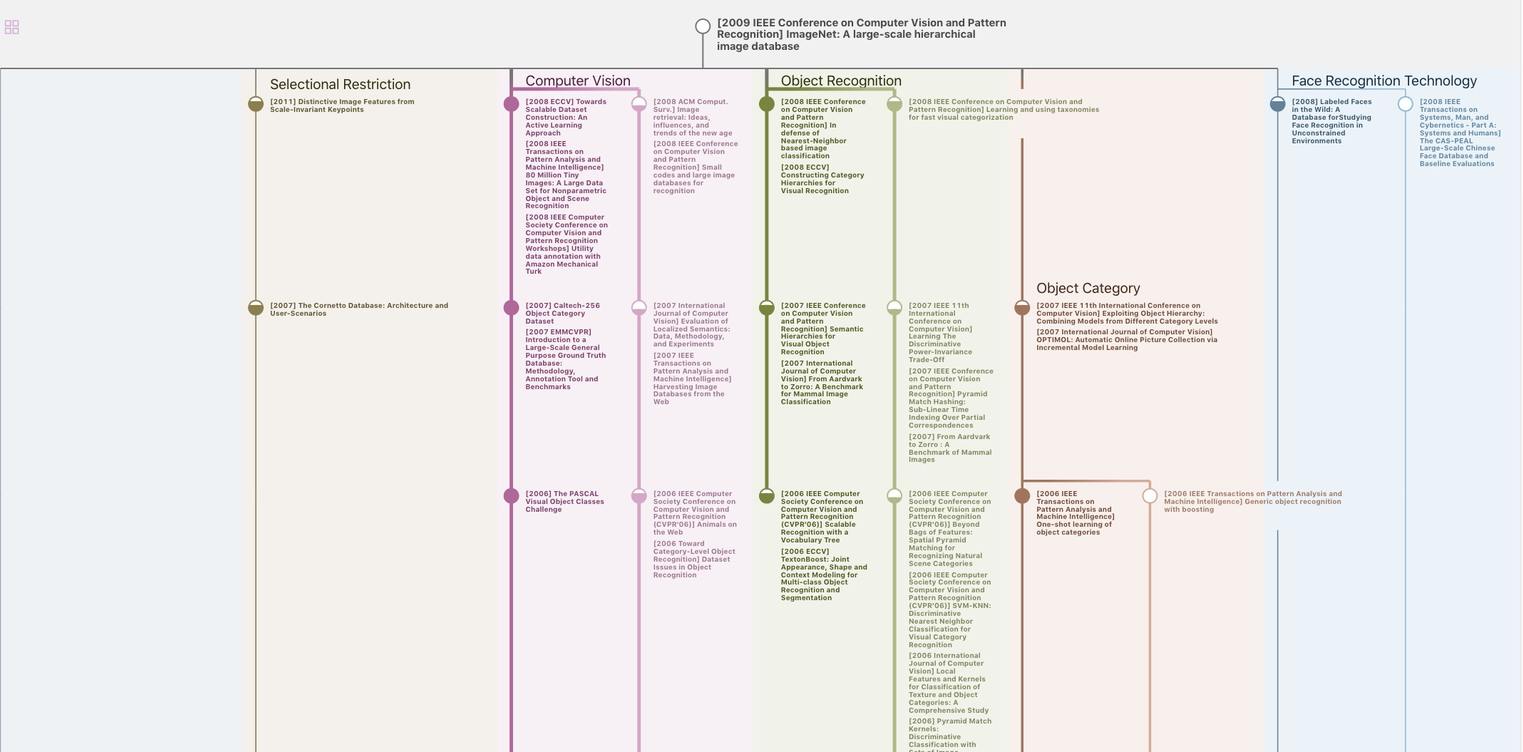
生成溯源树,研究论文发展脉络
Chat Paper
正在生成论文摘要