Deep Learning-Based Pulse Height Estimation for Separation of Pile-Up Pulses From NaI(Tl) Detector
IEEE Transactions on Nuclear Science(2022)
摘要
Measured spectra in a high count rate environment are difficult to analyze because of the spectral distortions caused by the pulse pile-up effect. This study proposes a deep learning-based method for separating and predicting the true pulse height of a signal with pulse pile-up events for application to radiation measurement and spectroscopy with a scintillation detector. To train the deep learning model, pulse signals simulating scintillation pulses were prepared by using a predefined mathematical model with parameters determined by analysis of the scintillation pulse measured from a NaI(Tl) detector. To simulate realistic scintillation pulses, Gaussian noises corresponding to thermal and shot noises were added to the signals. The trained model was validated with signals measured from two gamma-ray sources,
$^{\mathbf {22}}$
Na and
$^{\mathbf {137}}$
Cs. The model was then evaluated using two performance indicators, restoration and separation rates, which represent how much the net count is restored in the region of interest (ROI) and the separation accuracy depending on the time interval. As a result, the deep learning model was confirmed to correctly estimate the pulse heights in a high pile-up environment up to certain restoration and separation rates.
更多查看译文
关键词
Convolutional neural network,deep learning,pile-up correction,pulse height estimation
AI 理解论文
溯源树
样例
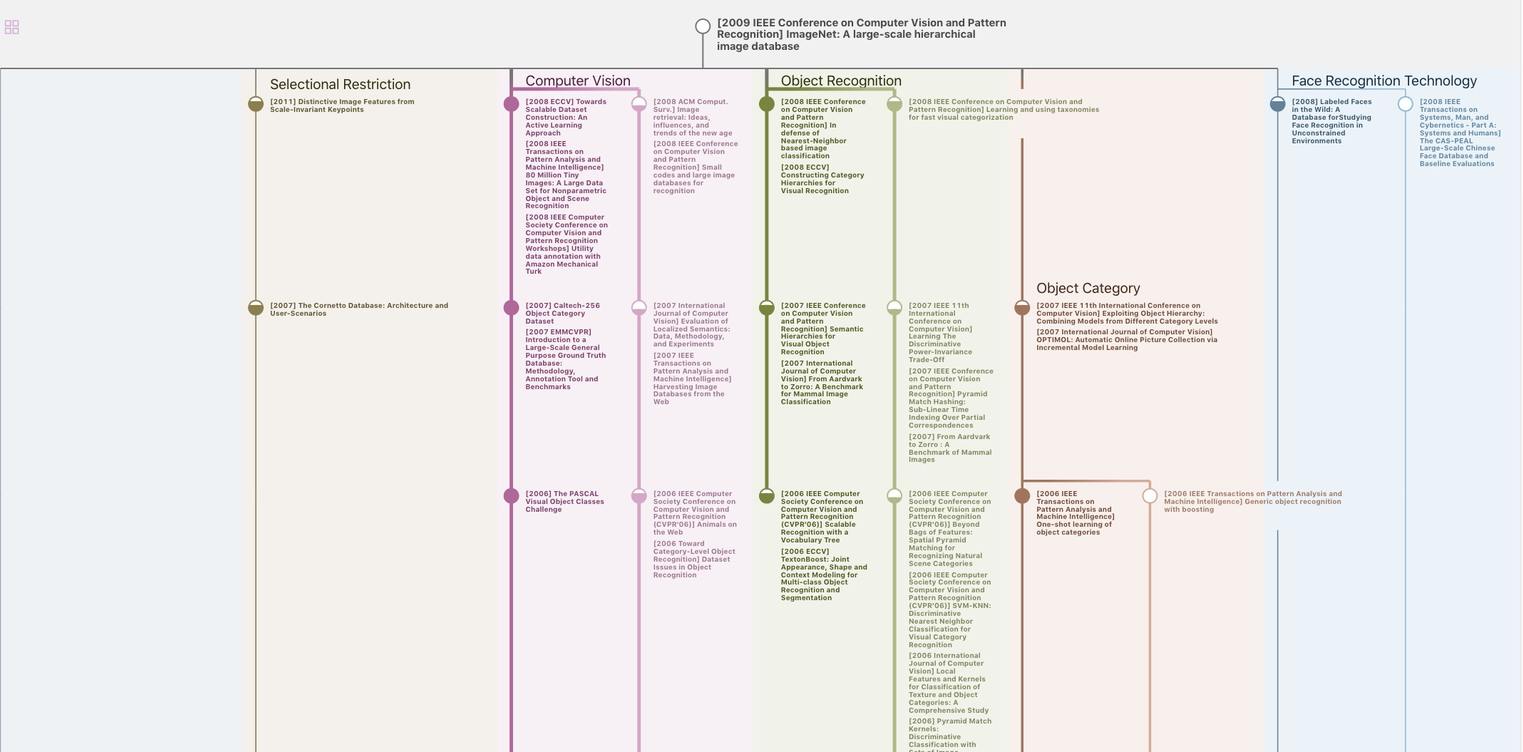
生成溯源树,研究论文发展脉络
Chat Paper
正在生成论文摘要