An effective pest detection method with automatic data augmentation strategy in the agricultural field
SIGNAL IMAGE AND VIDEO PROCESSING(2022)
摘要
Currently, computer vision technology has been applied to detect and recognize pests for integrated pest management (IPM). Recent studies have shown that the accuracy of pest detection and recognition has been rapidly improved with the development of deep learning. However, complex backgrounds, various poses, and different scales among insect species in the field will aggravate the difficulty of pest detection. To address the pest detection and recognition problem in wild field, in this paper, we firstly devise a novel automatic data augmentation method to search for the appropriate augmentation strategy adaptively and model data more effectively. Secondly, Res2Net is used as backbone for obtaining richer detailed information of small pest, and a reverse feature fusion layer is introduced into feature pyramid networks (FPN) to learn more details. During network training, the CIoU bounding box regression loss function and cross entropy loss after label smoothing are introduced for accurate localization and recognition of small pests. When testing, the test time augmentation (TTA) strategy is used to further improve pest detection performance and reduce the probability of missing detection by inferring pest images at different scales. We evaluate the performance of our method on the pest dataset including 4 k images and 4 classes (wheat sawfly, wheat aphid, wheat mite and rice planthopper). Our method achieves the pest detection performance of 81.0% mean Average Precision (mAP), which improves 5.7%, 4.0% and 3.1% compared to three state-of-the-art approaches YOLOv4, Faster R-CNN, and Cascade R-CNN detectors, respectively.
更多查看译文
关键词
Deep learning, Pest detection and recognition, Data augmentation, Computer vision
AI 理解论文
溯源树
样例
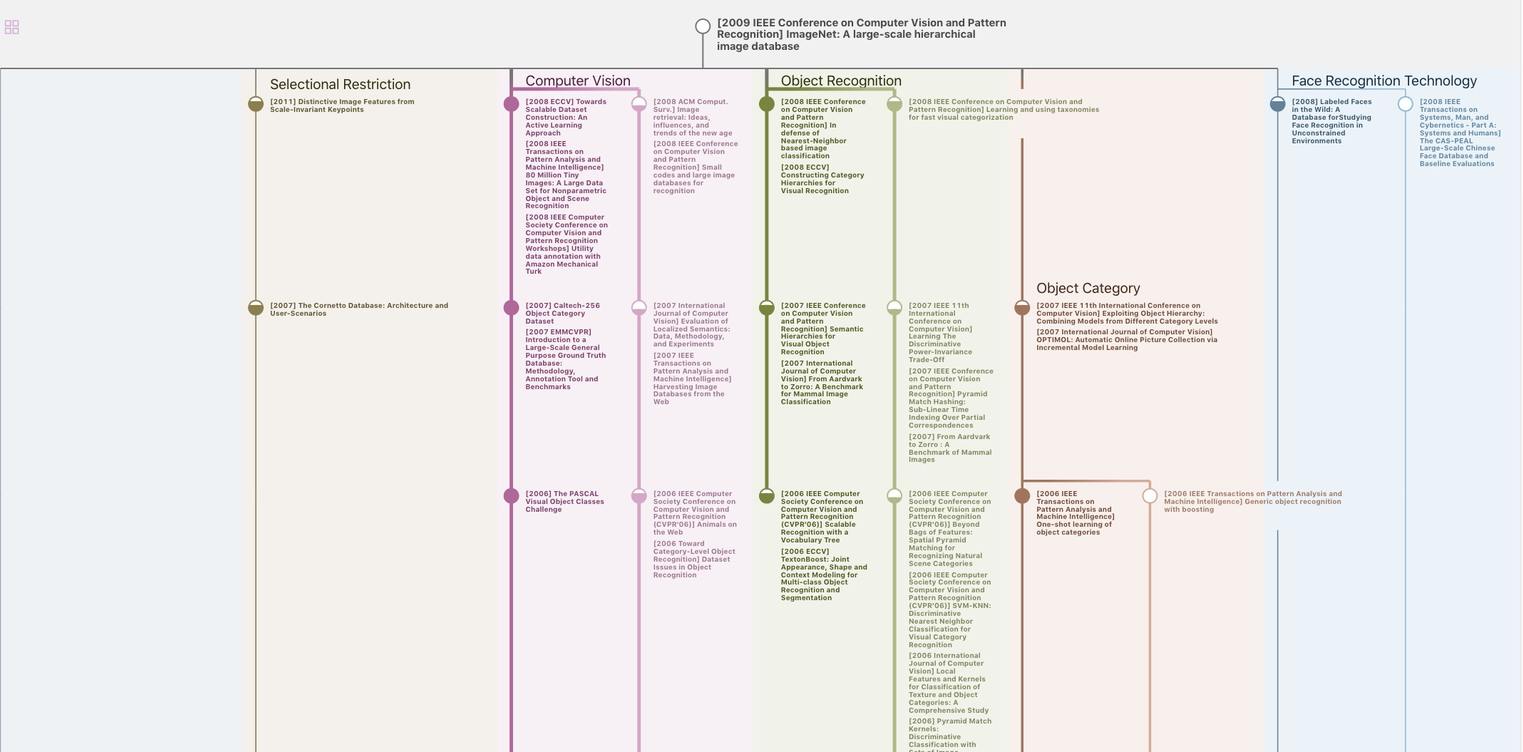
生成溯源树,研究论文发展脉络
Chat Paper
正在生成论文摘要