Accurate prediction of salmon freshness under temperature fluctuations using the convolutional neural network long short-term memory model
Journal of Food Engineering(2022)
摘要
Freshness prediction was a research hotspot in the field of food science. The current microbial kinetic equations could predict the freshness under certain fixed temperature conditions, but they were no longer effective when the temperature was fluctuated. To solve this problem, this paper used deep learning techniques to mine the inherent relation of variable temperature during storage and proposed a novel model named CNN_LSTM (convolutional neural network_ long short-term memory). The model didn't need to fit the parameters of a fixed equation, and it had the advantage of predicting freshness within a range of temperature fluctuations. The results showed that CNN_LSTM could get better prediction results than classic microbial kinetics methods such as logistic equation, Gompertz equation and Arhenius equation under fixed temperature conditions. When the temperature fluctuated, the model could still accurately predict total viable counts (TVC) under variable temperature conditions, with the determination coefficient (R2) greater than 0.95 and the root mean square error (RMSE) less than 0.2. In addition, the model had the potential to predict freshness under different change factors besides temperature fluctuations, which provided a new prospect for freshness prediction.
更多查看译文
关键词
Freshness,Temperature fluctuation,CNN_LSTM,Salmon
AI 理解论文
溯源树
样例
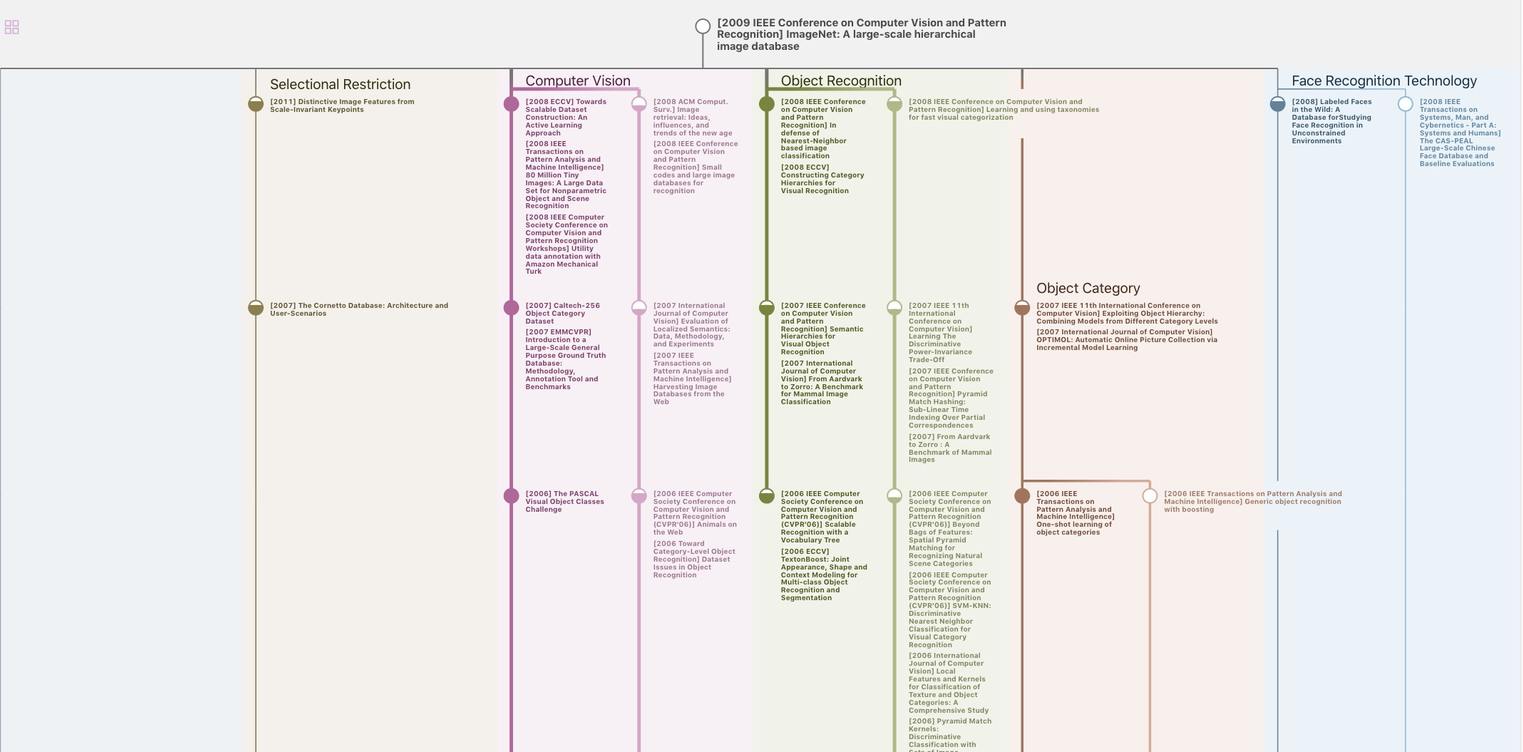
生成溯源树,研究论文发展脉络
Chat Paper
正在生成论文摘要