Epilepsy detection from electroencephalogram signal using singular value decomposition and extreme learning machine classifier
INTERNATIONAL JOURNAL OF BIOMEDICAL ENGINEERING AND TECHNOLOGY(2022)
摘要
Automatic detection of seizure plays an important role for both long-term monitoring and diagnosis of epilepsy. In this work, the proposed singular value decomposition-extreme learning machine (SVD-ELM) classifier technique provide good generalised performance with a remarkable fast learning speed in comparison to existing conventional techniques. Here, both feature extraction and classification of EEG signal has been done for detection of epileptic seizure of human brain, taking Bonn University dataset. Proposed method is based upon the multi-scale eigenspace analysis of the matrices generated using discrete wavelet transform (DWT) of EEG signal by SVD at substantial scale and are classified using extracted singular value features and extreme learning machine (ELM) with dissimilar activation functions. The proposed SVD-ELM technique has been applied for the first time on EEG signal for epilepsy detection using five class classification which produces overall accuracy of 95% (p < 0.001) with sine and radbas activation function.
更多查看译文
关键词
electroencephalogram, EEG, epilepsy, discrete wavelet transform, DWT, singular value decomposition, SVD, extreme learning machine, ELM, eigenvalue, EEG classification, neurons, activation functions
AI 理解论文
溯源树
样例
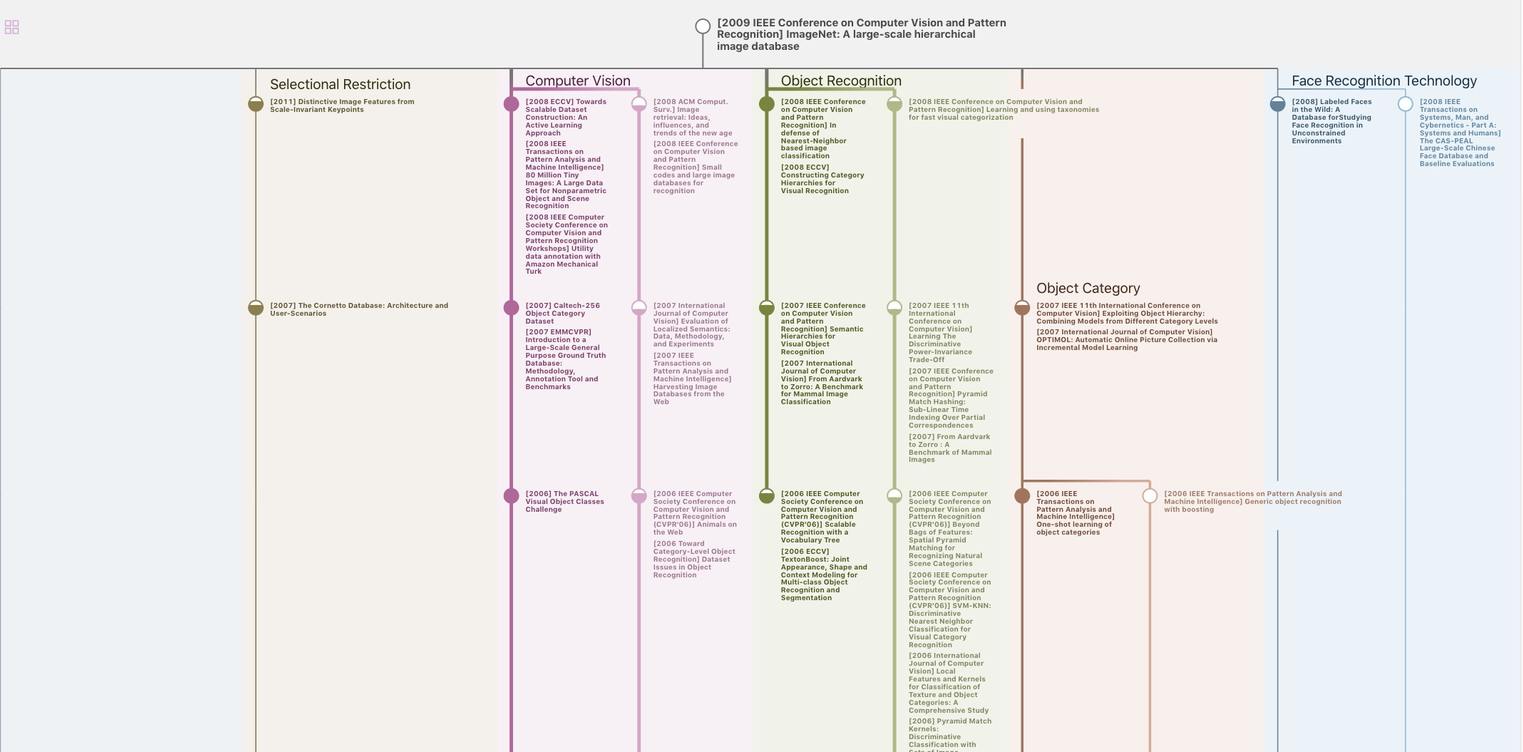
生成溯源树,研究论文发展脉络
Chat Paper
正在生成论文摘要