Mapping Within-Field Soil Health Variations Using Apparent Electrical Conductivity, Topography, and Machine Learning
AGRONOMY-BASEL(2022)
摘要
High-resolution maps of soil health measurements could help farmers finetune input resources and management practices for profit maximization. Within-field soil heath variations can be mapped using local topography and apparent electrical conductivity (ECa) as predictors. To address these issues, a study was conducted in Texas Blackland Prairie soils with the following objectives: (i) to assess and map within-field soil health variations using machine learning; (ii) to evaluate the usefulness of topography and ECa as soil health predictors; and (iii) to quantify the relationship between ECa and soil health index and use ECa to estimate soil health spatial distribution. We collected 218 topsoil (0-15 cm) samples following a 35 m x 35 m grid design and analyzed for one-day CO2, organic C, organic N, and soil health index (SHI) based on the Haney Soil Health Tool. A random forest model was applied to predict and map those properties on a 5 m x 5 m grid where ECa, and terrain attributes were used as predictors. Furthermore, the empirical relationship between SHI and ECa was established and mapped across the field. Results showed that the study area was variable in terms of one-day CO2, organic C, organic N, SHI, and ECa distribution. The ECa, wetness index, multiresolution valley bottom flatness, and topographic position index were among the top predictors of soil health measurements. The model was sufficiently robust to predict one day CO2, organic C, organic N (R-2 between 0.24-0.90), and SHI (R-2 between 0.47-0.90). Overall, we observed a moderate to strong spatial dependency of soil health measurements which could impact within-field yield variability. The study confirmed the applicability of easy to obtain ECa as a good predictor of SHI, and the predicted maps at high resolution which could be useful in site-specific management decisions within these types of soils.
更多查看译文
关键词
digital soil mapping, ECa, precision agriculture, random forests, soil quality, terrain attributes
AI 理解论文
溯源树
样例
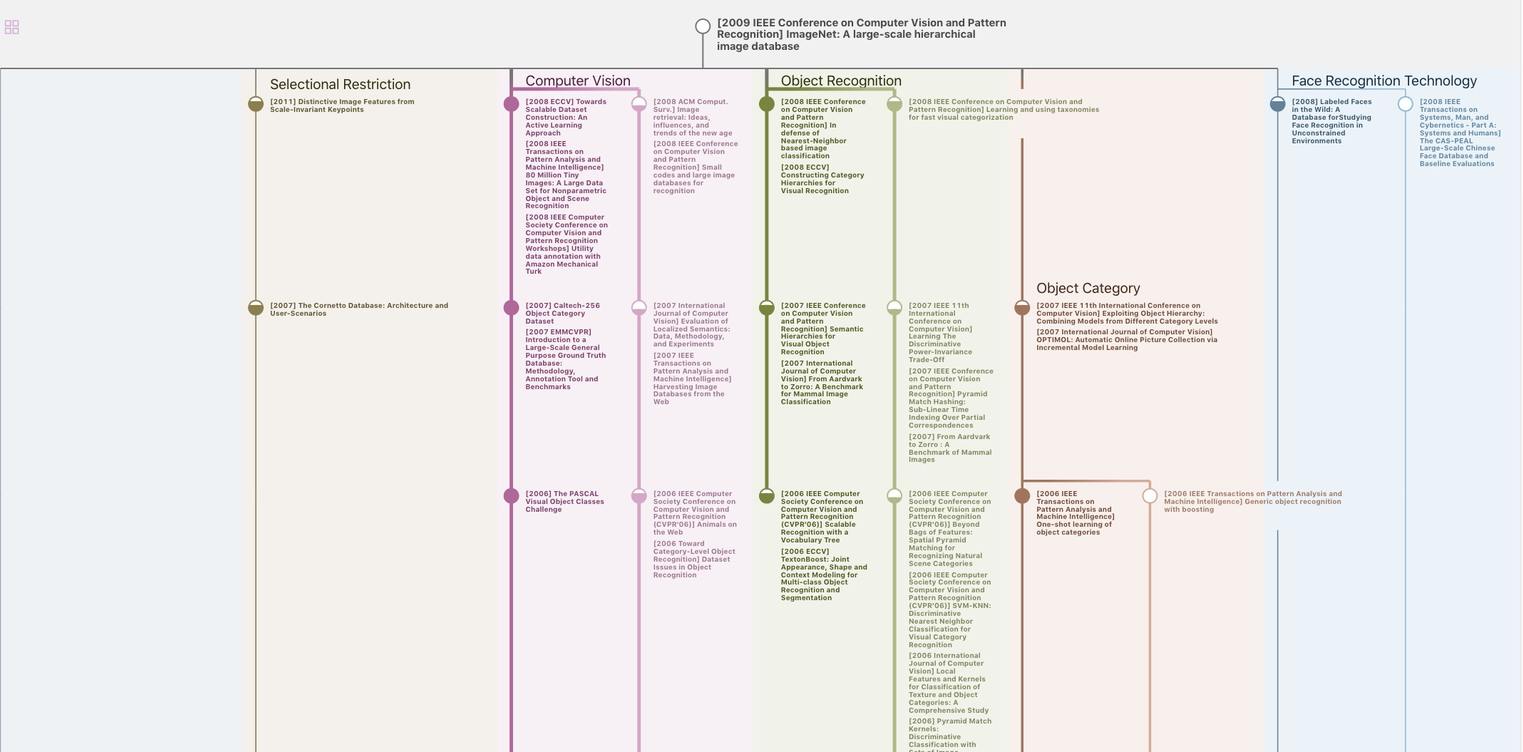
生成溯源树,研究论文发展脉络
Chat Paper
正在生成论文摘要