A Systematic Mapping Study and Empirical Comparison of Data-Driven Intrusion Detection Techniques in Industrial Control Networks
Archives of Computational Methods in Engineering(2022)
摘要
A rising communication between modern industrial control infrastructure and the external Internet worldwide has led to a critical need to secure the network from multifarious cyberattacks. An intrusion detection system (IDS) is a preventive mechanism where new sorts of hazardous threats and malicious activities could be detected before harming the industrial process’s critical infrastructure. This study reviews the cutting-edge technology of artificial intelligence in developing IDS in industrial control networks by carrying out a systematic mapping study. We included 74 foremost publications from the current literature. These chosen publications were grouped following the types of learning tasks, i.e., supervised, unsupervised, and semi-supervised. This review article helps researchers understand the present status of artificial intelligence techniques applied to IDS in industrial control networks. Other mapping categories were also covered, including year published, publication venues, dataset considered, and IDS approaches. This study reports an empirical assessment of several classification algorithms such as random forest, gradient boosting machine, extreme gradient boosting machine, deep neural network, and stacked generalization ensemble. Statistical significance tests were also used to assess the classifiers’ performance differences in multiple scenarios and datasets. This paper provides a contemporary systematic mapping study and empirical evaluation of IDS approaches in industrial control networks.
更多查看译文
关键词
industrial control networks,systematic mapping study,data-driven
AI 理解论文
溯源树
样例
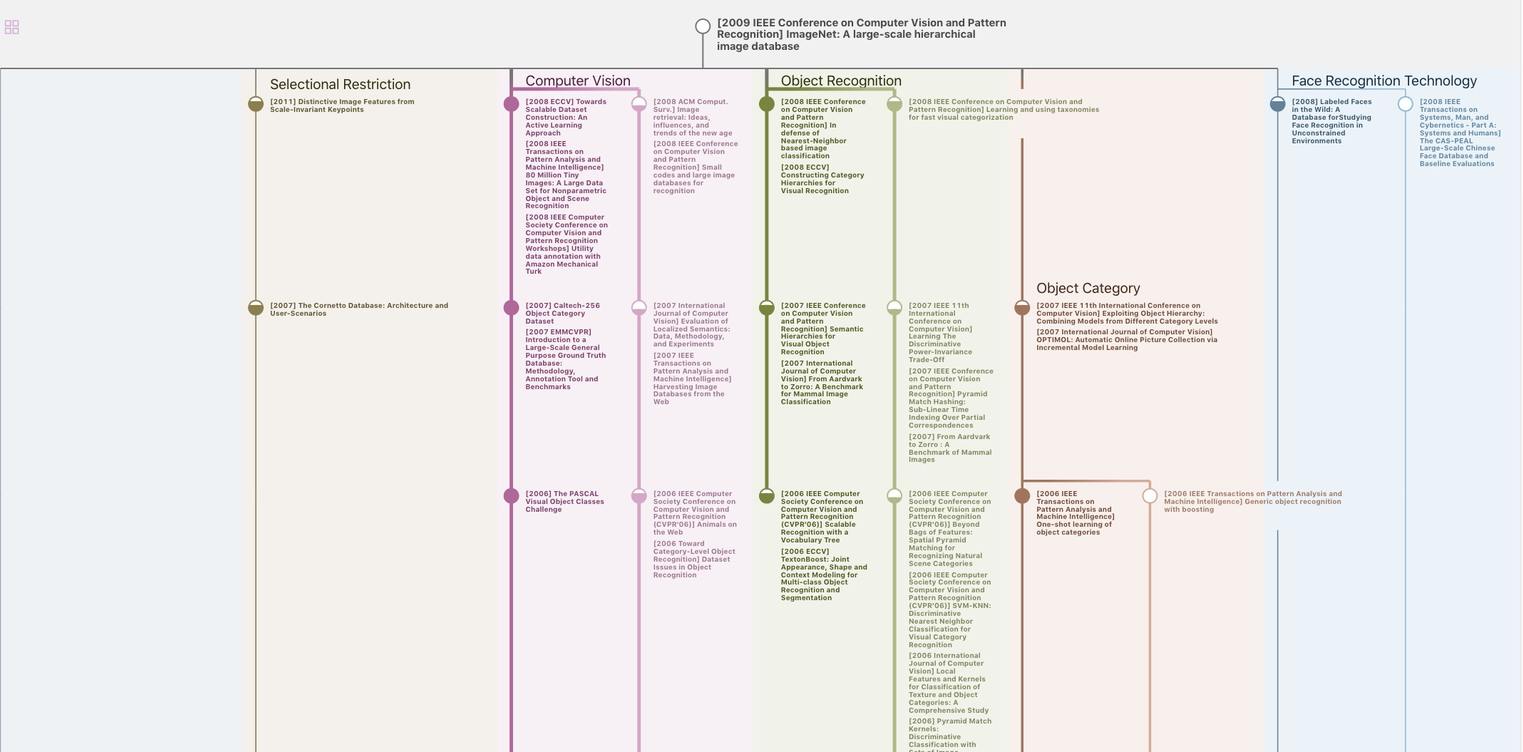
生成溯源树,研究论文发展脉络
Chat Paper
正在生成论文摘要