Double information preserving canonical correlation analysis
Engineering Applications of Artificial Intelligence(2022)
摘要
The methods based on canonical correlation analysis (CCA-based methods) are typical and effective methods for unsupervised dimensionality reduction of multi-view data. However, the traditional CCA-based methods ignore the dissimilarity information while considering the similarity information of samples, which will make the heterogeneous samples in the subspace cannot be well separated. In this paper, we propose a novel unsupervised multi-view dimensionality reduction method: Double Information Preserving Canonical Correlation Analysis (DIPCCA). DIPCCA aims at finding two projection matrices by integrating two cross double weight graphs with CCA to explore the similarity and dissimilarity information of data in cross views. Furthermore, on the basis of consistency and complementarity, DIPCCA uses the similarity information to maintain the local structure, and the dissimilarity information to disperse embedded samples of distinct clusters, so as to extract more discriminative features. Moreover, CCA and a new locality-preserving CCA are two special cases of DIPCCA when parameters take special values. In order to better extract the features of nonlinear data and more than two views, DIPCCA is extended to Double Information Preserving Kernel Canonical Correlation Analysis and Double Information Preserving Multiple Canonical Correlation Analysis, respectively. Experiments on an artificial dataset and three real multi-view datasets show our proposed methods have better performance than the traditional CCA-based methods.
更多查看译文
关键词
Unsupervised learning,Multi-view learning,Dimensionality reduction,Similarity and dissimilarity information
AI 理解论文
溯源树
样例
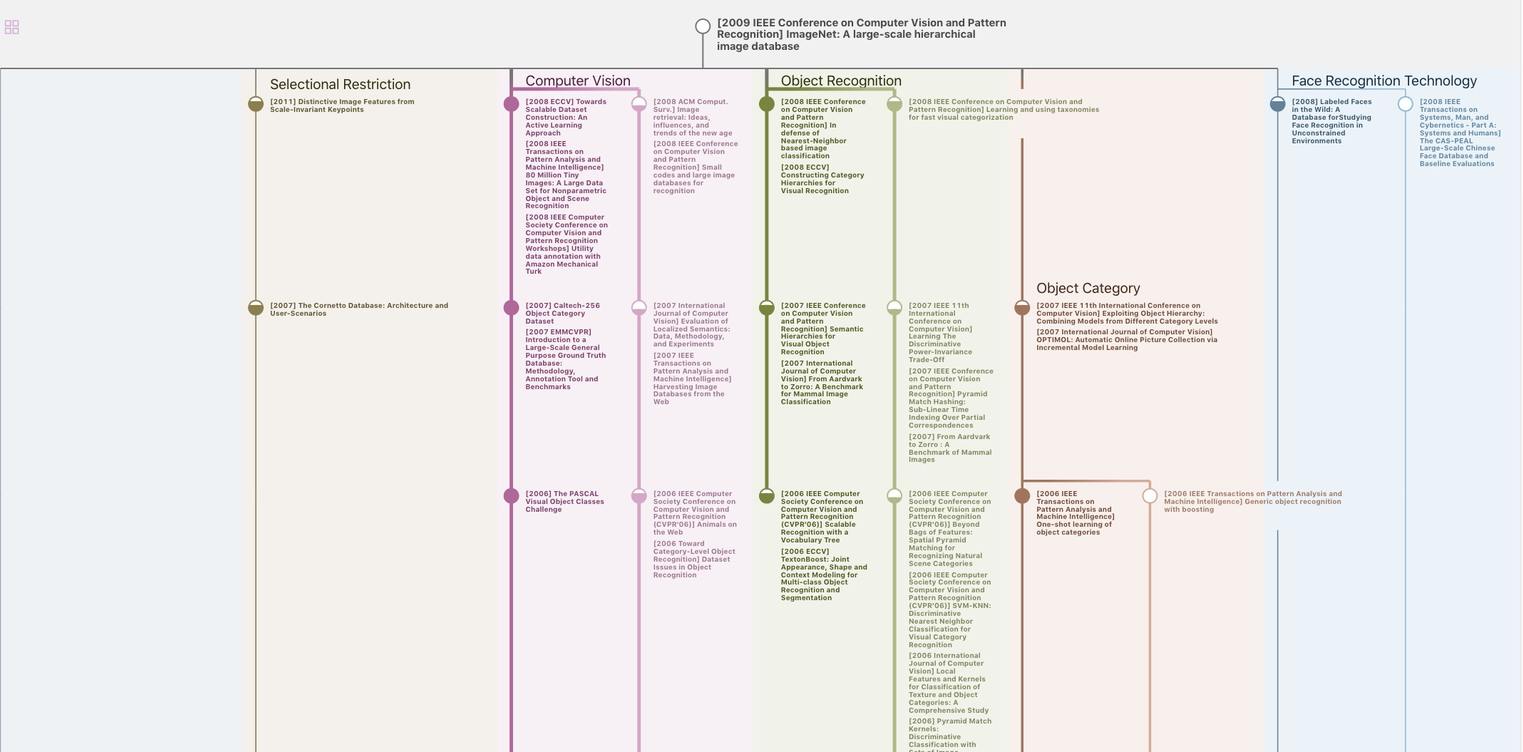
生成溯源树,研究论文发展脉络
Chat Paper
正在生成论文摘要