SEM: Safe Exploration Mask for Q-Learning
Engineering applications of artificial intelligence(2022)
Abstract
Most reinforcement learning algorithms focus on discovering the optimal policy to maximize reward while neglecting the safety issue during the exploration stage, which is not acceptable in industrial applications. This paper concerns the efficient method to improve the safety of the agent during the exploration stage in q-learning without any prior knowledge. We propose a novel approach named safe exploration mask to reduce the number of safety violations in q-learning by modifying the transition possibility of the environment. To this end, a safety indicator function consisting of distance metric and controllability metric is designed. The safety indicator function can be learned by the agent through bootstrapping without additional optimization solver. We prove that the safety indicator function will converge in tabular q-learning and introduce two tricks to mitigate the divergence in approximation-based q-learning. Based on the safety indicator function, the safe exploration mask is generated to modify the original exploration policy by reducing the transition possibility of unsafe actions. Finally, the simulations in both discrete and continuous environments demonstrate the advantages, feasibility, and safety of our method in both discrete and continuous q-learning algorithms.
MoreTranslated text
Key words
Reinforcement learning,Safe exploration,Fuzzy Q-learning,Safe reinforcement learning
AI Read Science
Must-Reading Tree
Example
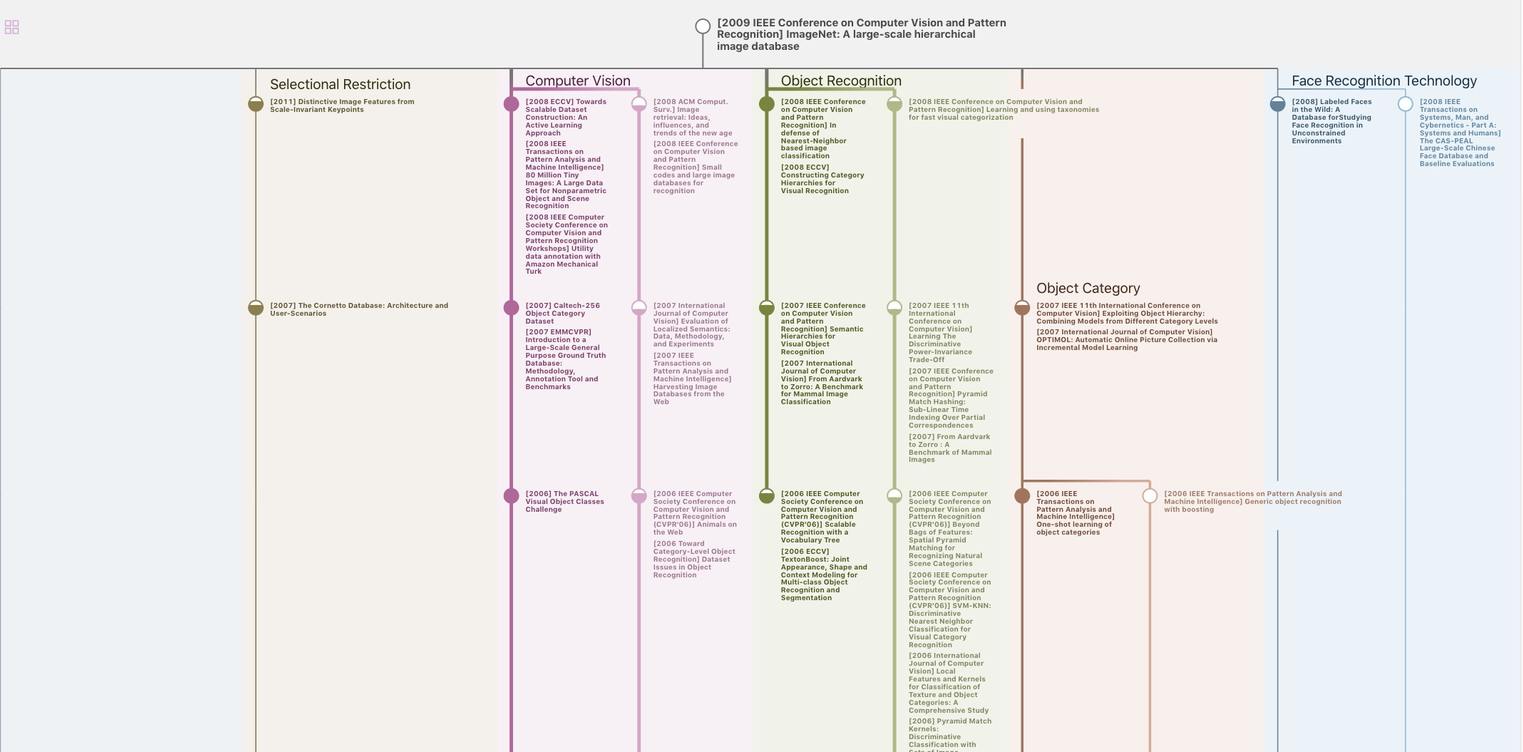
Generate MRT to find the research sequence of this paper
Chat Paper
Summary is being generated by the instructions you defined