WDBM: Weighted Deep Forest Model Based Bearing Fault Diagnosis Method
CMC-COMPUTERS MATERIALS & CONTINUA(2022)
摘要
In the research field of bearing fault diagnosis, classical deep learning models have the problems of too many parameters and high computing cost. In addition, the classical deep learning models are not effective in the scenario of small data. In recent years, deep forest is proposed, which has less hyper parameters and adaptive depth of deep model. In addition, weighted deep forest (WDF) is proposed to further improve deep forest by assigning weights for decisions trees based on the accuracy of each decision tree. In this paper, weighted deep forest model-based bearing fault diagnosis method (WDBM) is proposed. The WDBM is regard as a novel bearing fault diagnosis method, which not only inherits the WDF's advantages-strong and so on, but also realizes effective diagnosis with high precision and low cost under the condition of small samples. To verify the performance of the WDBM, experiments are carried out on Case Western Reserve University bearing data set (CWRU). Experiments results demonstrate that WDBM can achieve comparative recognition accuracy, with less computational overhead and faster convergence speed.
更多查看译文
关键词
Deep forest, bearing fault diagnosis, weights
AI 理解论文
溯源树
样例
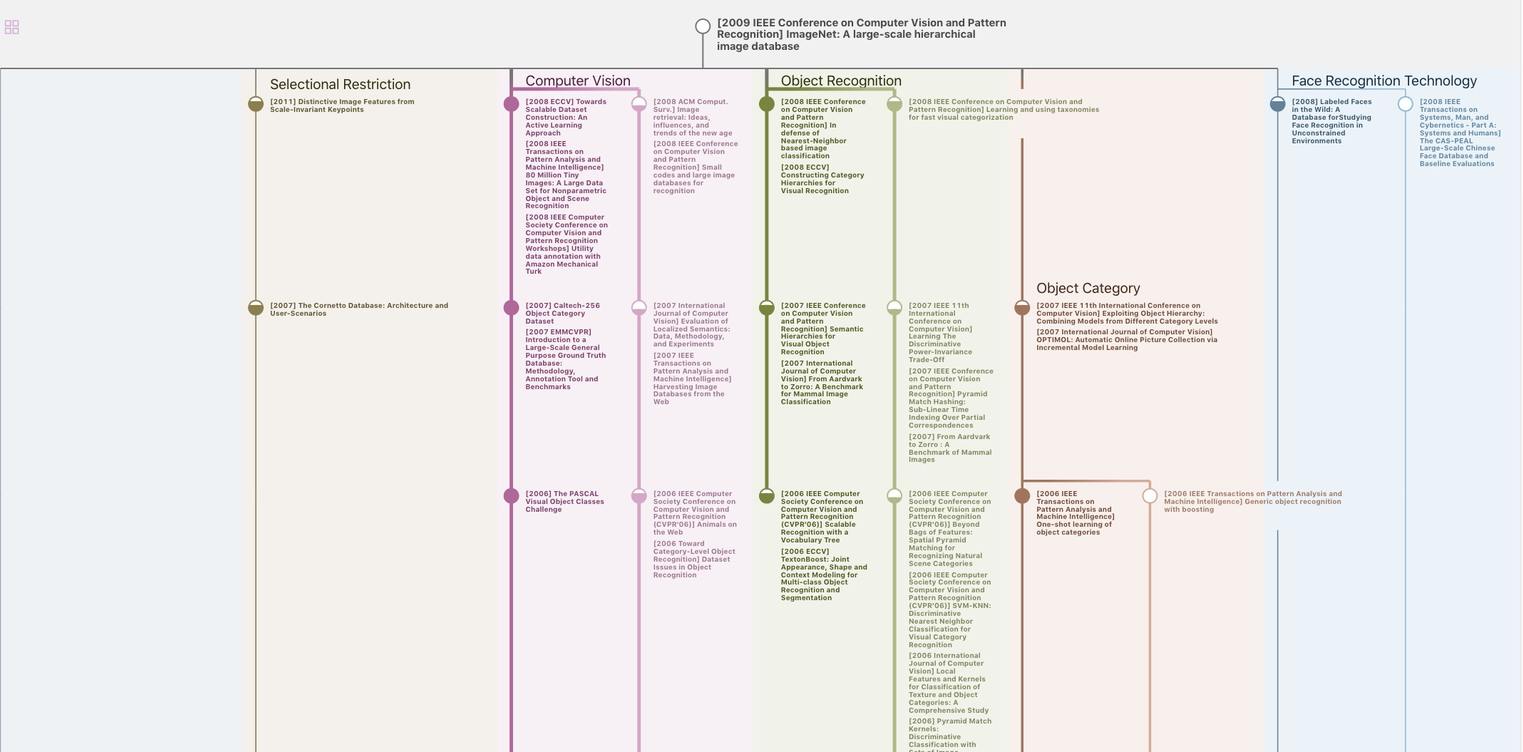
生成溯源树,研究论文发展脉络
Chat Paper
正在生成论文摘要