Development of a Nonlinear Model Predictive Control for Stabilization of a Gas-Lift Oil Well
INDUSTRIAL & ENGINEERING CHEMISTRY RESEARCH(2022)
摘要
A gas-lift oil well is an oil well that uses high-pressure natural gas to improve oil production. This type of oil well is unstable if not enough gas is available for operation, when a phenomenon called slugging may occur. Besides slugging, other relevant challenges of the problem are unknown internal state and well parameters and unmeasurable disturbances. In this work, a nonlinear model predictive controller (NMPC) for this type of oil well was developed. To build this NMPC, a parameter estimation was performed with data gathered by a four-factor design of experiments. Using the internal states generated during the parameter estimation, a machine learning algorithm was trained to infer the internal state from sensor data. The NMPC was tested against slugging, set point changes, and unmeasurable disturbances and compared with results from both a perfect NMPC and an NMPC with states estimated by extended Kalman filter. Estimation of the internal states by the neural network was accurate enough to allow for proper control. The NMPC actions were aggressive but successfully curbed slugging and kept the controlled variables around their set points. The main contributions of this work are developing a methodology for state inference supported by neural networks and presenting a pair of nonlinear models for model mismatch studies.
更多查看译文
AI 理解论文
溯源树
样例
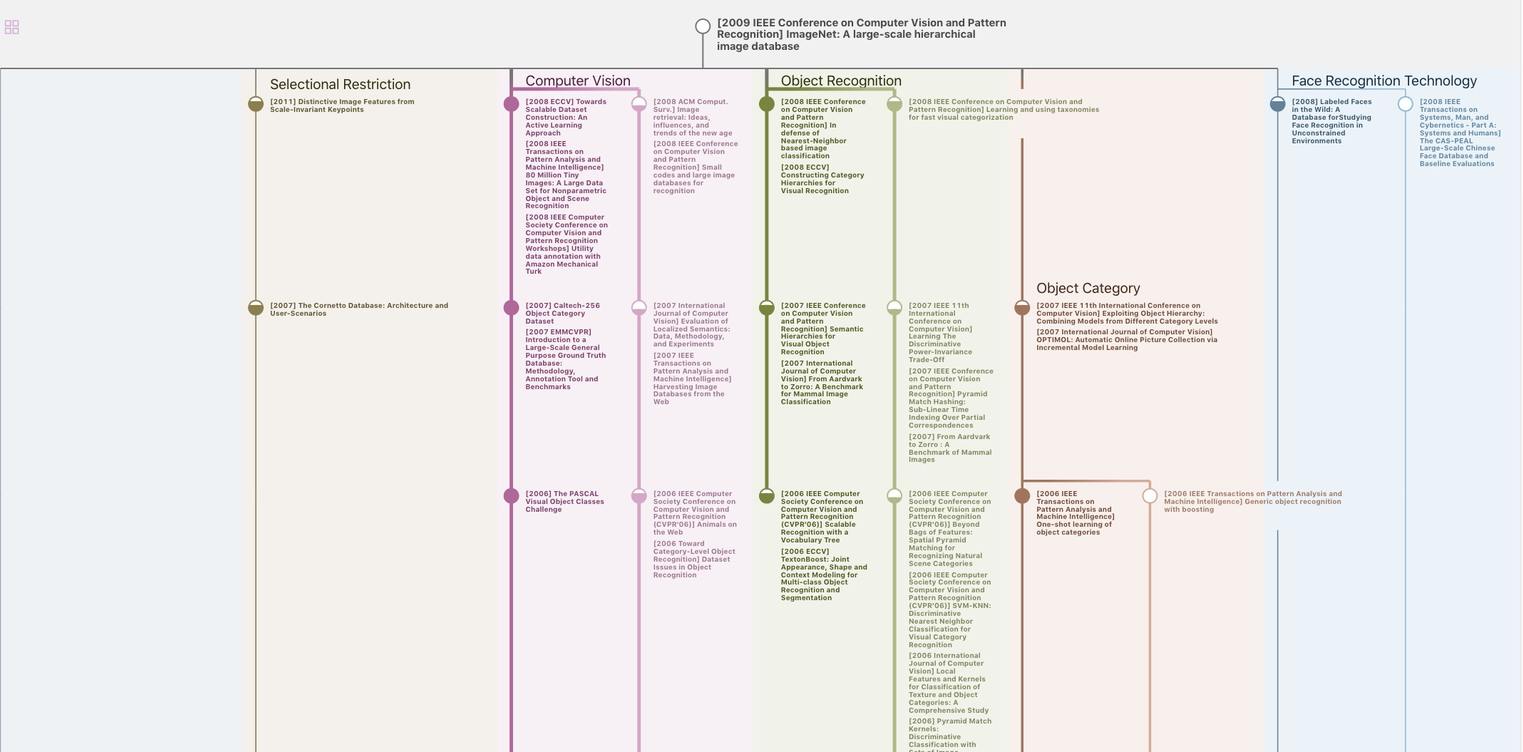
生成溯源树,研究论文发展脉络
Chat Paper
正在生成论文摘要