Interpreting Deep Learning Models for Knowledge Tracing
INTERNATIONAL JOURNAL OF ARTIFICIAL INTELLIGENCE IN EDUCATION(2022)
摘要
As a prominent aspect of modeling learners in the education domain, knowledge tracing attempts to model learner’s cognitive process, and it has been studied for nearly 30 years. Driven by the rapid advancements in deep learning techniques, deep neural networks have been recently adopted for knowledge tracing and have exhibited unique advantages and capabilities. Due to the complex multilayer structure of deep neural networks and their ”black box” operations, these deep learning based knowledge tracing (DLKT) models also suffer from non-transparent decision processes. The lack of interpretability has painfully impeded DLKT models’ practical applications, as they require the user to trust in the model’s output. To tackle such a critical issue for today’s DLKT models, we present an interpreting method by leveraging explainable artificial intelligence (xAI) techniques. Specifically, the interpreting method focuses on understanding the DLKT model’s predictions from the perspective of its sequential inputs. We conduct comprehensive evaluations to validate the feasibility and effectiveness of the proposed interpreting method at the skill-answer pair level. Moreover, the interpreting results also capture the skill-level semantic information, including the skill-specific difference, distance and inner relationships. This work is a solid step towards fully explainable and practical knowledge tracing models for intelligent education.
更多查看译文
关键词
Artificial intelligence in education,Intelligent tutoring system,Educational data mining,Intelligent agent,Interpretability of deep learning
AI 理解论文
溯源树
样例
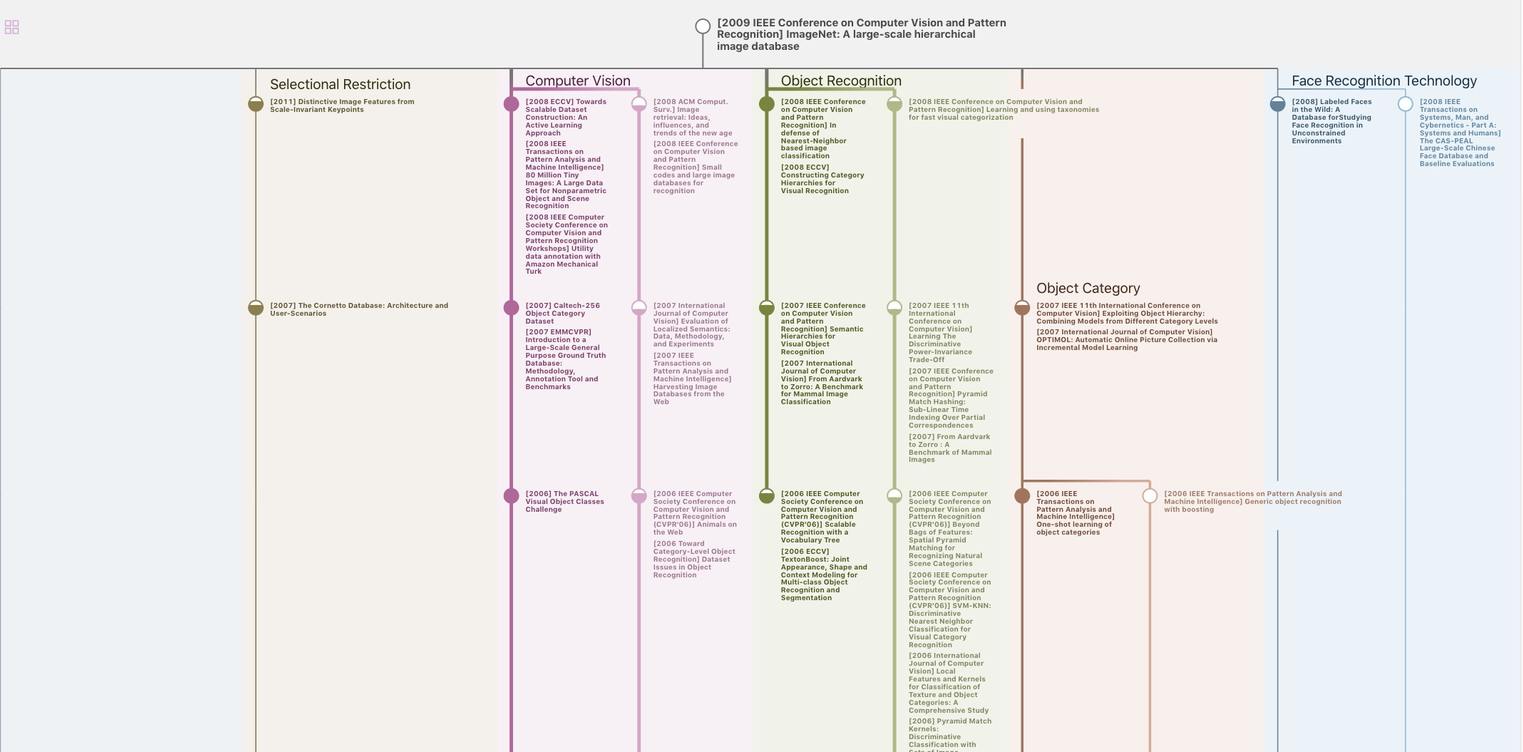
生成溯源树,研究论文发展脉络
Chat Paper
正在生成论文摘要