An Improved Multi-Label Learning Method with ELM-RBF and a Synergistic Adaptive Genetic Algorithm
ALGORITHMS(2022)
摘要
Profiting from the great progress of information technology, a huge number of multi-label samples are available in our daily life. As a result, multi-label classification has aroused widespread concern. Different from traditional machine learning methods which are time-consuming during the training phase, ELM-RBF (extreme learning machine-radial basis function) is more efficient and has become a research hotspot in multi-label classification. However, because of the lack of effective optimization methods, conventional extreme learning machines are always unstable and tend to fall into local optimum, which leads to low prediction accuracy in practical applications. To this end, a modified ELM-RBF with a synergistic adaptive genetic algorithm (ELM-RBF-SAGA) is proposed in this paper. In ELM-RBF-SAGA, we present a synergistic adaptive genetic algorithm (SAGA) to optimize the performance of ELM-RBF. In addition, two optimization methods are employed collaboratively in SAGA. One is used for adjusting the range of fitness value, the other is applied to update crossover and mutation probability. Sufficient experiments show that ELM-RBF-SAGA has excellent performance in multi-label classification.
更多查看译文
关键词
ELM-RBF, adaptive genetic algorithm, multi-label classification, optimization
AI 理解论文
溯源树
样例
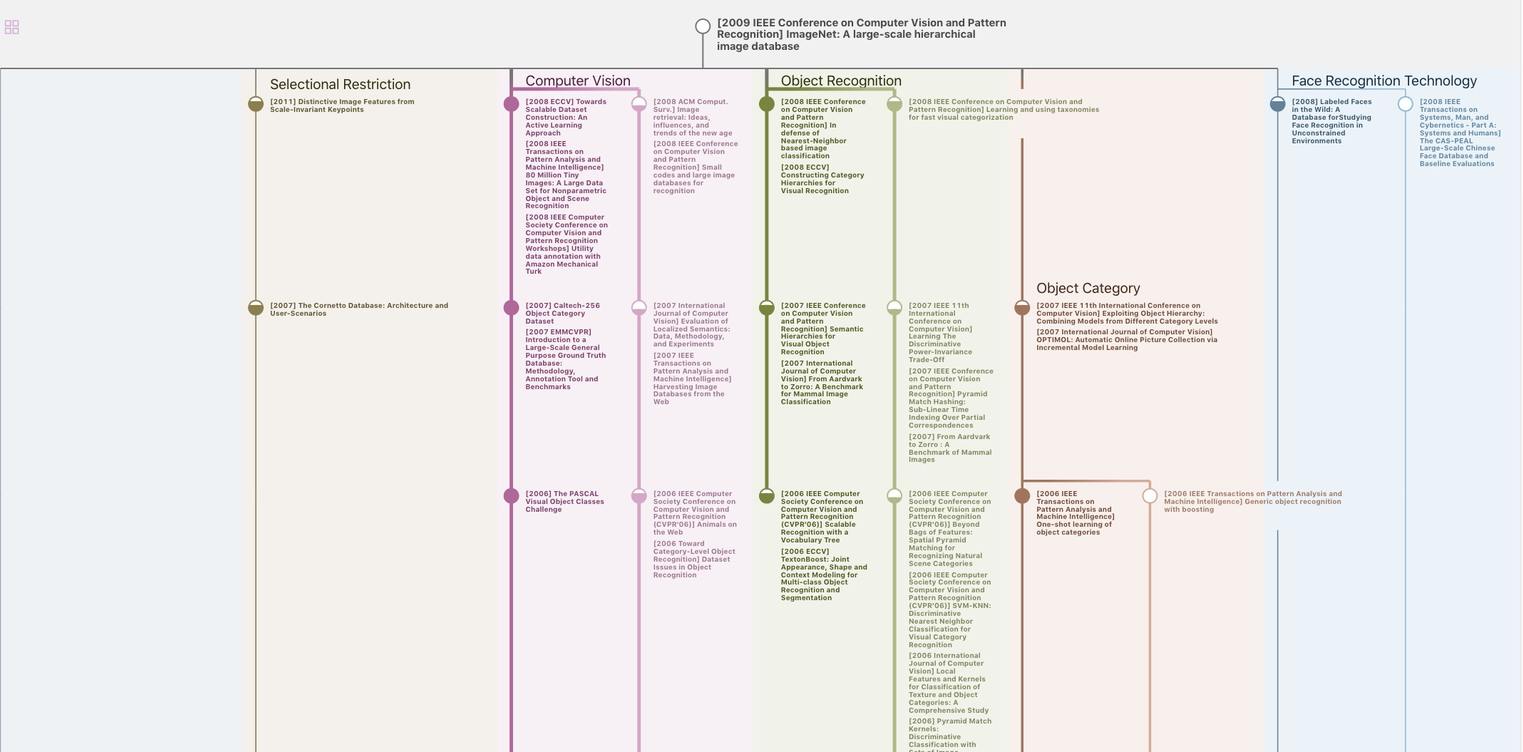
生成溯源树,研究论文发展脉络
Chat Paper
正在生成论文摘要