Robust Probit Linear Mixed Models for Longitudinal Binary Data
Biometrical journal(2022)
摘要
In this paper, we propose Bayesian analysis methods dealing with longitudinal data involving repeated binary outcomes on subjects with dropouts. The proposed Bayesian methods implement probit models with random effects to capture heterogeneity and hypersphere decomposition to model the correlation matrix for serial correlation of repeated responses. We investigate the model robustness against misspecifications of the probit models along with techniques to handle missing data. The parameters of the proposed models are estimated by implementing an Markov chain Monte Carlo (MCMC) algorithm, and simulations were performed to provide a comparison with other models and validate the choice of prior distributions. The simulations show that when suitable correlation structures are specified, the proposed approach improves estimation of the regression parameters in terms of the mean percent relative error and the mean squared error. Finally, two real data examples are provided to illustrate the proposed approach.
更多查看译文
关键词
correlation matrix,hypersphere decomposition,MCMC,R package
AI 理解论文
溯源树
样例
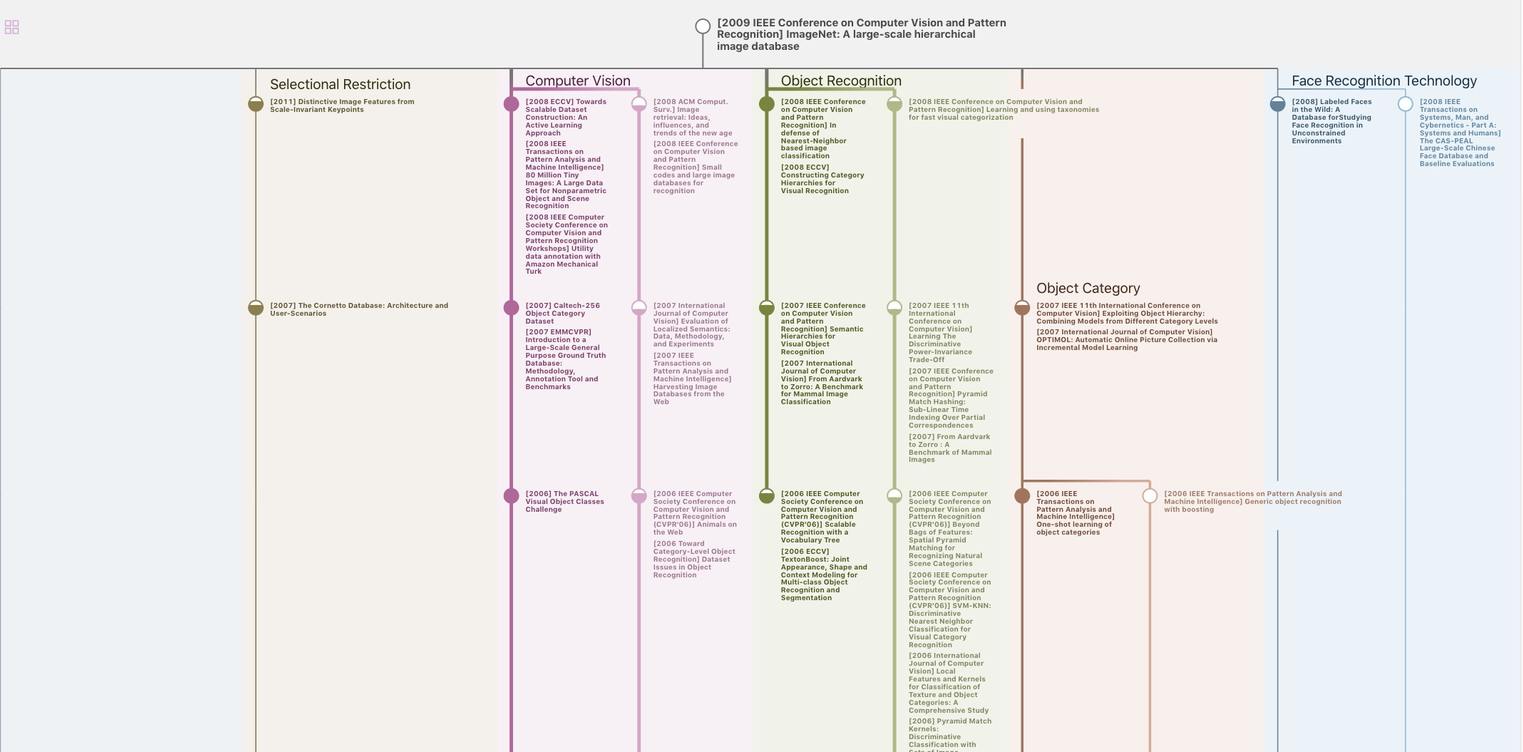
生成溯源树,研究论文发展脉络
Chat Paper
正在生成论文摘要