Data-driven reliable prediction of production indicators in the blast furnace using TS fuzzy neural network based on bat algorithm
JOURNAL OF EXPERIMENTAL & THEORETICAL ARTIFICIAL INTELLIGENCE(2024)
摘要
Operational optimisation of the blast furnace (BF) is significant in facilitating smooth operation and reducing the production cost. Operation indicators are pivotal parameters used to measure operating status and reflect the quality of molten iron of the BF. In this study, a data-driven method for the prediction of production indicators is proposed by a novel fuzzy neural network of Takagi-Sugeno with bat algorithm (BA-TS-FNN). In practice, a BF usually works under varying operating conditions due to internal or external factors, redundant features with high dimensionally lead to poor computation time and prediction performance. To solve this problem, mutual information is applied to enhance understanding of the data. Considering that the standard TS-FNN cannot commendably cope with rapid convergence, local optimal, and sensitivity to initial weight problems during learning the prediction model, a bat optimisation algorithm is proposed to search global optimal parameters and improve the convergence rate. Finally, the probability density function (PDF) of modelling error is estimated by the kernel density estimation (KDE) as a criterion to measure the performance of the method. Experiments with industrial data from BF have demonstrated that the proposed method produces higher estimating accuracy than other modelling methods.
更多查看译文
关键词
Data-driven model,production indicators,TS fuzzy neural network (TS-FNN),bat algorithm,blast furnace (BF)
AI 理解论文
溯源树
样例
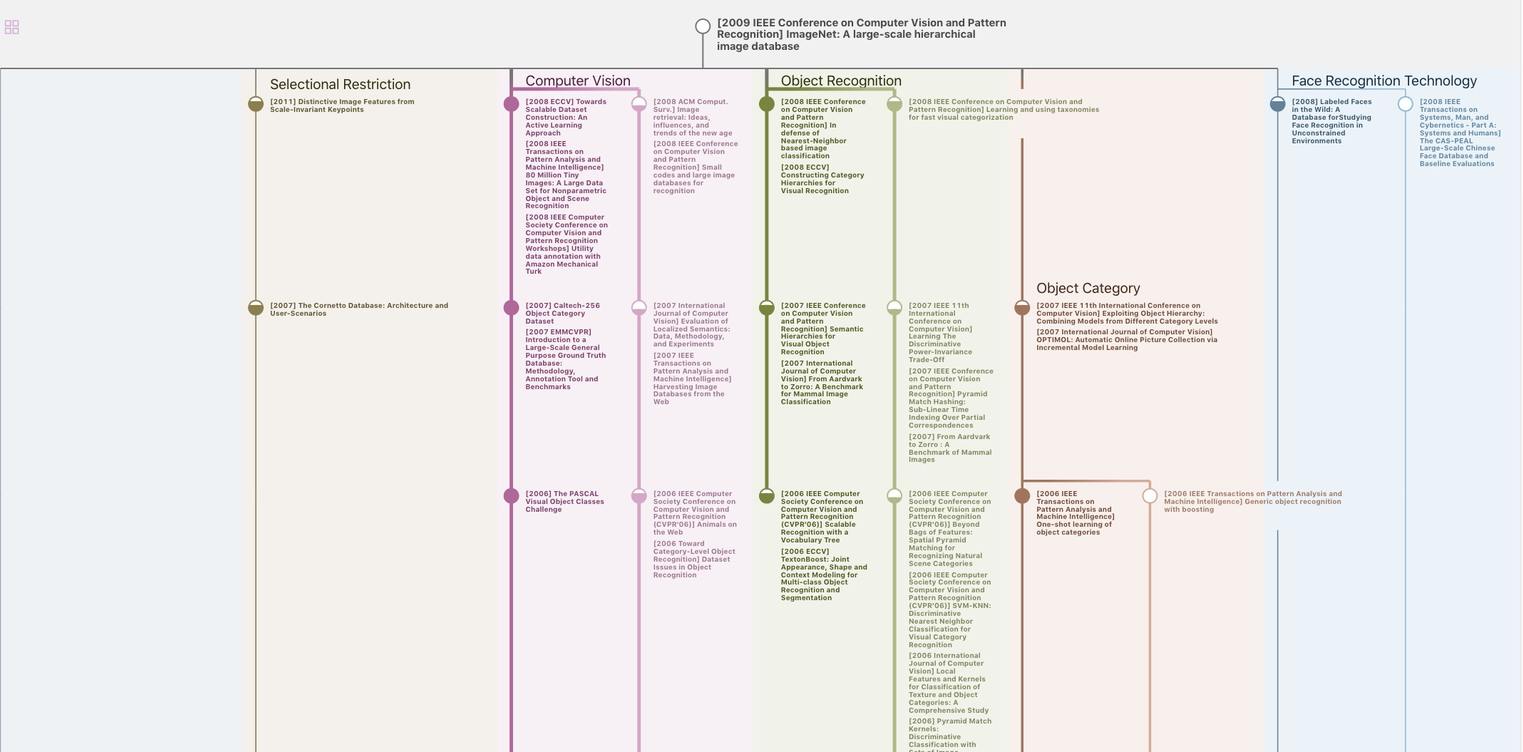
生成溯源树,研究论文发展脉络
Chat Paper
正在生成论文摘要