CDAML: a cluster-based domain adaptive meta-learning model for cross domain recommendation
WORLD WIDE WEB-INTERNET AND WEB INFORMATION SYSTEMS(2022)
摘要
Recommender systems play an important role in providing users required information in a timely and effective manner. However, the cold-start problem of limited historical records of a new user in target domain makes it difficult to model user’s comprehensive preferences. This severely affects the accuracy of recommendation. Although some meta-learning based approaches have alleviated the cold-start problem by learning well-generalized initial parameters for each user, they neglect user’s information in source domains, and seem to be weak in providing each user suitable initial parameters separately. To tackle these challenges, we propose a novel cluster-based domain adaptive meta-learning model for cross-domain recommendation (CDAML). Specially, we utilize the adversarial cross-domain methods to introduce domain adaptation into the meta-learning framework, which can transfer domain-independent user preferences (i.e. intrinsic preferences) from source domains for improved recommendation in target domain via adversarial learning. Besides, we further design a soft-clustering based method to guide the globally shared parameter initialization in a finer granularity of cluster level, which not only contribute to avoid local optima, but also better transfer the shared knowledge among users with similar cross-domain preferences. Finally, comprehensive experiments are conducted on three real-world datasets to demonstrate the superior performance of CDAML compared with state-of-the-art recommendation methods.
更多查看译文
关键词
Recommendation,Meta-learning,Cross-domain,Adversarial mechanism
AI 理解论文
溯源树
样例
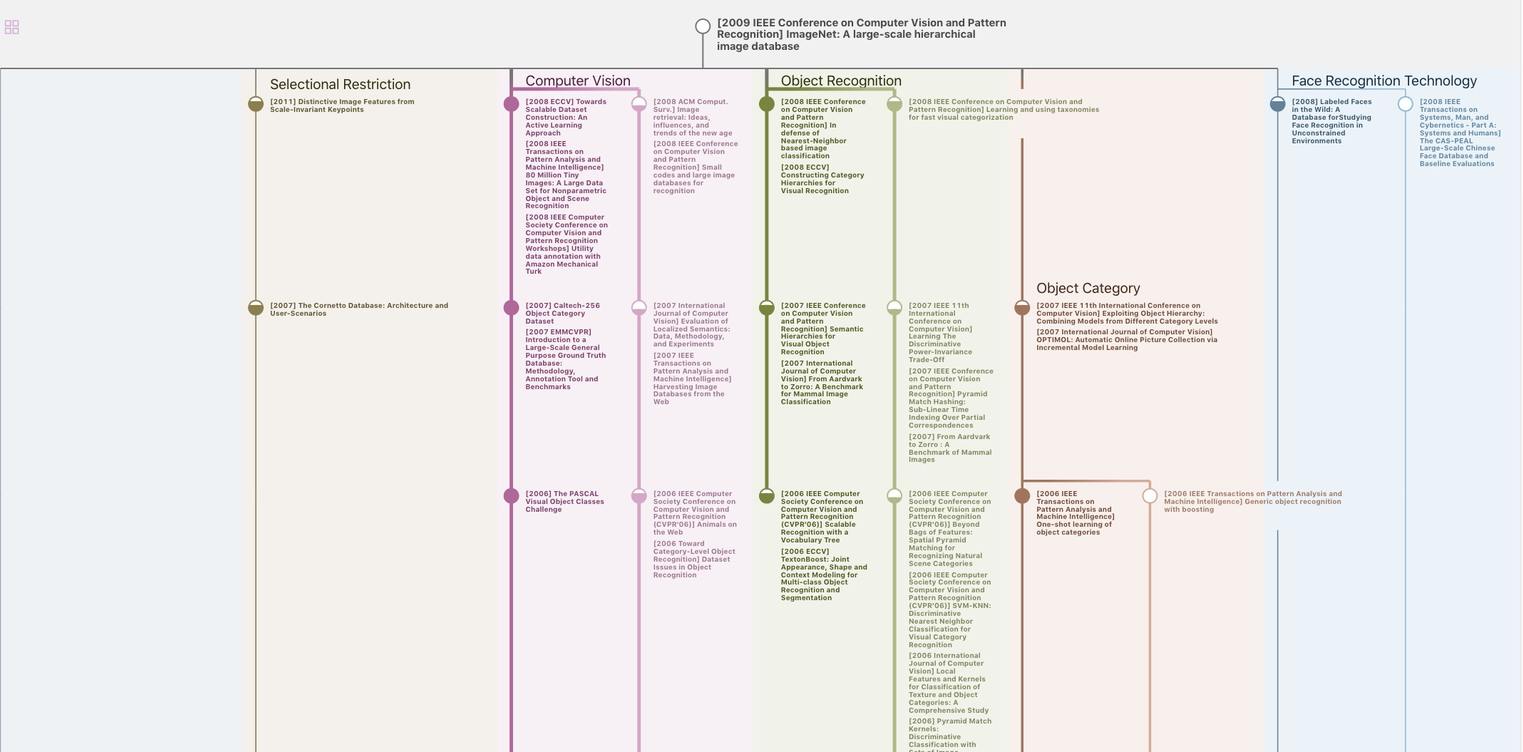
生成溯源树,研究论文发展脉络
Chat Paper
正在生成论文摘要