Precision radiotherapy by SPECT lung functional imaging in NSCLC
JOURNAL OF MENS HEALTH(2022)
摘要
Background: Single Photon Emission Computed Tomography (SPECT) could be used to avoid the non-affected perfusion areas in patients with non-small-cell lung cancer (NSCLC) and to potentially reduce lung toxicity. The aim of this study is to compare dosimetric differences between two different 3D-conformal treatment plans, with and without CT/SPECT contribution. Methods: Simulation Computed tomography (CT) scans were accurately co-registered with SPECT scans and three different areas, based on SPECT intensity perfusion, were contoured: low perfusion (LP), medium perfusion (MP) and high perfusion (HP). Two different 3D-conformal plans, with co-planar and nonco-planar fields, were generated; one without SPECT information (anatomic plan), and one using the perfusion area identified with functional imaging (functional plan). Results: 9 patients were planned and a total of 18 plans were available for analysis. Anatomical and functional plans resulted in comparable planning target volume (PTV) coverage. In the functional plans, a significant reduction of dose in high perfusion areas was reported. The reduction of HP-V20 Gy values ranged from 15% to 8% (p = 0.046), the ipsiTIP-V20 Gy from 38% to 22% (p = 0.028) and ipsiIIP-Dmean reduction from 16 Gy to 12 Gy (p = 0.039). No significant differences in other organs at risk (OARS) metrics were reported between anatomical and functional plans. Conclusions: Despite the few cases reported, the strength of our study lies in the reported benefit of functional lung information in 3D conformal radiation planning, without compromising target coverage or worsening dose distribution to the OARs. There is an urgent need for prospective clinical and randomized trials in order to define the role of Tung functional imaging in reducing toxicity in clinical practice.
更多查看译文
关键词
pecision medicine, functional imaging, NSCLC, radiotherapy
AI 理解论文
溯源树
样例
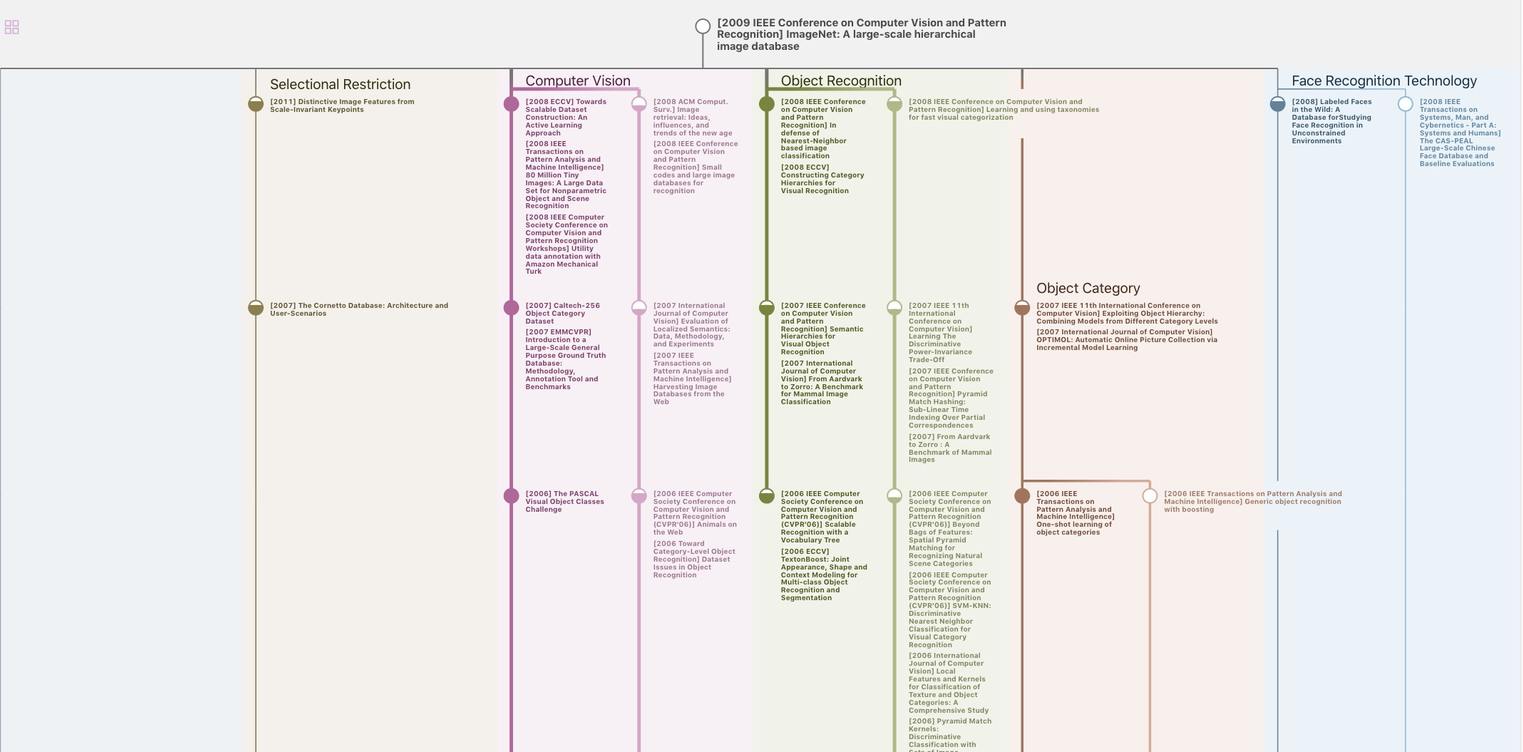
生成溯源树,研究论文发展脉络
Chat Paper
正在生成论文摘要