Deep Learning-Based Denoising in Brain Tumor CHO PET: Comparison with Traditional Approaches
APPLIED SCIENCES-BASEL(2022)
摘要
F-1(8)-choline (CHO) PET image remains noisy despite minimum physiological activity in the normal brain, and this study developed a deep learning-based denoising algorithm for brain tumor CHO PET. Thirty-nine presurgical CHO PET/CT data were retrospectively collected for patients with pathological confirmed primary diffuse glioma. Two conventional denoising methods, namely, block-matching and 3D filtering (BM3D) and non-local means (NLM), and two deep learning-based approaches, namely, Noise2Noise (N2N) and Noise2Void (N2V), were established for imaging denoising, and the methods were developed without paired data. All algorithms improved the image quality to a certain extent, with the N2N demonstrating the best contrast-to-noise ratio (CNR) (4.05 +/- 3.45), CNR improvement ratio (13.60% +/- 2.05%) and the lowest entropy (1.68 +/- 0.17), compared with other approaches. Little changes were identified in traditional tumor PET features including maximum standard uptake value (SUVmax), SUVmean and total lesion activity (TLA), while the tumor-to-normal (T/N ratio) increased thanks to smaller noise. These results suggested that the N2N algorithm can acquire sufficient denoising performance while preserving the original features of tumors, and may be generalized for abundant brain tumor PET images.
更多查看译文
关键词
CHO, PET, denoising, deep learning, evaluation
AI 理解论文
溯源树
样例
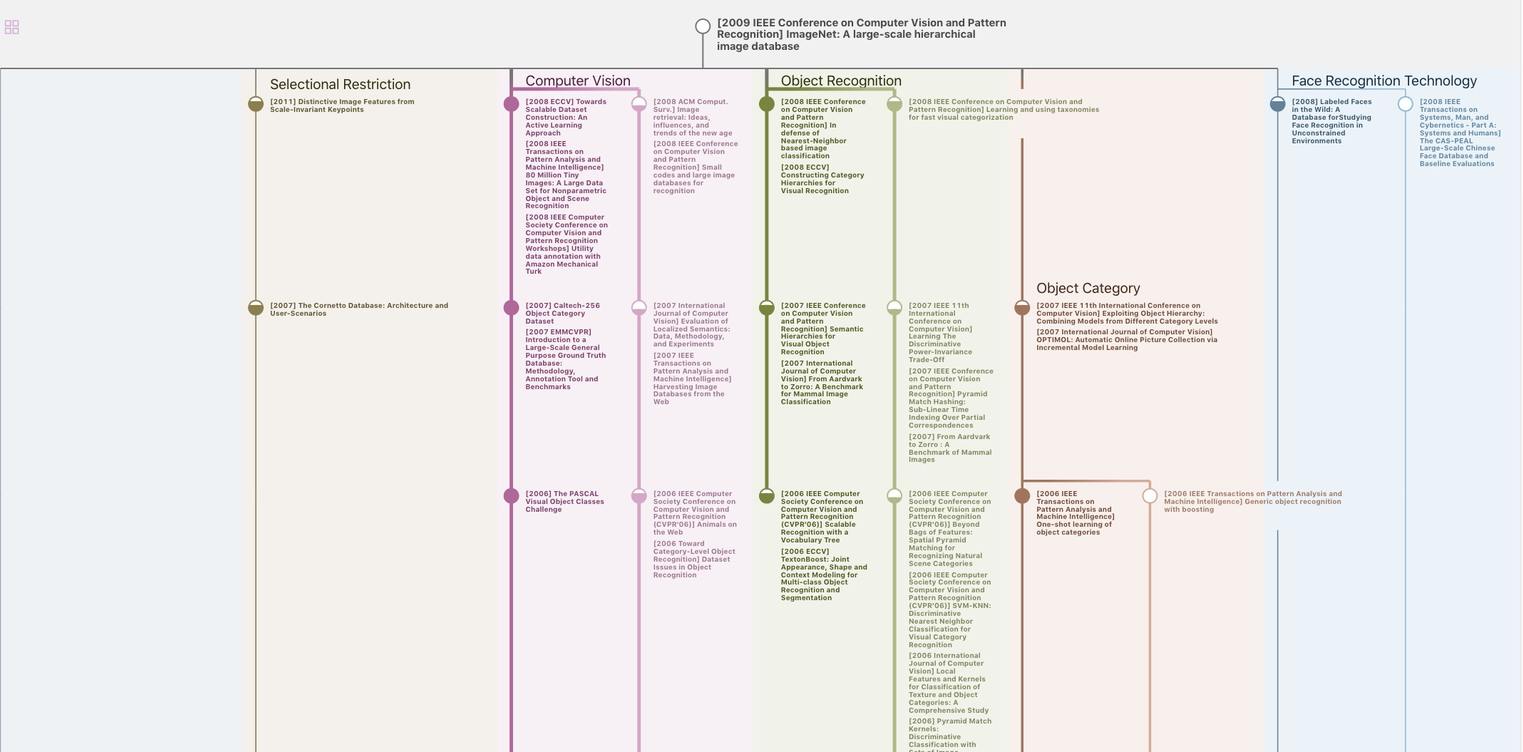
生成溯源树,研究论文发展脉络
Chat Paper
正在生成论文摘要