Dual Auto-Encoder GAN-Based Anomaly Detection for Industrial Control System
APPLIED SCIENCES-BASEL(2022)
摘要
As a core tool, anomaly detection based on a generative adversarial network (GAN) is showing its powerful potential in protecting the safe and stable operation of industrial control systems (ICS) under the Internet of Things (IoT). However, due to the long-tailed distribution of operating data in ICS, existing GAN-based anomaly detection models are prone to misjudging an unseen marginal sample as an outlier. Moreover, it is difficult to collect abnormal samples from ICS. To solve these challenges, a dual auto-encoder GAN-based anomaly detection model is proposed for the industrial control system, simply called the DAGAN model, to achieve an accurate and efficient anomaly detection without any abnormal sample. First, an "encoder-decoder-encoder" architecture is used to build a dual GAN model for learning the latent data distribution without any anomalous sample. Then, a parameter-free dynamic strategy is proposed to robustly and accurately learn the marginal distribution of the training data through dynamic interaction between two GANs. Finally, based on the learned normal distribution and marginal distribution, an optimized anomaly score is used to measure whether a sample is an outlier, thereby reducing the probability of a marginal sample being misjudged. Extensive experiments on multiple datasets demonstrate the advantages of our DAGAN model.
更多查看译文
关键词
anomaly detection, dual GAN, auto-encoder, industrial control system
AI 理解论文
溯源树
样例
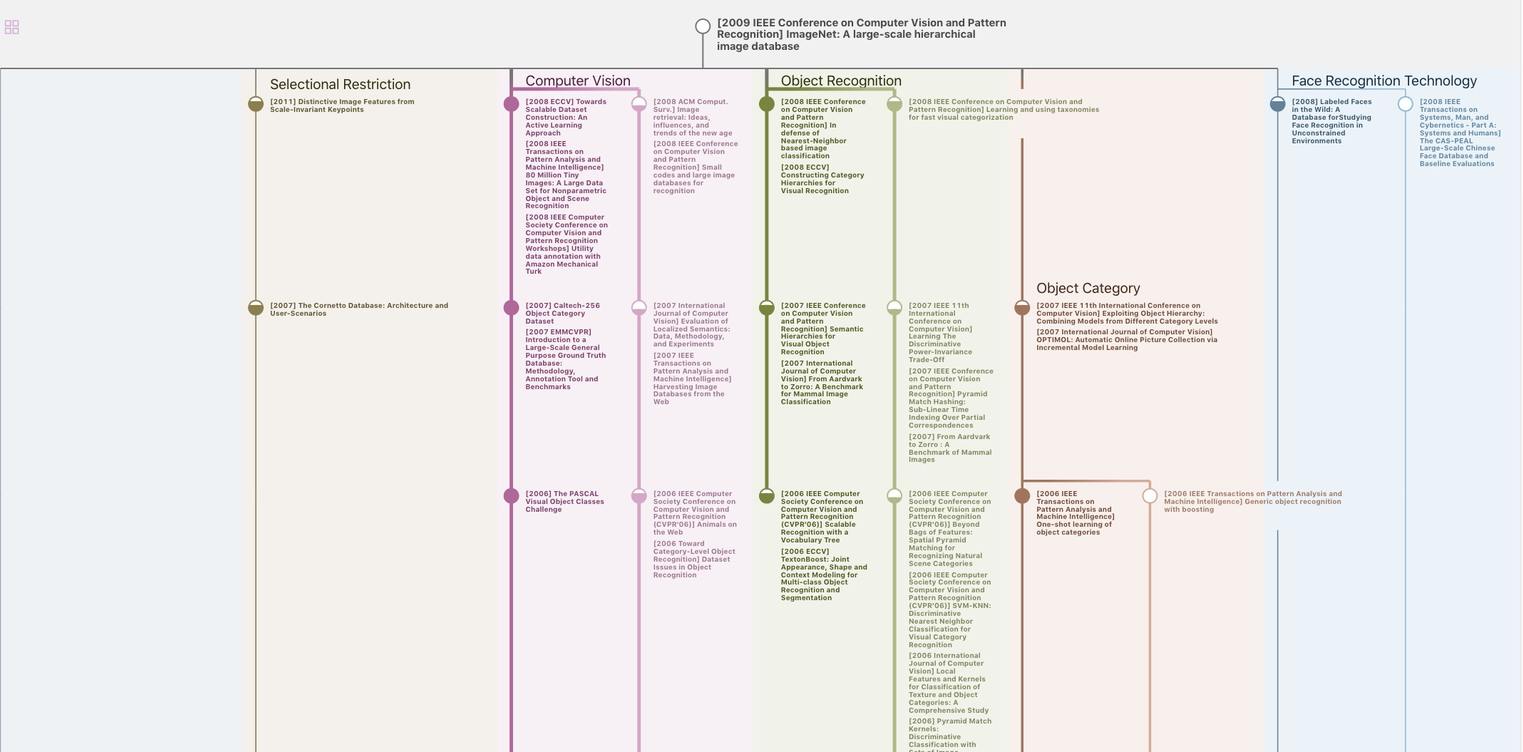
生成溯源树,研究论文发展脉络
Chat Paper
正在生成论文摘要