Exploring Loss Functions for Optimising the Accuracy of Siamese Neural Networks in Re-Identification Applications
FOURTEENTH INTERNATIONAL CONFERENCE ON MACHINE VISION (ICMV 2021)(2022)
摘要
As Re-Identification (Re-ID) is becoming more and more common in today's world, the need for more optimized algorithms also becomes more wanted. This is due to the importance of high accuracy as the consequences of an incorrect match can mean security issues, if used to gain access or result in incorrect findings in science due to wrong data. This paper explores enhancing the performance of Siamese Neural Networks by exploring the performance of loss functions to better suit the user's Re-IDing needs. These loss functions are Triplet loss, Triplet Hard loss and Quadruplet loss. Results show that the Triplet hard loss function performs better than the two others. The functions were tested on a human dataset as well as on animal datasets.
更多查看译文
关键词
Re-Identification, Siamese Neural Networks, Few Shot Learning, Triplet Loss, Metric Learning
AI 理解论文
溯源树
样例
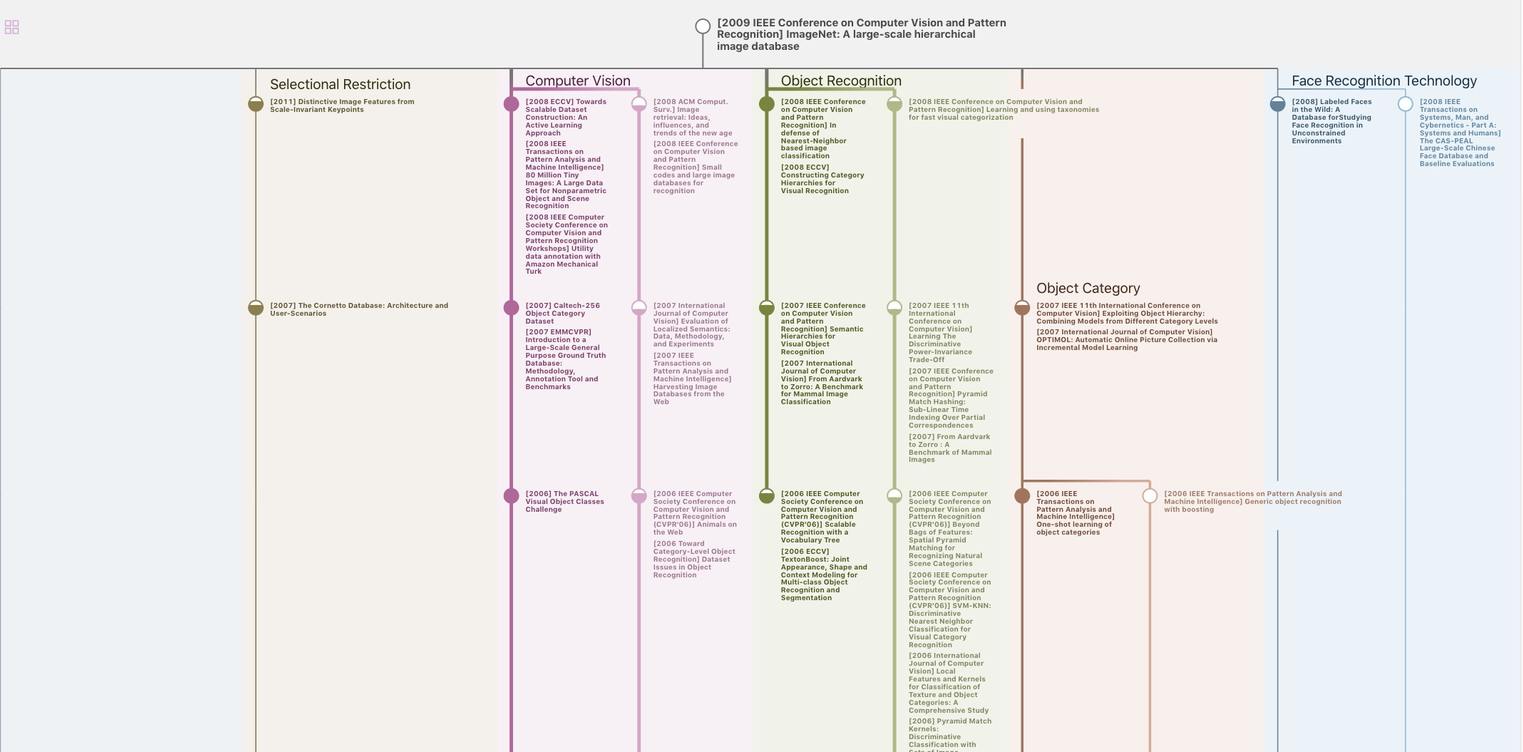
生成溯源树,研究论文发展脉络
Chat Paper
正在生成论文摘要