Point-to-Point Navigation of a Fish-Like Swimmer in a Vortical Flow With Deep Reinforcement Learning
FRONTIERS IN PHYSICS(2022)
摘要
Efficient navigation in complex flows is of crucial importance for robotic applications. This work presents a numerical study of the point-to-point navigation of a fish-like swimmer in a time-varying vortical flow with a hybrid method of deep reinforcement learning (DRL) and immersed boundary-lattice Boltzmann method (IB-LBM). The vortical flow is generated by placing four stationary cylinders in a uniform flow. The swimmer is trained to discover effective navigation strategies that could help itself to reach a given destination point in the flow field, utilizing only the time-sequential information of position, orientation, velocity and angular velocity. After training, the fish can reach its destination from random positions and orientations, demonstrating the effectiveness and robustness of the method. A detailed analysis shows that the fish utilizes highly subtle tail flapping to control its swimming orientation and take advantage of the reduced streamwise flow area to reach it destination, and in the same time avoiding entering the high flow velocity area.
更多查看译文
关键词
vortical flow, immersed boundary-lattice Boltzmann method, deep reinforcement learning, point-to-point navigation, robotic fish, target-directed swimming, fish swimming
AI 理解论文
溯源树
样例
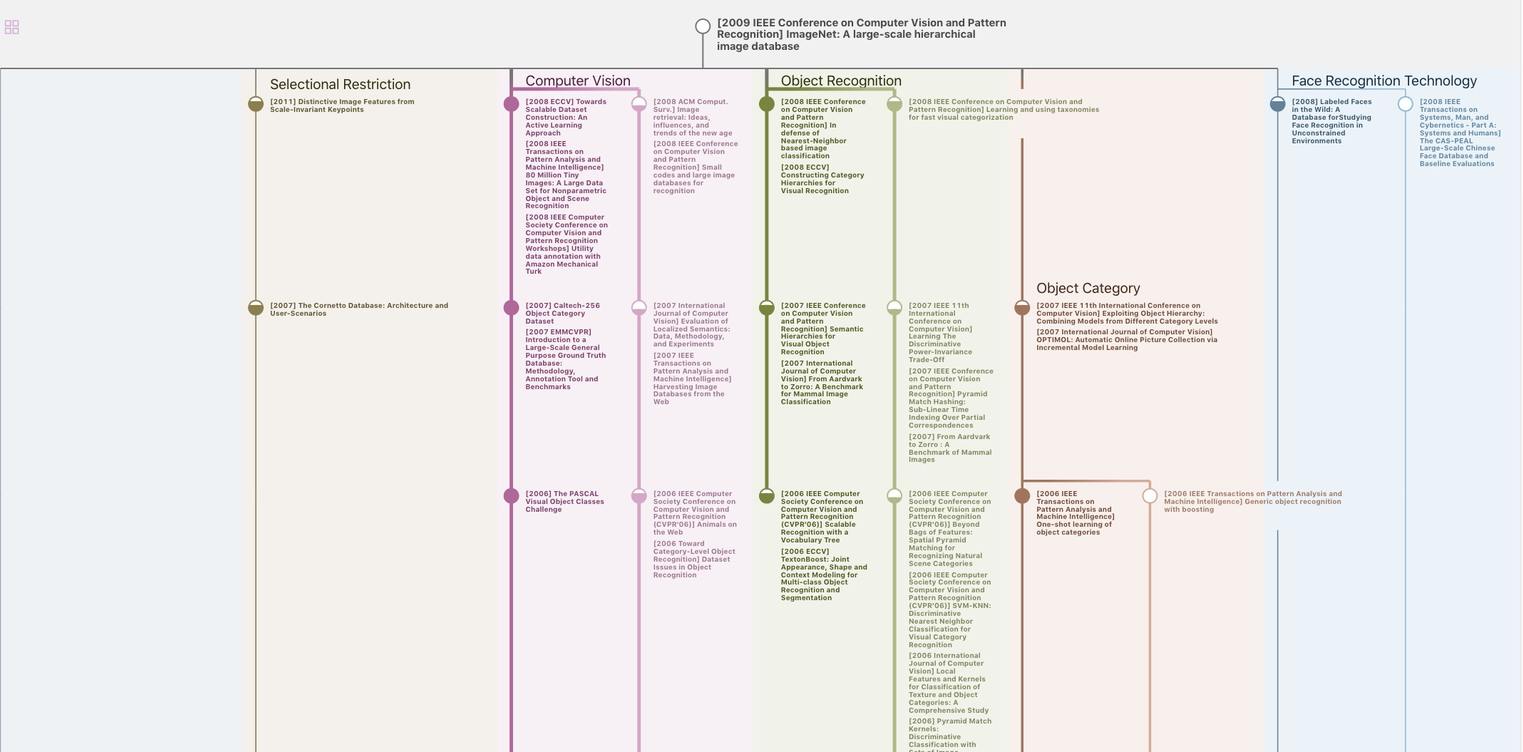
生成溯源树,研究论文发展脉络
Chat Paper
正在生成论文摘要