ANN Modelling of Surface Roughness of FDM Parts Considering the Effect of Hidden Layers, Neurons, and Process Parameters
ADVANCES IN MATERIALS AND PROCESSING TECHNOLOGIES(2024)
摘要
Hidden layers perform a vital role in the performance of artificial neural networks (ANNs), especially for serpentine problems where the curtailment of accuracy and time complexity is observed. A higher number of hidden layers and neurons enlarge the order of weights and influence the ANN accuracy. In the present work, an ANN model is developed to predict the surface roughness of fused deposition modelling (FDM) parts considering the effect of the number of neurons, hidden layers, layer height, infill density, nozzle temperature, and print speed. A feedforward back-propagation machine learning algorithm is used to model an ANN. This study finds better prediction accuracy with the ANN architecture having two hidden layers with 150 neurons on each layer (R-squared: 0.875) followed by one hidden layer with 250 neurons (R-squared: 0.83). This study finds a decrease in the surface roughness of FDM parts with the increase in infill density. However, the surface roughness was observed to increase with the layer height, print speed, and nozzle temperature. This study finds a decrease in the surface roughness of FDM parts with the increase in infill density and an increase in the surface roughness with the layer height, print speed, and nozzle temperature.
更多查看译文
关键词
ANN,fused deposition modelling,surface roughness,process parameters
AI 理解论文
溯源树
样例
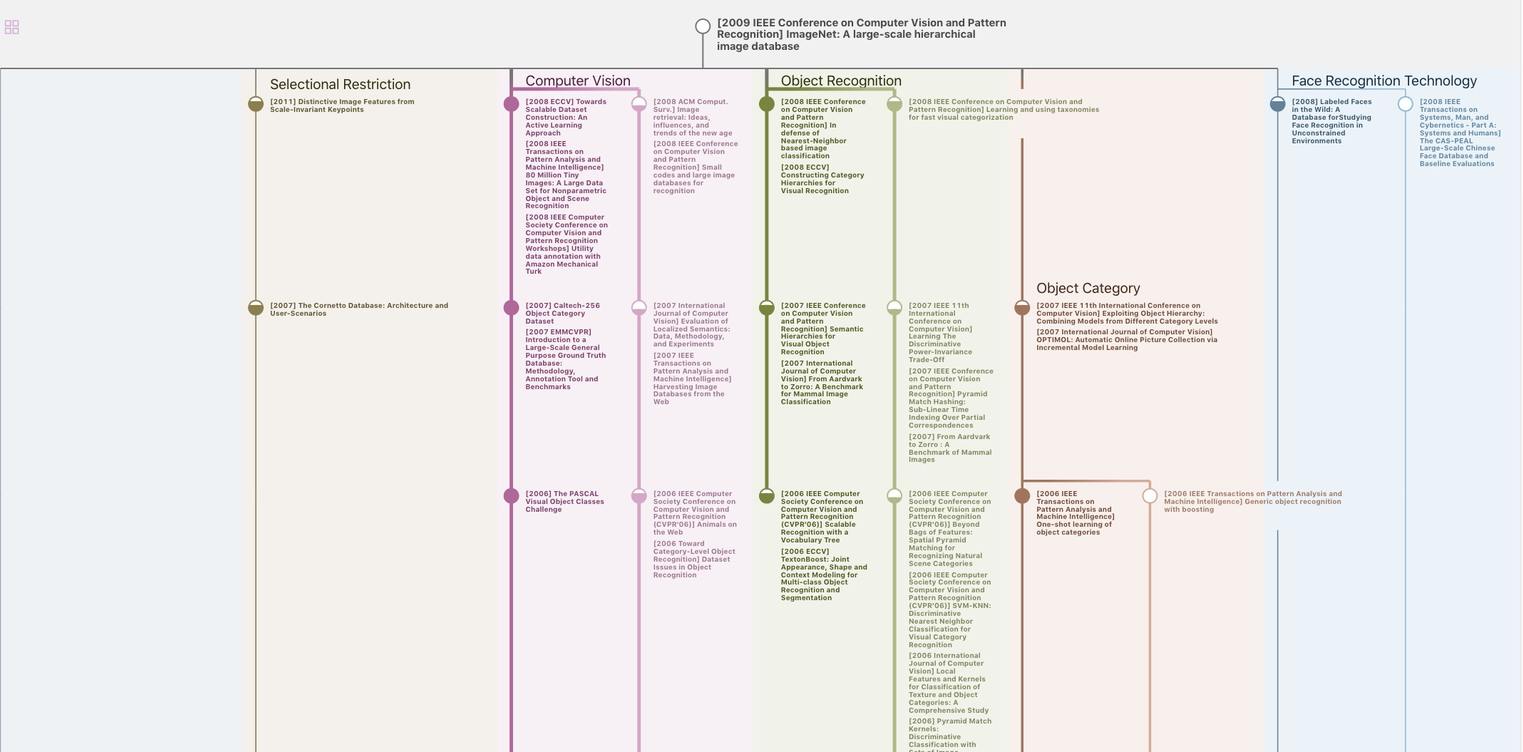
生成溯源树,研究论文发展脉络
Chat Paper
正在生成论文摘要