Flexural capacity assessment of precast deck joints based on deep forest
Structures(2022)
摘要
The bending performance of the precast deck system in accelerated bridge construction (ABC) is highly dependent on the flexural capacity of wet joints between decks, whereas precise predictive models are not yet available owing to its complexity and diversity. This paper thus proposed an efficient and accurate approach for the flexural capacity assessment using Deep Forest (DF) that integrates the benefits of deep learning and ensemble learning. The key variables were extracted from experimental observations and domain knowledge, and an extensive database of 391 deck-level bending tests was constructed. Then, the DF model was developed, which substitutes the neurons in deep neural networks (DNN) with random forests (RF) to construct a deep cascade structure. The performance of DF was shown to be superior to that of DNN, RF, and three popular tree-based models on all of the metrics employed. The comparison of DF with mechanics-based models including sectional analysis and struct-and-tie model further demonstrated its superiority. Finally, the decision-making mechanism of flexural capacity prediction was also discussed from global and local levels via impurity-based feature importance and local interpretable model-agnostic explanations method, allowing design recommendations to be made.
更多查看译文
关键词
Accelerated bridge construction (ABC),Precast deck joint,Flexural capacity,Deep Forest,Deep learning,Ensemble learning
AI 理解论文
溯源树
样例
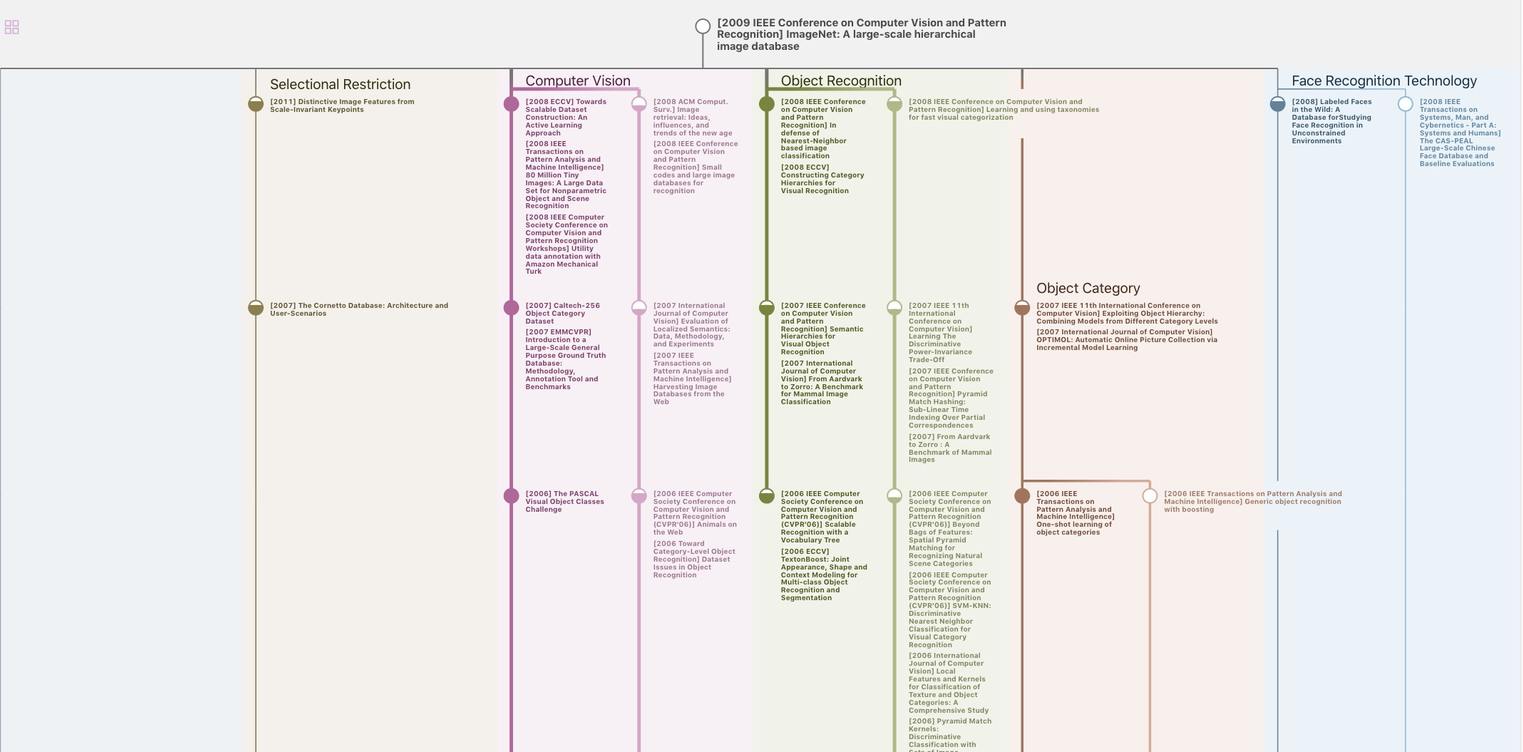
生成溯源树,研究论文发展脉络
Chat Paper
正在生成论文摘要