Improving Non-Autoregressive Machine Translation Using Sentence-Level Semantic Agreement
Applied sciences(2022)
摘要
Theinference stage can be accelerated significantly using a Non-Autoregressive Transformer (NAT). However, the training objective used in the NAT model also aims to minimize the loss between the generated words and the golden words in the reference. Since the dependencies between the target words are lacking, this training objective computed at word level can easily cause semantic inconsistency between the generated and source sentences. To alleviate this issue, we propose a new method, Sentence-Level Semantic Agreement (SLSA), to obtain consistency between the source and generated sentences. Specifically, we utilize contrastive learning to pull the sentence representations of the source and generated sentences closer together. In addition, to strengthen the capability of the encoder, we also integrate an agreement module into the encoder to obtain a better representation of the source sentence. The experiments are conducted on three translation datasets: the WMT 2014 EN → DE task, the WMT 2016 EN → RO task, and the IWSLT 2014 DE → DE task, and the improvement in the NAT model’s performance shows the effect of our proposed method.
更多查看译文
关键词
machine translation,non-autoregressive,contrastive learning,semantic agreement
AI 理解论文
溯源树
样例
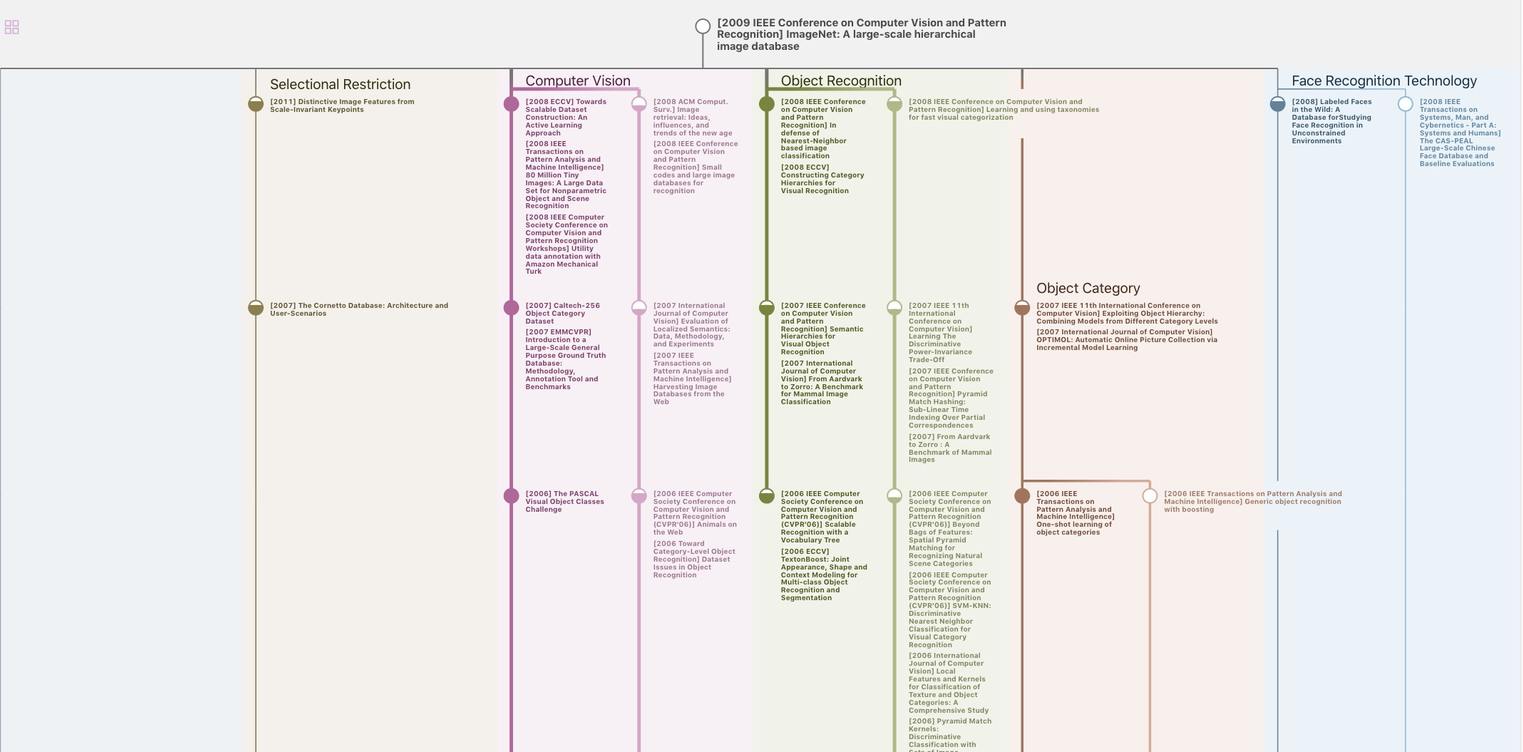
生成溯源树,研究论文发展脉络
Chat Paper
正在生成论文摘要