HAGDAVS: Height-Augmented Geo-Located Dataset for Detection and Semantic Segmentation of Vehicles in Drone Aerial Orthomosaics
DATA(2022)
摘要
Detection and Semantic Segmentation of vehicles in drone aerial orthomosaics has applications in a variety of fields such as security, traffic and parking management, urban planning, logistics, and transportation, among many others. This paper presents the HAGDAVS dataset fusing RGB spectral channel and Digital Surface Model DSM for the detection and segmentation of vehicles from aerial drone images, including three vehicle classes: cars, motorcycles, and ghosts (motorcycle or car). We supply DSM as an additional variable to be included in deep learning and computer vision models to increase its accuracy. RGB orthomosaic, RG-DSM fusion, and multi-label mask are provided in Tag Image File Format. Geo-located vehicle bounding boxes are provided in GeoJSON vector format. We also describes the acquisition of drone data, the derived products, and the workflow to produce the dataset. Researchers would benefit from using the proposed dataset to improve results in the case of vehicle occlusion, geo-location, and the need for cleaning ghost vehicles. As far as we know, this is the first openly available dataset for vehicle detection and segmentation, comprising RG-DSM drone data fusion and different color masks for motorcycles, cars, and ghosts. Dataset: https://doi.org/10.5281/zenodo.6323712. Dataset License: Licensed under Creative Commons Attribution 4.0 International.
更多查看译文
关键词
vehicle detection, semantic segmentation, orthomosaics, Geographic Information Systems (GIS)
AI 理解论文
溯源树
样例
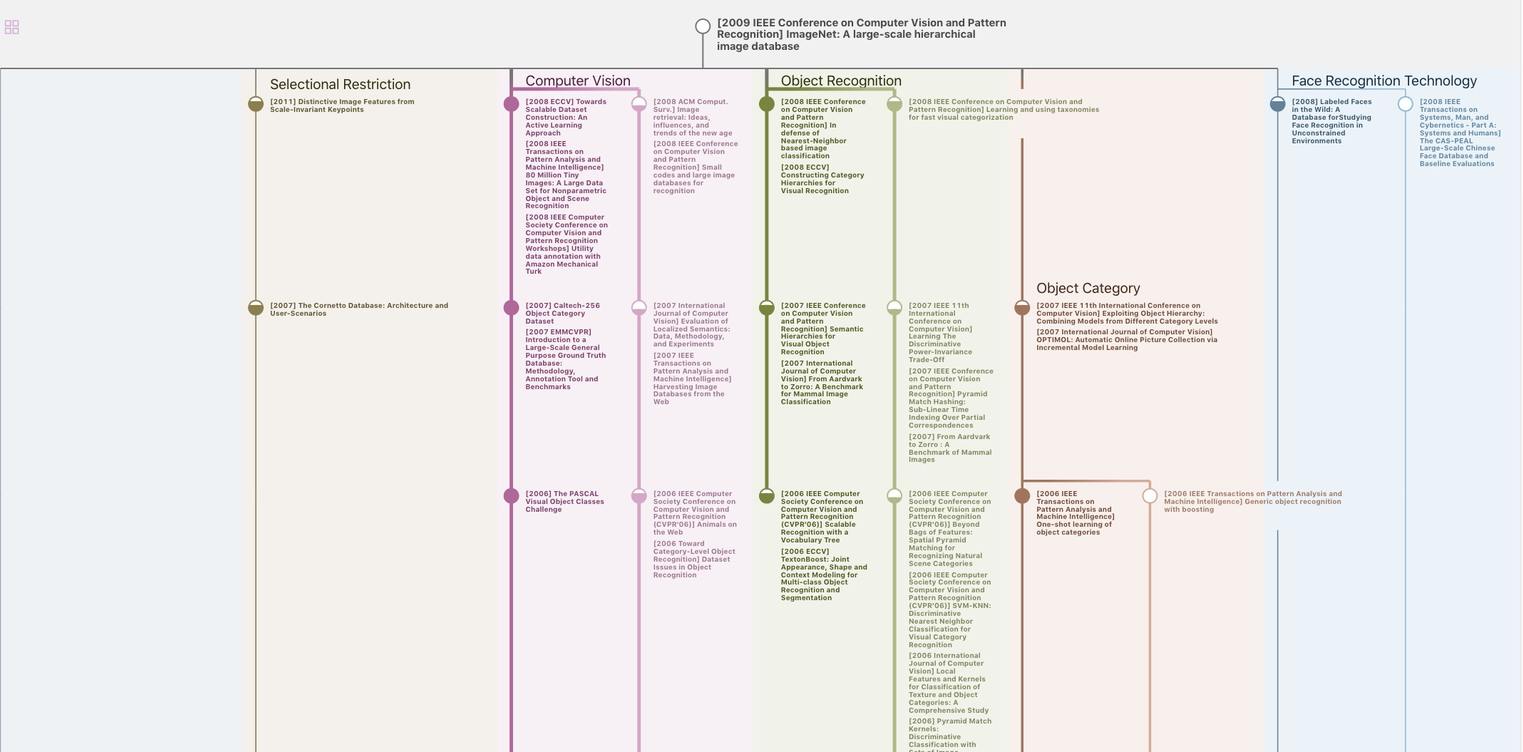
生成溯源树,研究论文发展脉络
Chat Paper
正在生成论文摘要