Combination of Visual and Semantic Criteria for Automated Selection of Region Proposals in a Bounding Box
FOURTEENTH INTERNATIONAL CONFERENCE ON MACHINE VISION (ICMV 2021)(2022)
摘要
Deep learning based techniques have been widely used for semantic segmentation. The underlying voluminous DNN models are trained on large datasets that have been annotated at the pixel level by humans. Such low-level annotation tasks are expensive to obtain for newly collected datasets. Alternatively, we propose ComViSe, a segmentation pipeline that requires only high-level annotations that remain relatively accessible (e.g., bounding boxes and labels of a detection, labels of a legend) to segment a given image. ComViSe embeds a segmentation framework, pre-trained on a semantically different dataset, to generate image region proposals. The pipeline relies then on several semantic, visual and geometric criteria to characterize each proposed region, and combines them to select the optimal segmentation mask, comparing diverse aggregation strategies from handcrafted formula to automatic ones, supervised or not. An experimental study conducted on the PASCAL VOC dataset shows that these effectively combined criteria are enough to select the mask proposals with the best IoU score in most cases, and that the aggregation can be done automatically.
更多查看译文
关键词
segmentation with test clues, region proposal, fusion of outputs, combination of criteria
AI 理解论文
溯源树
样例
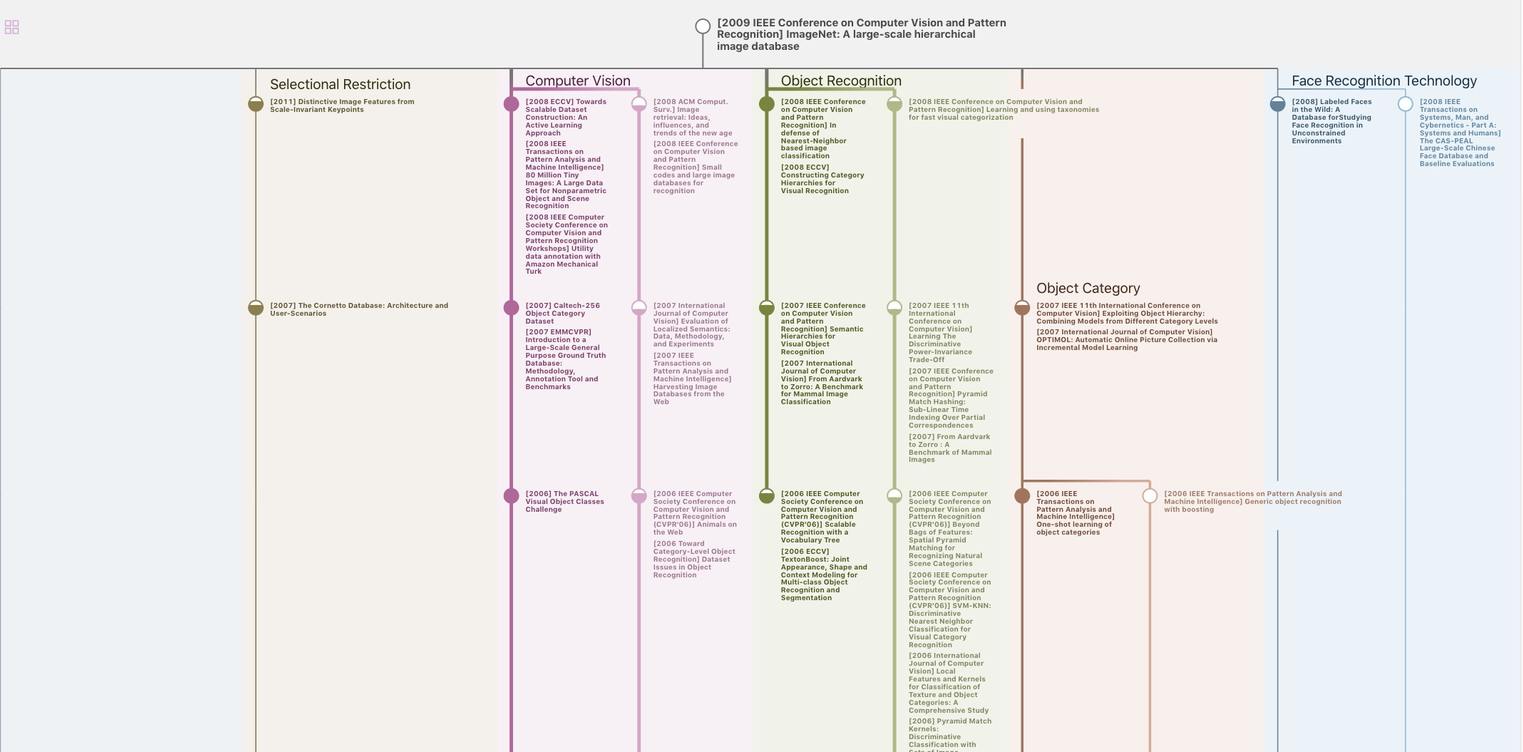
生成溯源树,研究论文发展脉络
Chat Paper
正在生成论文摘要