Global Translation of Classification Models
INFORMATION(2022)
摘要
The widespread and growing usage of machine learning models, particularly for critical areas such as law, predicate the need for global interpretability. Models that cannot be audited are vulnerable to biases inherited from the datasets that were used to develop them. Moreover, locally interpretable models are vulnerable to adversarial attacks. To address this issue, the present paper proposes a new methodology that can translate any existing machine learning model into a globally interpretable one. MTRE-PAN is a hybrid SVM-decision tree architecture that leverages the interpretability of linear hyperplanes by creating a set of polygons that delimit the decision boundaries of the target model. Moreover, the present paper introduces two new metrics: certain and boundary model parities. These metrics can be used to accurately evaluate the performance of the interpretable model near the decision boundaries. These metrics are used to compare MTRE-PAN to a previously proposed interpretable architecture called TRE-PAN. As in the case of TRE-PAN, MTRE-PAN aims at providing global interpretability. The comparisons are performed over target models developed using three benchmark datasets: Abalone, Census and Diabetes data. The results show that MTRE-PAN generates interpretable models that have a lower number of leaves and a higher agreement with the target models, especially around the most important regions in the feature space, namely the decision boundaries.
更多查看译文
关键词
explainability, global interpretation, translation, machine learning, TRE-PAN, explainable AI
AI 理解论文
溯源树
样例
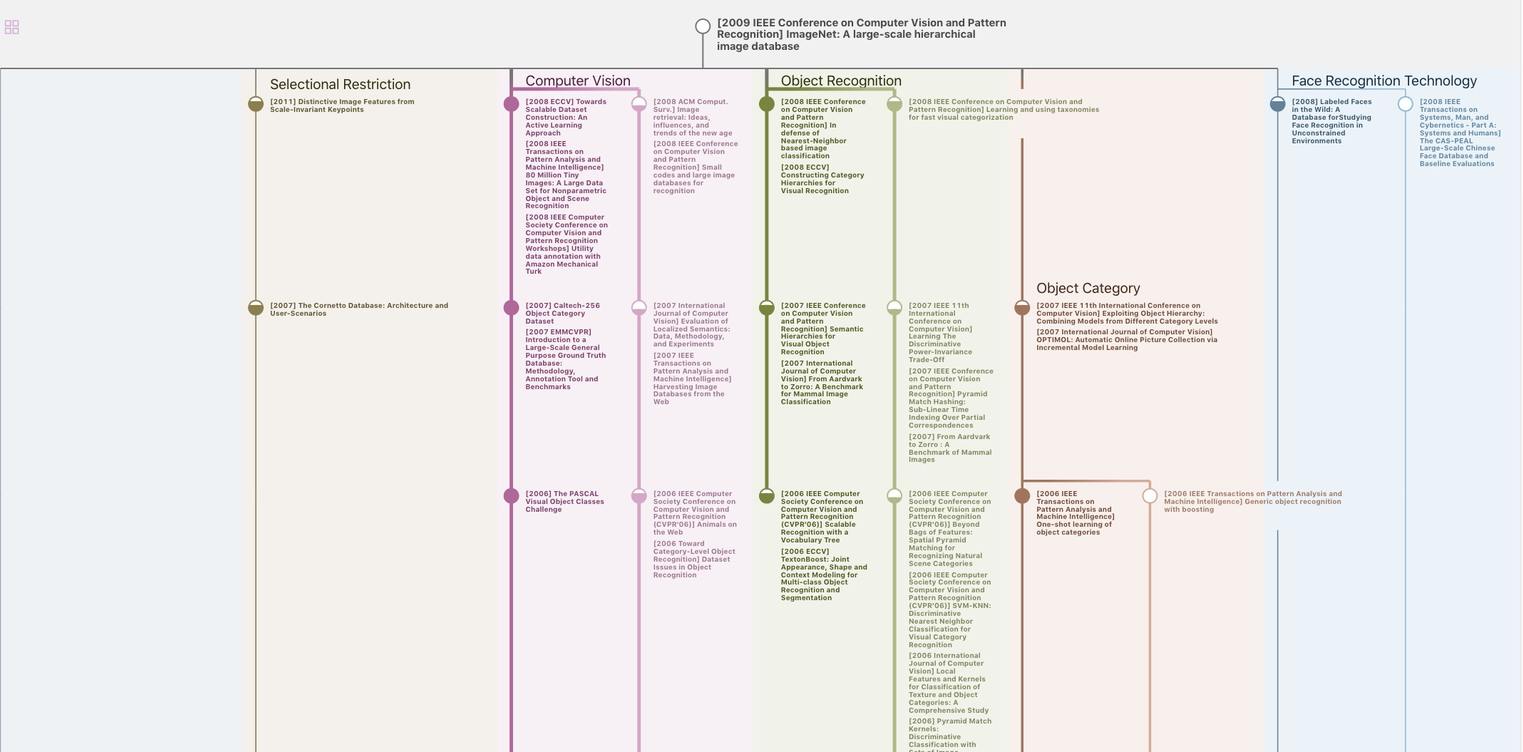
生成溯源树,研究论文发展脉络
Chat Paper
正在生成论文摘要