Comparison of Nitrogen Dioxide Predictions During a Pandemic and Non-pandemic Scenario in the City of Madrid using a Convolutional LSTM Network
INTERNATIONAL JOURNAL OF COMPUTATIONAL INTELLIGENCE AND APPLICATIONS(2022)
摘要
Traditionally, machine learning technologies with the methods and capabilities available, combined with a geospatial dimension, can perform predictive analyzes of air quality with greater accuracy. However, air pollution is influenced by many external factors, one of which has recently been caused by the restrictions applied to curb the relentless advance of COVID-19. These sudden changes in air quality levels can negatively influence current forecasting models. This work compares air pollution forecasts during a pandemic and non-pandemic period under the same conditions. The ConvLSTM algorithm was applied to predict the concentration of nitrogen dioxide using data from the air quality and meteorological stations in Madrid. The proposed model was applied for two scenarios: pandemic (January-June 2020) and non-pandemic (January-June 2019), each with sub-scenarios based on time granularity (1-h, 12-h, 24-h and 48-h) and combination of features. The Root Mean Square Error was taken as the estimation metric, and the results showed that the proposed method outperformed a reference model, and the feature selection technique significantly improved the overall accuracy.
更多查看译文
关键词
Air quality prediction, machine learning, ConvLSTM, COVID-19
AI 理解论文
溯源树
样例
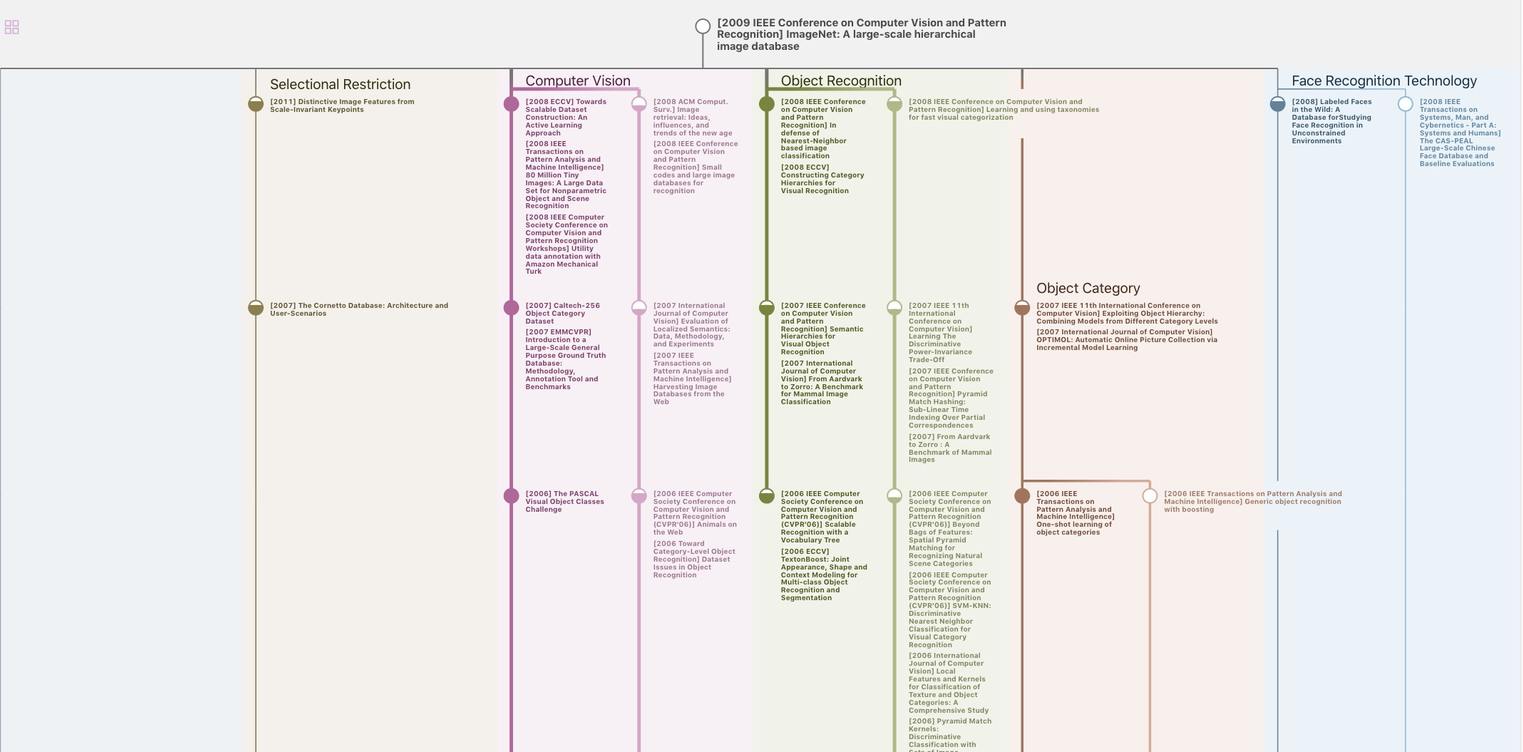
生成溯源树,研究论文发展脉络
Chat Paper
正在生成论文摘要