Comprehensively Evaluating the Performance of Species Distribution Models Across Clades and Resolutions: Choosing the Right Tool for the Job
LANDSCAPE ECOLOGY(2022)
摘要
Context Species distribution modeling (SDM) is an integral tool for conservation, biogeography, and climate change biology. However, practioners have to choose from increasingly numerous SDM algorithms performing well under different conditions, including Glade and resolution. Objectives To identify the most suitable SDM algorithms for trees, birds, mammals, and insects, uncover the driving factors of predictive performance, and examine how resolution affects performance and variable importance. Methods We use 27 SDM implementations, including random forests (RF), boosted regression trees (BRT), and Mahalanobis distance (MAH), and a comprehensive dataset of 49 species in Europe (trees, birds, mammals, and insects) to fit a total of 19,845 models, at 20 km, 10 km, and 5 km resolution. For selected species, we also compare the mapped predictions of 3 algorithms, and assess how variable importance changes with resolution for BRT. Results RF and BRT outperformed in terms of model performance (AUC = 0.938) for all clades (but not species), whereas decision trees, MaxLike, and Lasso overall underperformed (AUC =0.848). The performance majorly depended on both Glade (F =101.4) and its interaction with resolution (F=133.2), and displayed a general decline with resolution, while variable importance exhibited complex shifts in response to resolution. Conclusions RF and BRT are highly recommended but may require bias correction methods, whereas decision trees appeared unfavorable-particularly at higher resolutions. Given the complicated picture at the species level, varying tendencies to overfit, and resolution effects on both model performance and variable importance, we urge to routinely explore a range of algorithms, parametrizations, and resolutions. [GRAPHICS] .
更多查看译文
关键词
Algorithm,Clades,Species distribution model,Model performance,Resolution
AI 理解论文
溯源树
样例
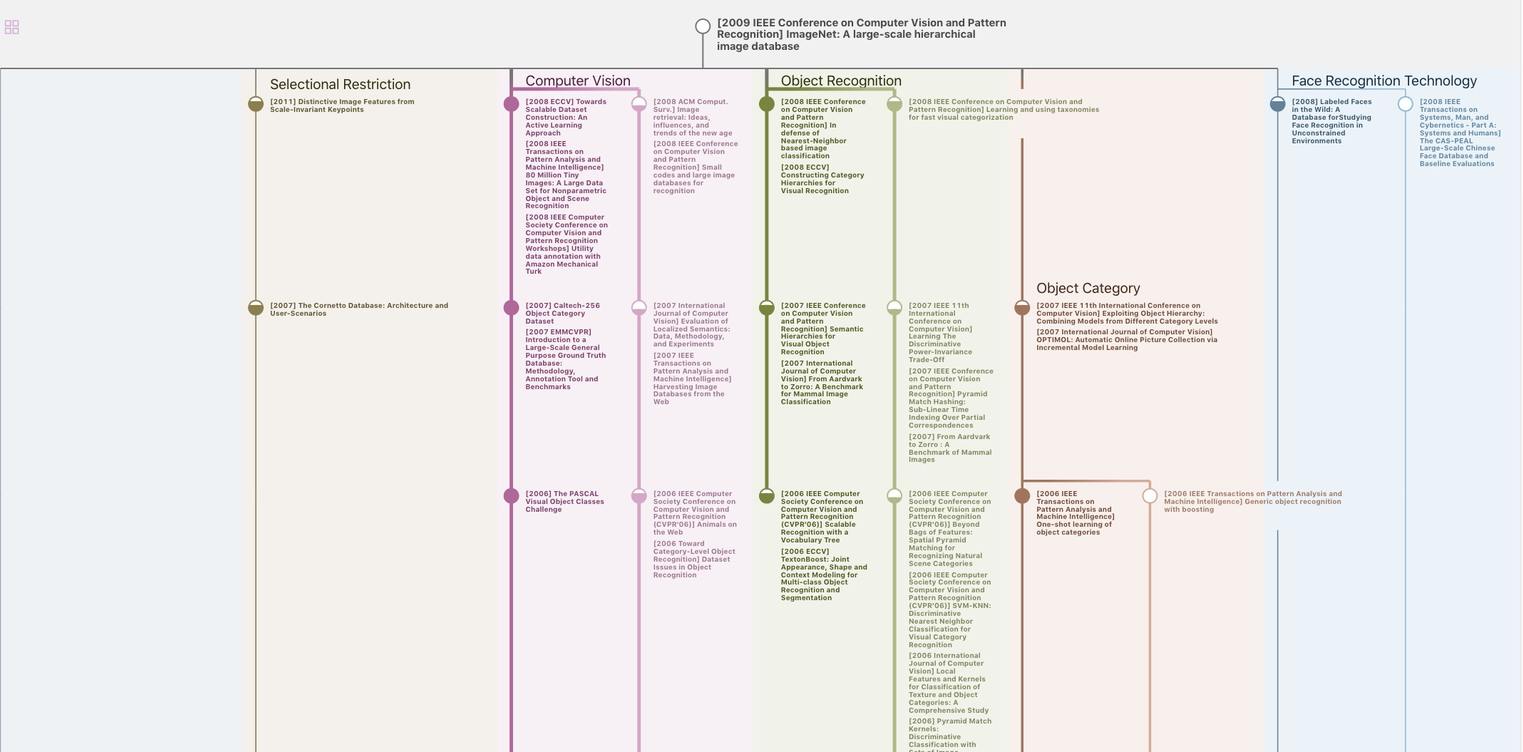
生成溯源树,研究论文发展脉络
Chat Paper
正在生成论文摘要