Invariant surface elastic properties in FCC metals and their correlation to bulk properties revealed by machine learning methods
Journal of the Mechanics and Physics of Solids(2022)
摘要
We present a combination of machine-learned models that predicts the surface elastic properties of general free surfaces in face-centered cubic (FCC) metals. These models are built by combining a semi-analytical method based on atomistic simulations to calculate surface properties with the artificial neural network (ANN) method or the boosted regression tree (BRT) method. The latter is also used to link bulk properties and surface orientation to surface properties. The surface elastic properties are represented by their invariants considering plane elasticity within a polar method. The resulting models are shown to accurately predict the surface elastic properties of seven pure FCC metals (Cu, Ni, Ag, Au, Al, Pd, Pt). The BRT model reveals the correlations between bulk and corresponding surface properties in terms of invariants, which can be used to guide the design of complex nano-sized particles, wires and films. Finally, by expressing the surface excess energy density as a function of surface elastic invariants, fast predictions of surface energy as a function of in-plane deformations can be made from these model constructs.
更多查看译文
关键词
Surface elastic properties,Surface elastic invariant,Molecular statics,Machine learning methods
AI 理解论文
溯源树
样例
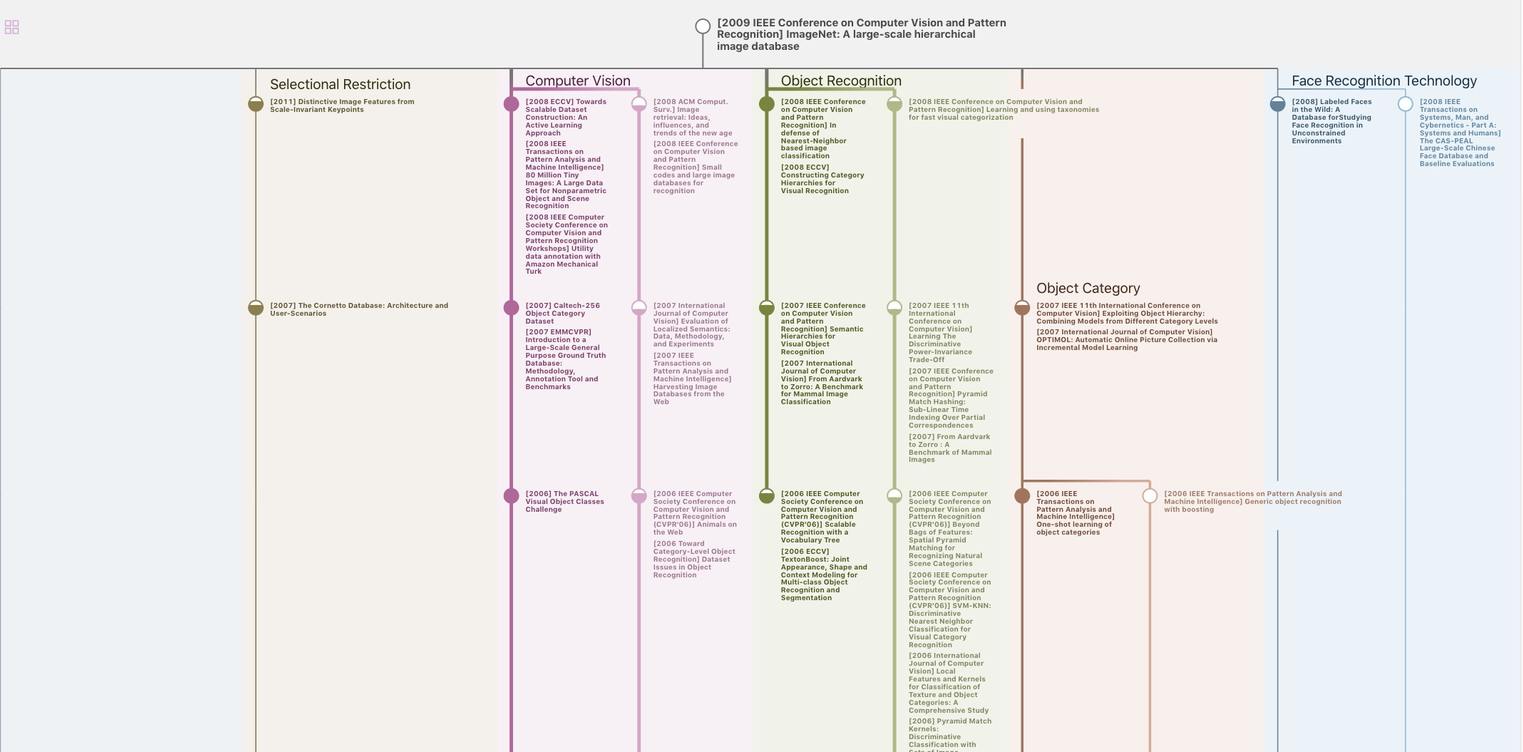
生成溯源树,研究论文发展脉络
Chat Paper
正在生成论文摘要