Energy-Aware Adaptive Multi-Exit Neural Network Inference Implementation for a Millimeter-Scale Sensing System
IEEE Transactions on Very Large Scale Integration (VLSI) Systems(2022)
摘要
Implementing a neural network (NN) inference in a millimeter-scale system is challenging due to limited energy and storage size. This article proposes an energy-aware adaptive NN inference implementation that utilizes one of two exits with different accuracies and computation options. The early-exit path provides a shorter processing time but less accuracy than the main-exit path. To compensate for the reduced accuracy, it additionally applies the main-exit path if the entropy of the early-exit inference is higher than a predetermined value. The NN is implemented with a custom low-power 180-nm CMOS processor chip and a 90-nm embedded flash memory chip and tested by the CIFAR-10 dataset. The measurement results show that the implemented convolutional NN (CNN) reduces processing time and thus energy consumption by 43.9% compared with a main-exit-only method while sacrificing its accuracy from 69.9% to 66.2%. Also, we explore the required minimum battery capacity at each optimal configuration for accuracy and/or energy consumption to achieve energy-autonomous operation under measured exemplary light profiles. It requires a minimum battery capacity of 855 mJ, acceptable for the target miniature system with two millimeter-scale batteries (684 mJ each). Compared with the state-of-the-art CNN technique (BranchyNet) allowing early stopping, the proposed design improves the accuracy by 0.7% and 3.3% to maintain energy-autonomous operation with two and one millimeter-scale batteries, respectively. Compared with the state-of-the-art lightweight CNN technique (MobileNet), this work provides flexibility with a tradeoff between accuracy and processing time for different application requirements.
更多查看译文
关键词
Battery capacity,energy harvesting,miniature system,multiexit,neural network (NN)
AI 理解论文
溯源树
样例
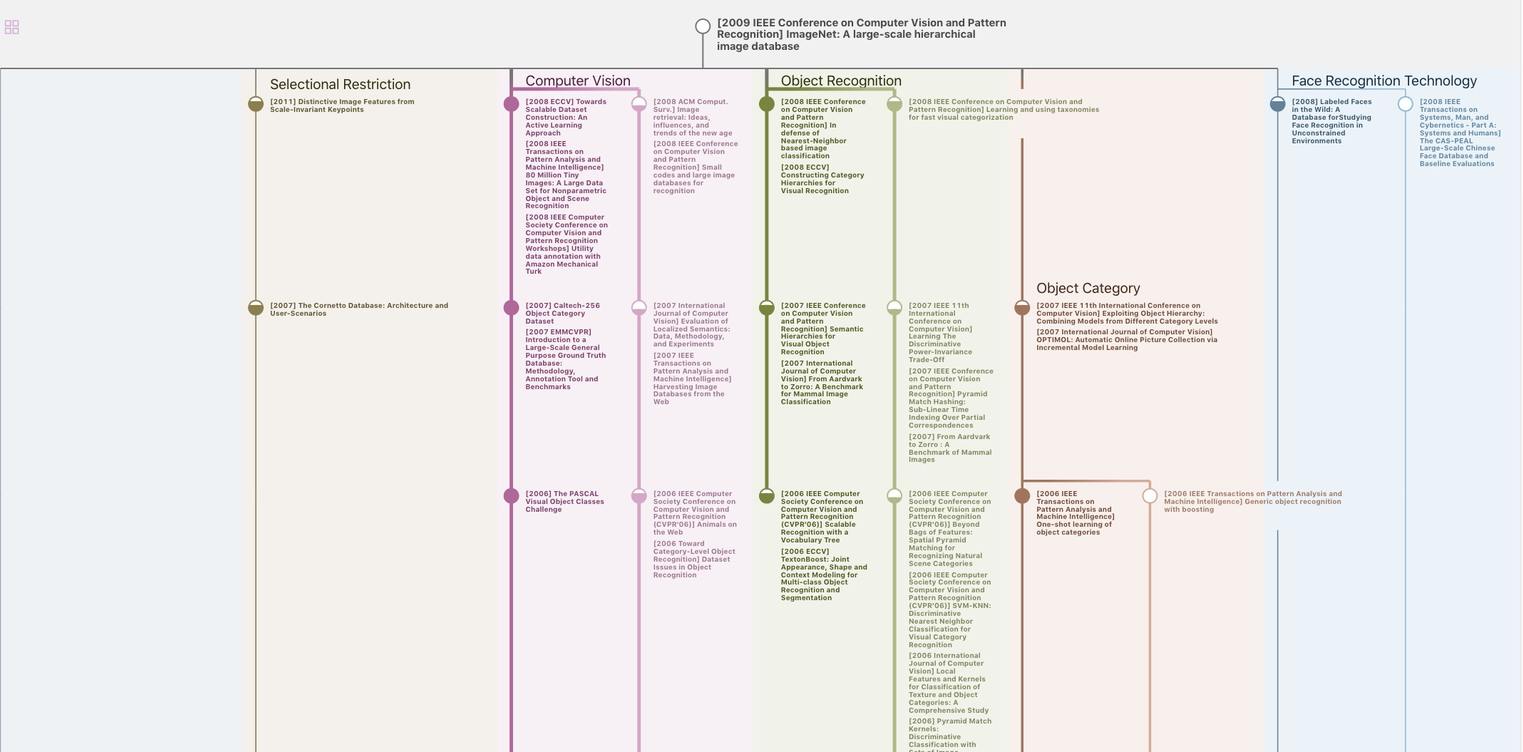
生成溯源树,研究论文发展脉络
Chat Paper
正在生成论文摘要