A Deep Learning-Based Approach for Statistical Robustness Evaluation in Proton Therapy Treatment Planning: a Feasibility Study.
Physics in Medicine & Biology(2023)
摘要
Objective. Robustness evaluation is critical in particle radiotherapy due to its susceptibility to uncertainties. However, the customary method for robustness evaluation only considers a few uncertainty scenarios, which are insufficient to provide a consistent statistical interpretation. We propose an artificial intelligence-based approach that overcomes this limitation by predicting a set of percentile dose values at every voxel and allows for the evaluation of planning objectives at specific confidence levels.Approach. We built and trained a deep learning (DL) model to predict the 5th and 95th percentile dose distributions, which corresponds to the lower and upper bounds of a two-tailed 90% confidence interval (CI), respectively. Predictions were made directly from the nominal dose distribution and planning computed tomography scan. The data used to train and test the model consisted of proton plans from 543 prostate cancer patients. The ground truth percentile values were estimated for each patient using 600 dose recalculations representing randomly sampled uncertainty scenarios. For comparison, we also tested whether a common worst-case scenario (WCS) robustness evaluation (voxel-wise minimum and maximum) corresponding to a 90% CI could reproduce the ground truth 5th and 95th percentile doses.Main results. The percentile dose distributions predicted by DL yielded excellent agreements with the ground truth dose distributions, with mean dose errors below 0.15 Gy and average gamma passing rates (GPR) at 1 mm/1% above 93.9, which were substantially better than the WCS dose distributions (mean dose error above 2.2 Gy and GPR at 1 mm/1% below 54). We observed similar outcomes in a dose-volume histogram error analysis, where the DL predictions generally yielded smaller mean errors and standard deviations than the WCS evaluation doses.Significance. The proposed method produces accurate and fast predictions (∼2.5 s for one percentile dose distribution) for a given confidence level. Thus, the method has the potential to improve robustness evaluation.
更多查看译文
关键词
deep learning,statistical robustness evaluation,proton radiotherapy,robustness evaluation,worst-case scenario,setup error,range error
AI 理解论文
溯源树
样例
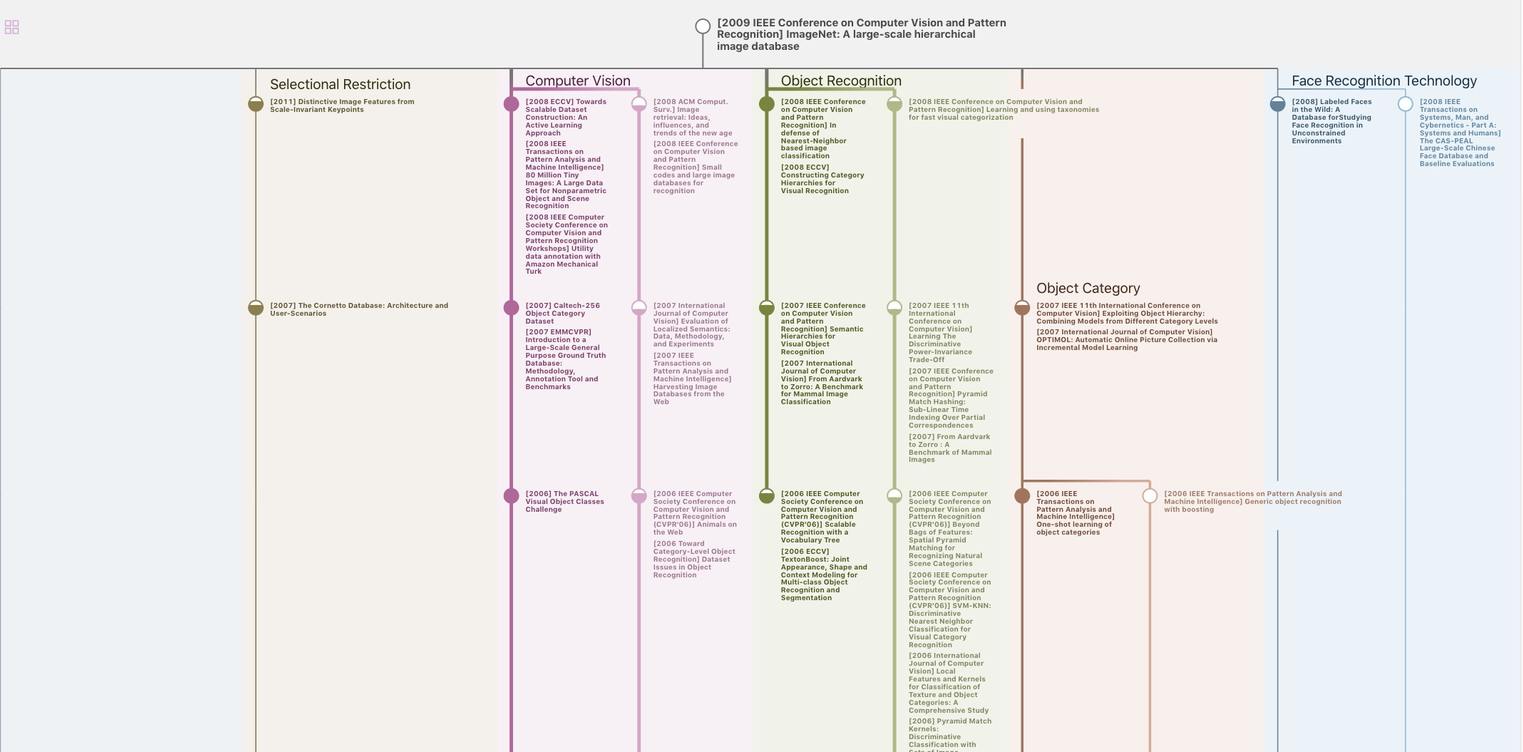
生成溯源树,研究论文发展脉络
Chat Paper
正在生成论文摘要