Levenshtein Augmentation Improves Performance of SMILES Based Deep-Learning Synthesis Prediction
semanticscholar(2020)
摘要
SMILESrandomization, a form of data augmentation, has previously been shown toincrease the performance of deep learning models compared to non-augmentedbaselines. Here, we propose a novel data augmentation method we call “Levenshteinaugmentation” which considers local SMILES sub-sequence similarity betweenreactants and their respective products when creating training pairs. The performanceof Levenshtein augmentation was tested using two state of the art models -transformer and sequence-to-sequence based recurrent neural networks withattention. Levenshtein augmentation demonstrated an increase performance over non-augmented, andconventionally SMILES randomization augmented data when used for training ofbaseline models. Furthermore, Levenshtein augmentation seemingly results inwhat we define as attentional gain – anenhancement in the pattern recognition capabilities of the underlying networkto molecular motifs.
更多查看译文
AI 理解论文
溯源树
样例
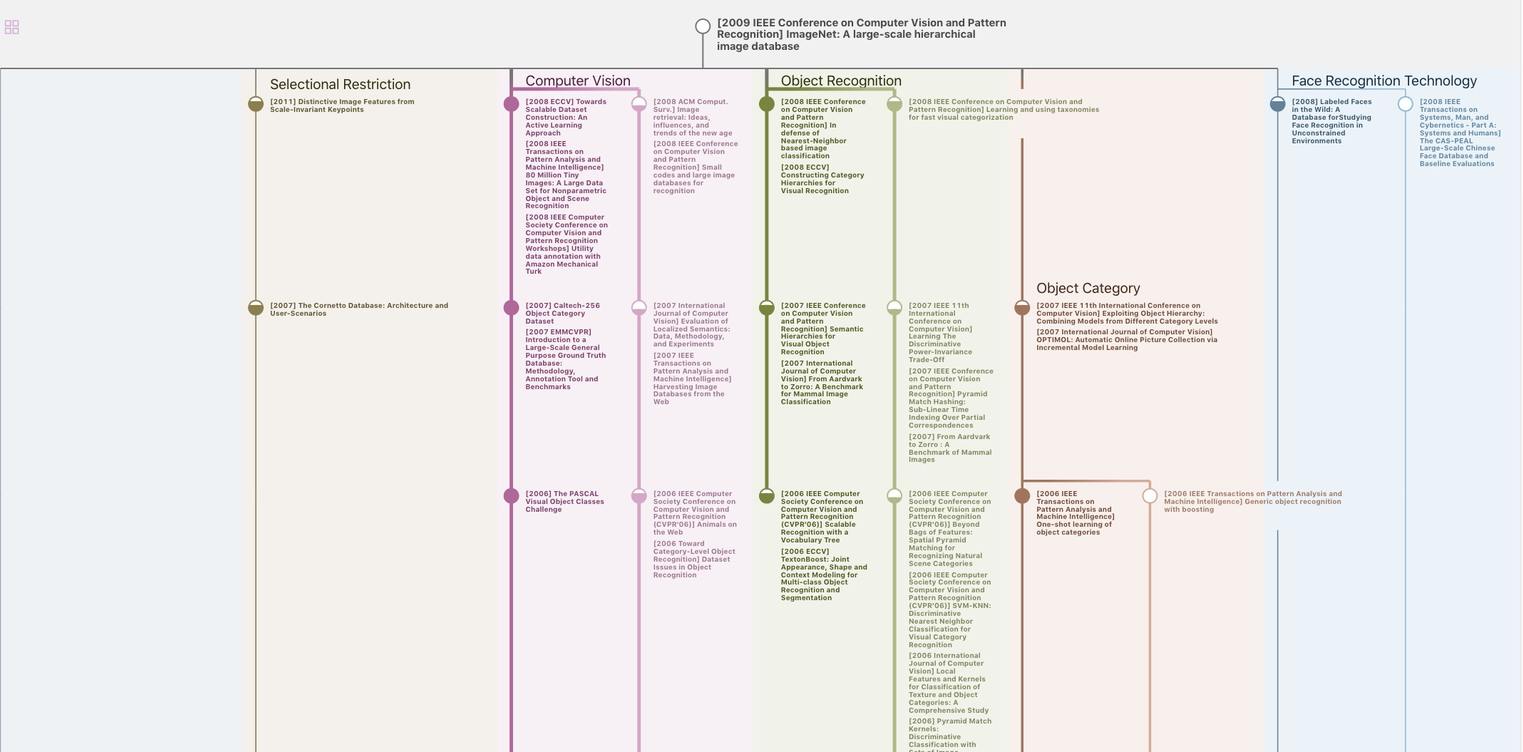
生成溯源树,研究论文发展脉络
Chat Paper
正在生成论文摘要