Science Autonomy and Space Science: Application to the ExoMars Mission
FRONTIERS IN ASTRONOMY AND SPACE SCIENCES(2022)
摘要
A machine learning approach for analyzing data from the Mars Organic Molecule Analyzer (MOMA) instrument has been developed in order to improve the accuracy and efficiency of this analysis and serves as a case study for the use of machine learning tools for space missions. MOMA is part of the science payload aboard the ExoMars rover, Rosalind Franklin, currently planned to land on Mars in 2023. Most NASA robotic space missions return only one thing: data. Remote planetary missions continue to produce more data as mission ambitions and instrument capabilities grow, yet the investigations are still limited by available bandwidth to transmit data back to Earth. To maximize the value of each bit, instruments need to be highly selective about which data are prioritized for return to Earth, as compression and transmission of the full data volume is often not feasible. The fundamental goal is to enable the concept of science autonomy, where instruments perform selected onboard science data analyses and then act upon those analyses through self-adjustment and tuning of instrument parameters. In this paper, we discuss the motivations, as well as related work on the use of machine learning for space missions. We also present a first step toward this vision of science autonomy for space science missions. This proof-of-concept exercise for the MOMA instrument aims to develop tools, used on Earth, to support Martian operations of the ExoMars mission. We also discuss the challenges and limitations of this implementation, as well as lessons learned and approaches that could be used for future space science missions.
更多查看译文
关键词
science autonomy, planetary science exploration, decision-making, machine learning, data science, exomars, mars organic molecule analyzer (MOMA), martian operations
AI 理解论文
溯源树
样例
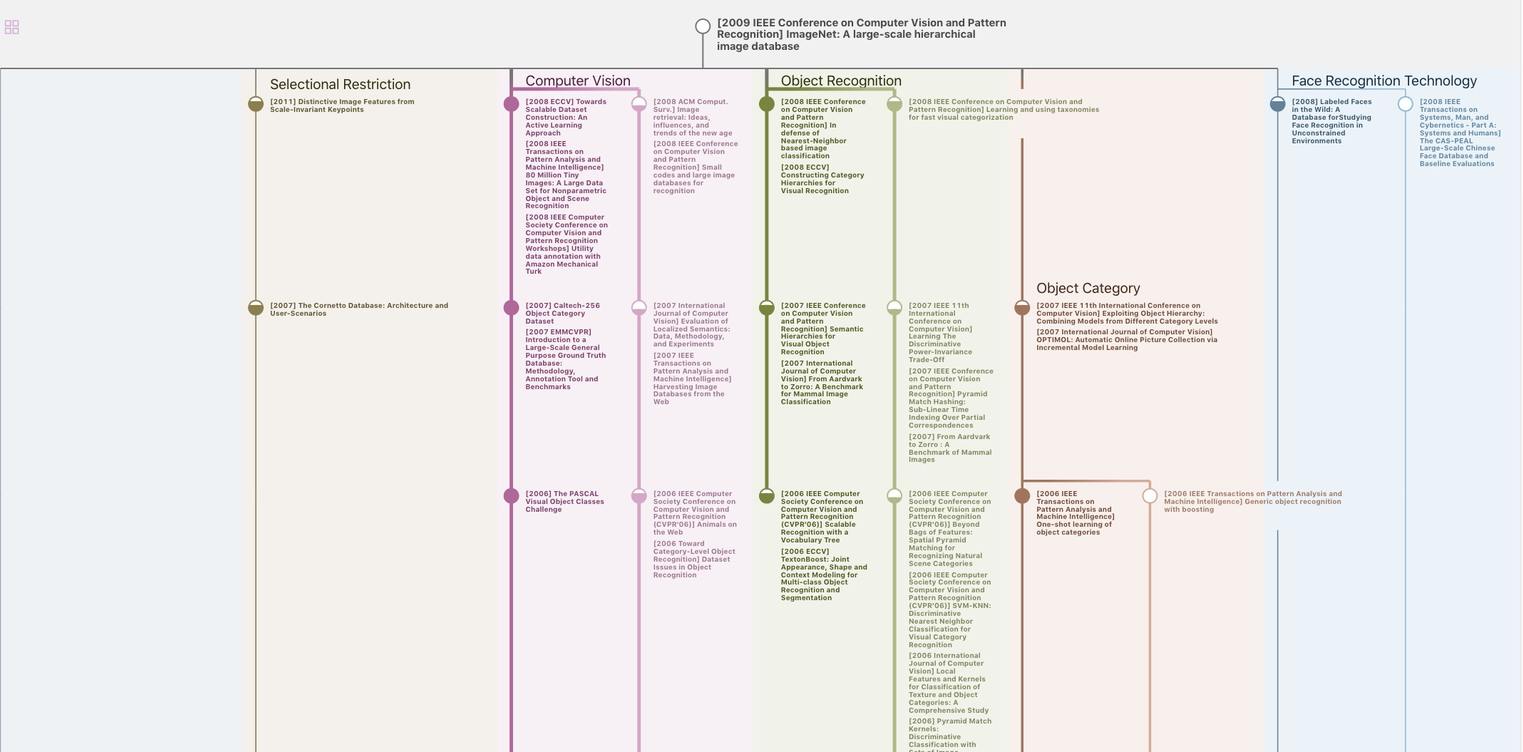
生成溯源树,研究论文发展脉络
Chat Paper
正在生成论文摘要