A Hybrid Model to Estimate Mean of Maximum Fields Inside Small Metal Enclosures Using Deep Neural Networks and Maximum Likelihood Estimator
IEEE Letters on Electromagnetic Compatibility Practice and Applications(2022)
摘要
This letter addresses a new regression model with the deep neural network (DNN) to estimate the mean of the maximum field inside two different nested-reverberation chamber configurations. In this model, a frequency range that the enclosure is expected to be in the over-moded regime is used as an input of the network, and the mean of the maximum field for both aperture scenarios is used as the output of the network. A 16-layer network with two regression heads provided the best regression model for both configurations, manifesting the trained model can accurately extrapolate the mean of maxima in the other frequency steps that are not used in the training data set. The tested and training root-mean-squared errors
$(485e^{-5}, 851e^{-5})$
are achieved with the network, demonstrating the network is feasible to detect the mean of maxima for two different nested-chamber configurations, extending the earlier work which considered estimation of the mean of maxima for a single nested chamber-configuration.
更多查看译文
关键词
Deep neural networks,dropout technique,mean of maximum fields,reverberation chambers
AI 理解论文
溯源树
样例
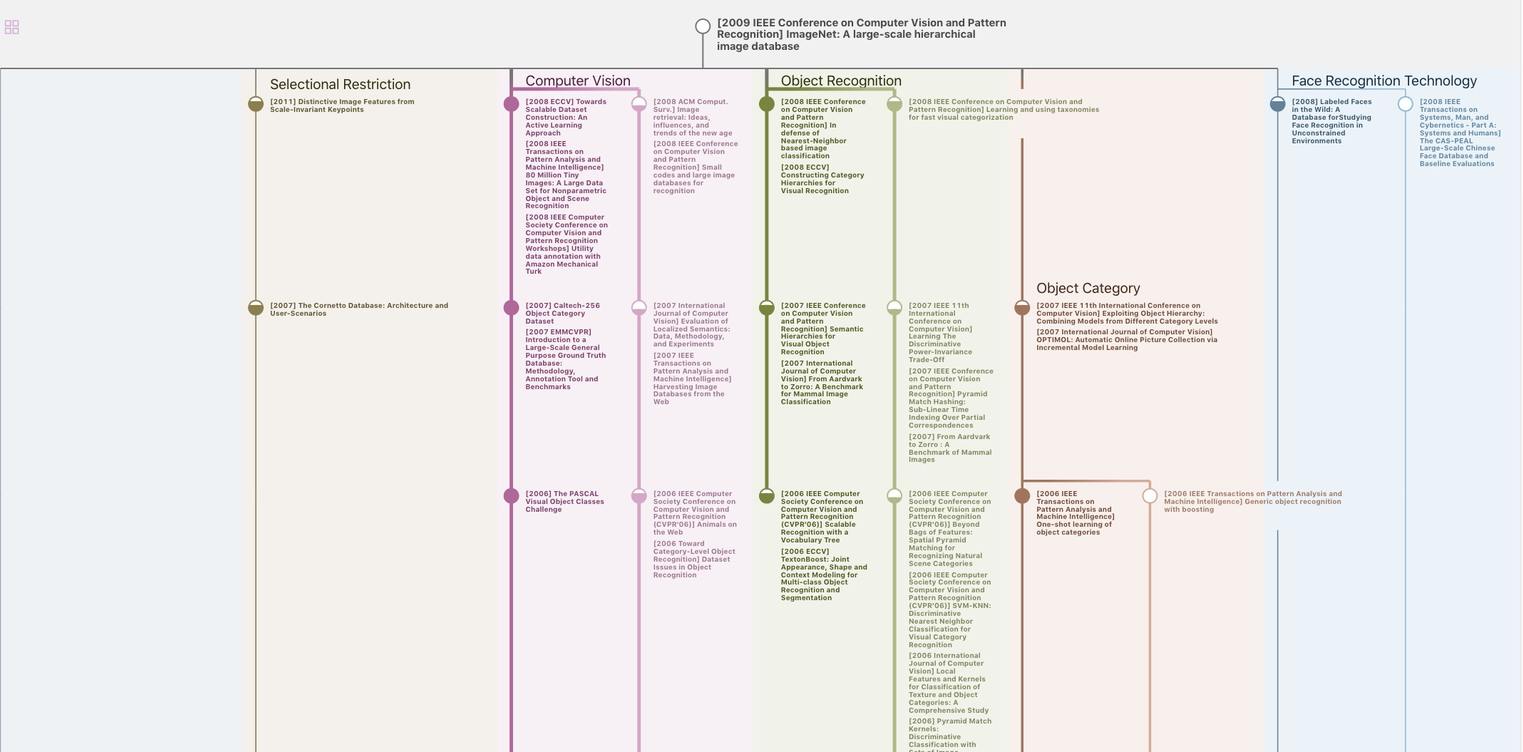
生成溯源树,研究论文发展脉络
Chat Paper
正在生成论文摘要