A Multiview Metric Learning Method for Few-Shot Fine-Grained Classification
IEEE ACCESS(2022)
摘要
Few-shot fine-grained image classification aims to solve the learning problem with few limited labeled examples. The existing methods use data augmentation to randomly transform the original examples to get new examples, and then use the new examples to train the model to improve the robustness and generalization ability of the learnt model. Due to each iteration of these methods uses a random transformation to get a new example, it will cause the unstable problem of the class center in the feature measurement stage. To solve this problem, a Multi-view Metric Learning (MML) method is proposed, which is based on a new concept (View Bag) and its effective similarity measurement method to achieve better few-shot fine-grained image classification. Firstly, a new example obtained by a kind of data augmentation is defined as a view, and a set of views generated by multiple data augmentation is defined as a view bag. Then, the view bag is sent into the model to extract the features, and a multi-view metric method with the view bag as the object is proposed to overcome the unstable problem of the class center. Finally, classification is performed by measuring the similarity between view bags. Experiments are conducted on three public datasets, CUB-2011-200, Stanford-Dogs and Stanford-Cars. The proposed method achieves 71.61 +/- 0.87%, 57.78 +/- 0.96% and 74.02 +/- 0.84% for the 5-way 1-shot classification task, and 88.72 +/- 0.51%, 76.30 +/- 0.68% and 92.94 +/- 0.37% for the 5-way 5-shot classification task, which have the state-of-the-art performances. Under the condition of the same backbone network, the proposed multi-view metric method can measure the similarity between examples more effectively, and improve the robustness and generalization ability of the model.
更多查看译文
关键词
Measurement, Training, Feature extraction, Task analysis, Robustness, Q measurement, Learning systems, Few-shot fine-grained image classification, fine-grained image classification, metric learning, date augmentation, multi-view metric
AI 理解论文
溯源树
样例
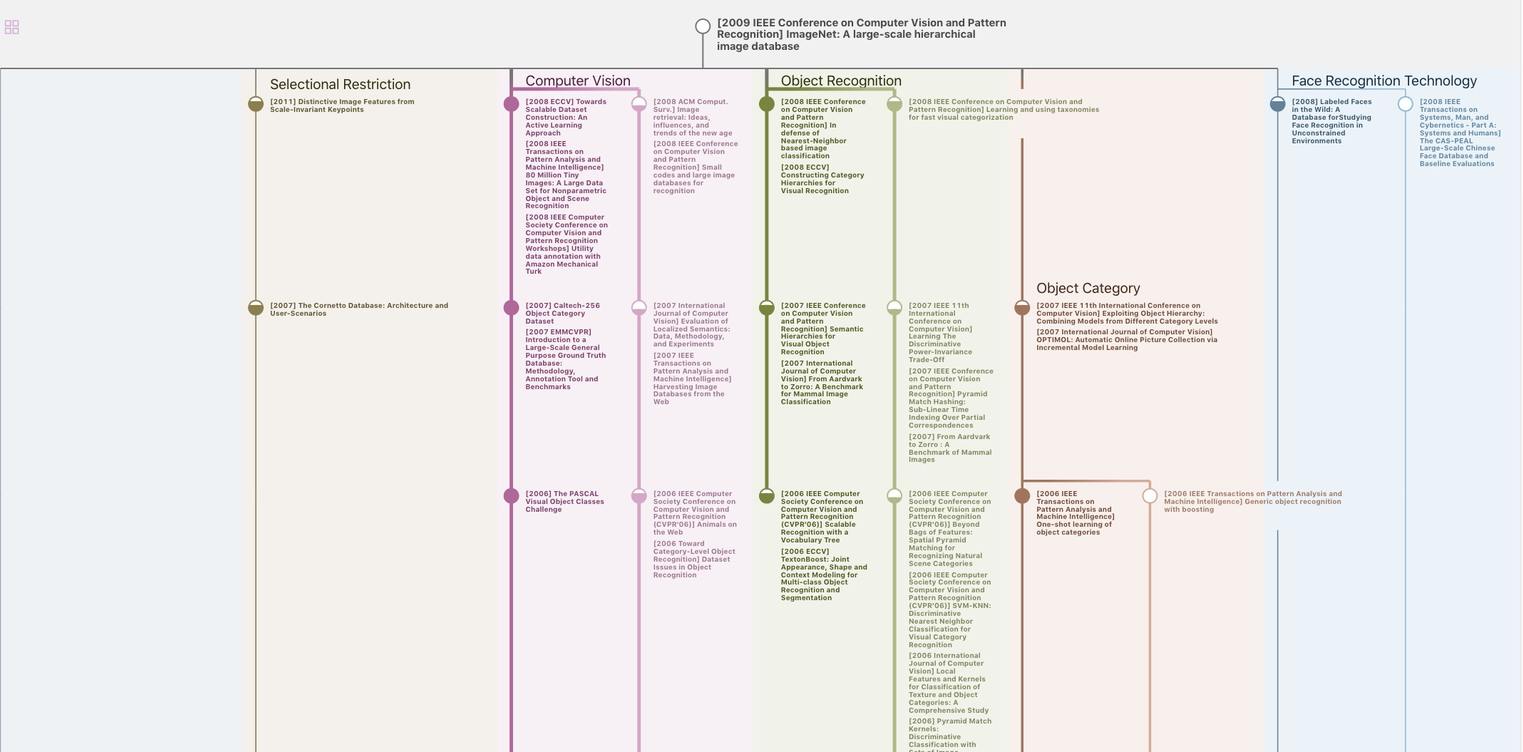
生成溯源树,研究论文发展脉络
Chat Paper
正在生成论文摘要