Learning-Based Joint QoE Optimization for Adaptive Video Streaming Based on Smart Edge
IEEE Transactions on Network and Service Management(2022)
摘要
The latest increase in HTTP-based adaptive video streaming over the Internet enables a growing number of clients to compete for a shared bottleneck bandwidth. This competition may affect users’ Quality of Experience (QoE) negatively, especially in terms of fairness and stability. This paper presents
Flex-Steward
, a solution that performs multi-client joint QoE optimization for adaptive video streaming during bottleneck bandwidth sharing. Joint QoE optimization refers to improving QoE fairness among clients with various video devices and availing from differentiated services with different priorities. Flex-Steward deploys an adaptive bitrate delivery algorithm based on Neural Networks (NN) and reinforcement learning at the network edge. It relies on a trained NN model to make appropriate bitrate recommendations in terms of video chunks to be requested by clients sharing the same bottleneck bandwidth. Flex-Steward is assessed in comparison with alternative state-of-the-art algorithms under different network conditions using a real-life prototype. Results show how Flex-Steward reduces the unfairness in terms of joint QoE optimization with between 10.9% and 41.7%.
更多查看译文
关键词
Bitrate recommendation,edge computing,reinforcement learning,joint QoE optimization
AI 理解论文
溯源树
样例
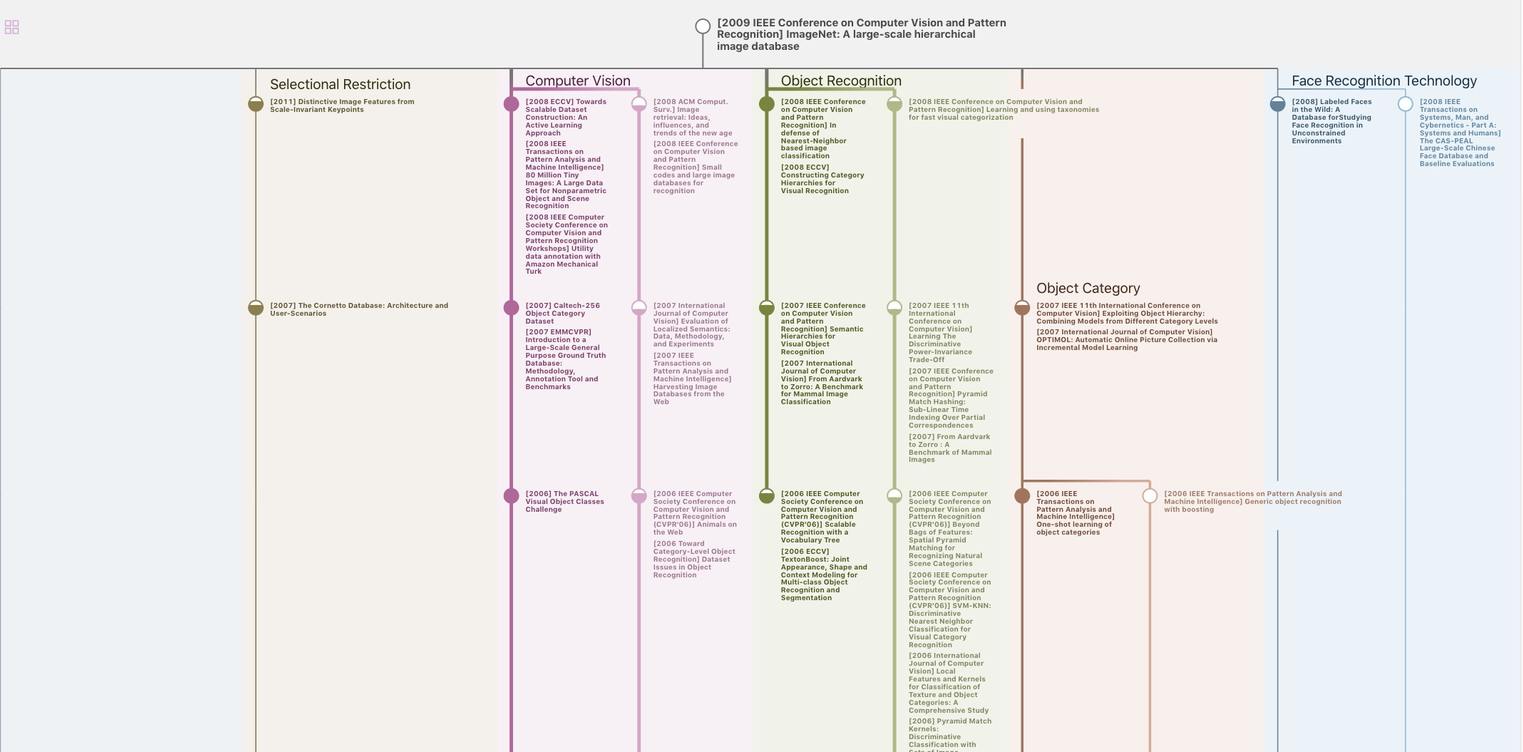
生成溯源树,研究论文发展脉络
Chat Paper
正在生成论文摘要