Lightweight Remote Sensing Road Detection Network
IEEE GEOSCIENCE AND REMOTE SENSING LETTERS(2022)
摘要
Aiming at the problems of large parameters and low detection speed of the existing remote sensing road detection network model, a lightweight remote sensing road detection network (LRSR-net) model is proposed in this letter. In the feature encoding stage of LRSR-net, an efficient road target feature extraction module dilated joint convolution module is proposed. This module can eliminate the feature loss caused by the pooling layer, so that the network model can use a small amount of parameters to learn the characteristics of road targets. In addition, in the feature decoding stage, to eliminate the damage of the target data structure caused by the upsampling operation, a joint decoding module is proposed. The joint decoding module can effectively map the characteristics of road targets and suppress the background clutter. The systematic experimental results have demonstrated that the proposed lightweight road target detection model has excellent detection performance under the condition of small model parameters, and most performance indexes are better than those of the existing advanced detection methods. The source code of this article can be obtained from https://github.com/yangzhen1252/IRSR-net.
更多查看译文
关键词
Feature extraction, Roads, Convolution, Decoding, Remote sensing, Kernel, Encoding, Dilated joint convolution module, joint decoding module, lightweight remote sensing road detection network (LRSR-net), remote sensing road detection
AI 理解论文
溯源树
样例
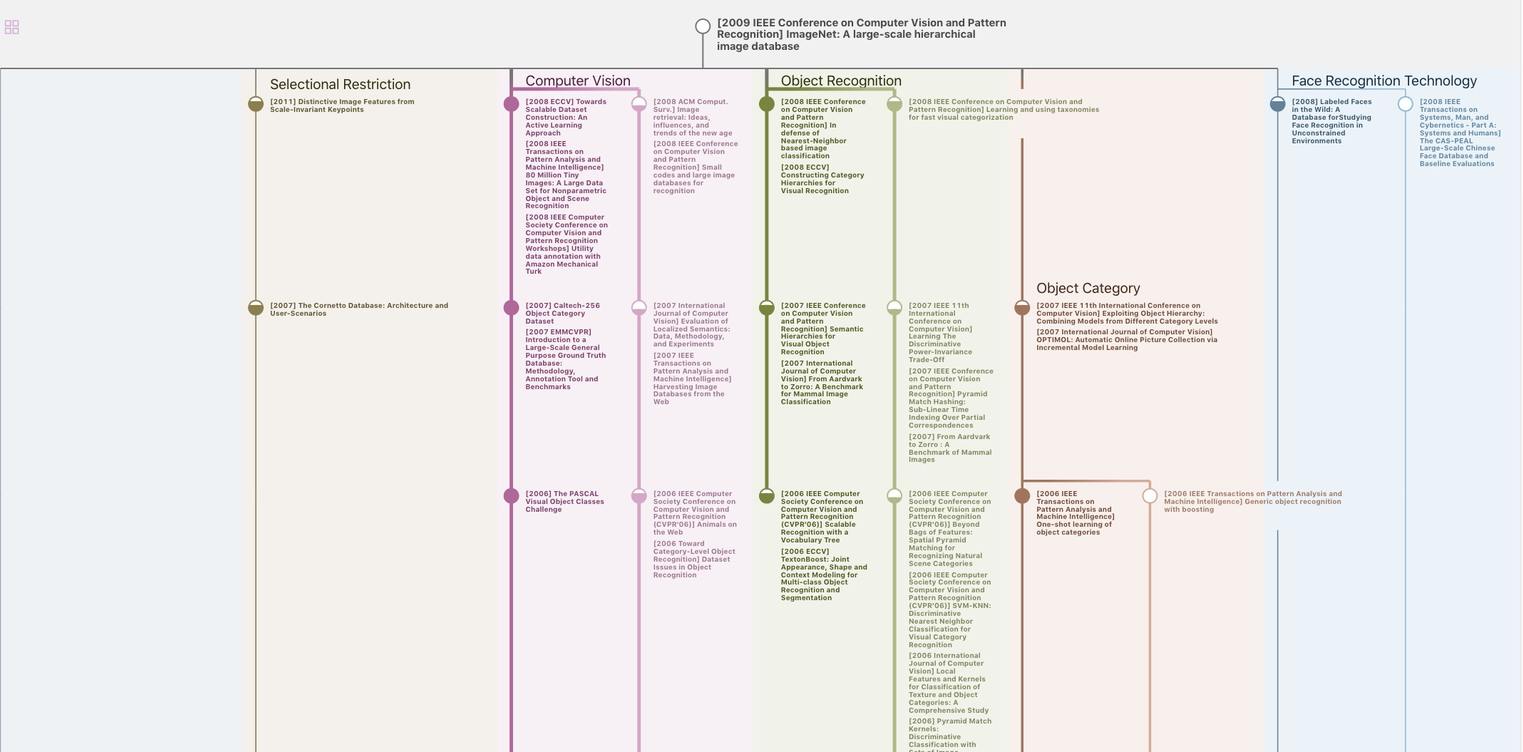
生成溯源树,研究论文发展脉络
Chat Paper
正在生成论文摘要