An Injury-Severity-Prediction-Driven Accident Prevention System
SUSTAINABILITY(2022)
摘要
Traffic accidents are inevitable events that occur unexpectedly and unintentionally. Therefore, analyzing traffic data is essential to prevent fatal accidents. Traffic data analysis provided insights into significant factors and driver behavioral patterns causing accidents. Combining these patterns and the prediction model into an accident prevention system can assist in reducing and preventing traffic accidents. This study applied various machine learning models, including neural network, ordinal regression, decision tree, support vector machines, and logistic regression to have a robust prediction model in injury severity. The trained model provides timely and accurate predictions on accident occurrence and injury severity using real-world traffic accident datasets. We proposed an informative negative data generator using feature weights derived from multinomial logit regression to balance the non-fatal accident data. Our aim is to resolve the bias that happens in the favor of the majority class as well as performance improvement. We evaluated the overall and class-level performance of the machine learning models based on accuracy and mean squared error scores. Three hidden layered neural networks outperformed the other models with 0.254 +/- 0.038 and 0.173 +/- 0.016 MSE scores for two different datasets. A neural network, which provides more accurate and reliable results, should be integrated into the accident prevention system.
更多查看译文
关键词
ordinal regression, neural network, transportation safety, injury severity prediction, sustainable transportation
AI 理解论文
溯源树
样例
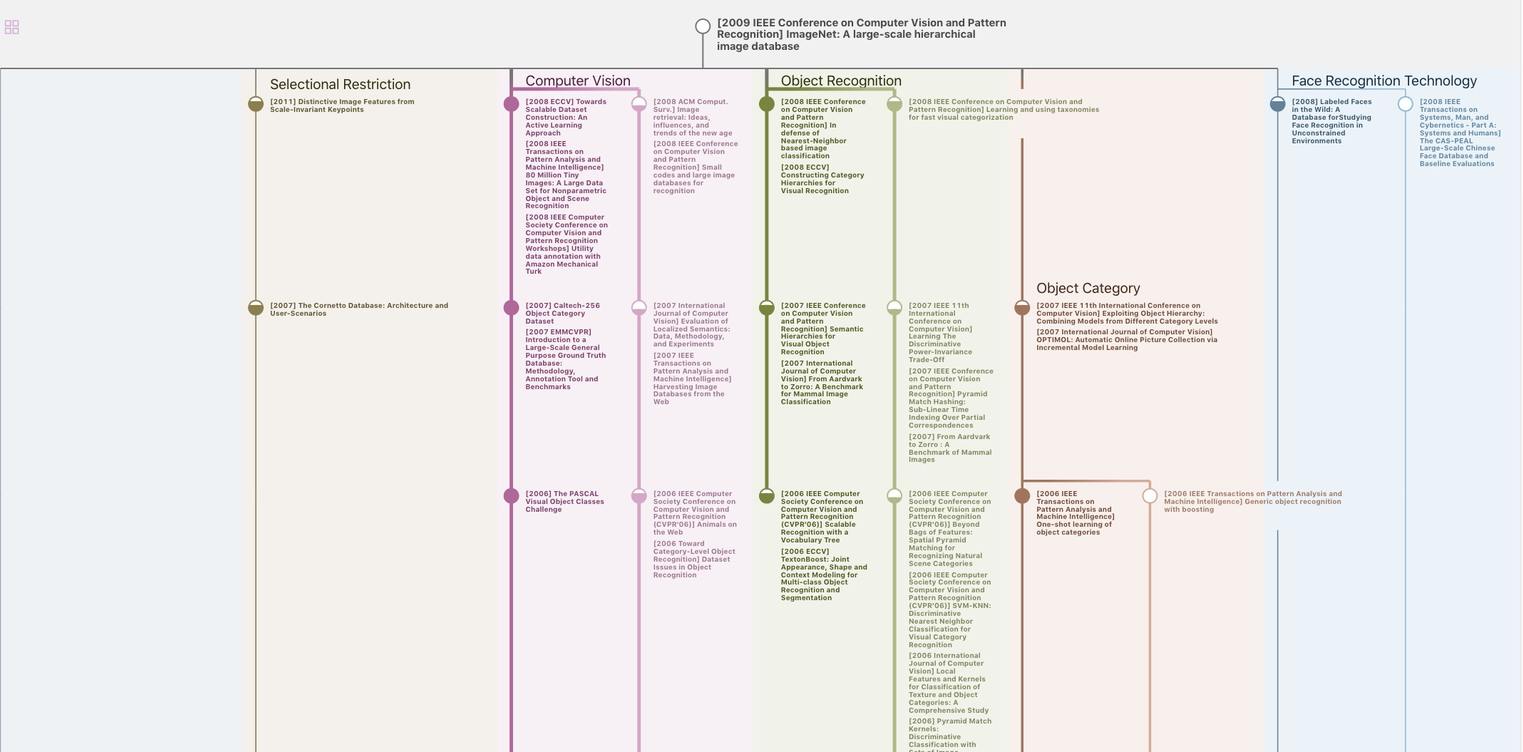
生成溯源树,研究论文发展脉络
Chat Paper
正在生成论文摘要