Brake wear induced PM10 emissions during the world harmonised light-duty vehicle test procedure-brake cycle
Journal of Cleaner Production(2022)
摘要
In this work, the particulate matter less than 10 μm (PM10) emissions from a medium-sized passenger vehicle's front brake wear were studied using a finite element analysis (FEA) and experimental approaches. The world harmonised light-duty vehicle test procedure-brake (WLTP-B) cycle was chosen to simulate real-world driving. An electrical low-pressure impactor (ELPI+) was used to count the brake wear particles on a brake dynamometer sealed in a chamber. In addition, a machine learning method, namely, extreme gradient boosting (XGBoost), was employed to capture the feature importance rankings of braking conditions contributing to brake wear PM10 emissions. The simulated PM10 emissions were quite consistent with the measured ones, with an overall relative error of 9%, indicating that the proposed simulation approach is promising to predict brake wear PM10 during the WLTP-B cycle. The simulated and experimental PM10 emission factors during the WLTP-B cycle were 6.4 mg km−1 veh−1 and 7.0 mg km−1 veh−1, respectively. Among the 10 trips of the WLTP-B cycle, the measured PM10 of trip #10 was the largest contributor, accounting for 49% of total PM10 emissions. On the other hand, the XGBoost results revealed that the top five most important factors governing brake wear PM10 emissions were dissipation energy, initial braking speed, final rotor temperature, braking power, and deceleration rate. From the perspective of friendly driving behaviour and regulation, limiting severe braking and high-speed braking has the potential to reduce PM10 emissions from brake wear.
更多查看译文
关键词
Non-exhaust emissions,Brake wear PM10 emissions,WLTP-B cycle,FEA,Machine learning
AI 理解论文
溯源树
样例
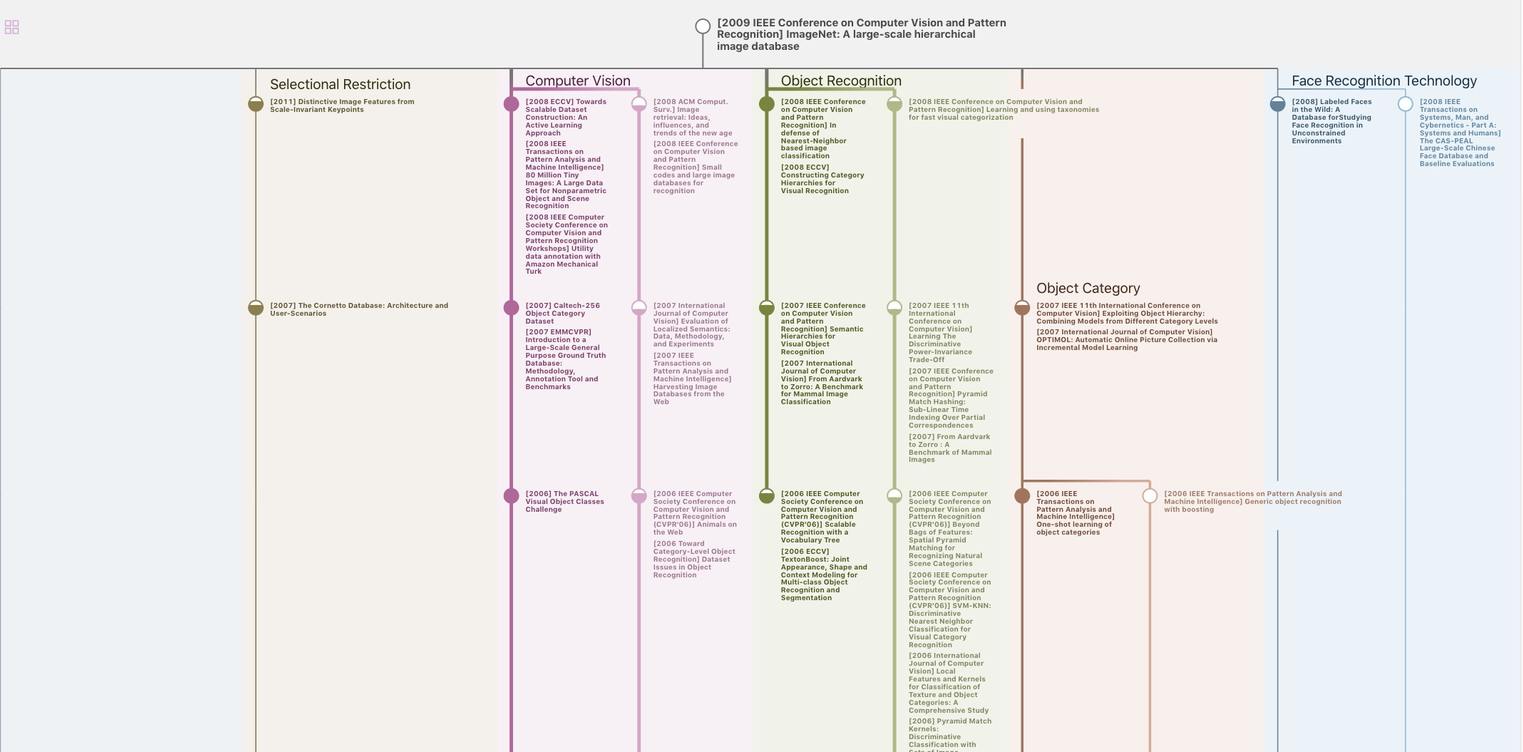
生成溯源树,研究论文发展脉络
Chat Paper
正在生成论文摘要